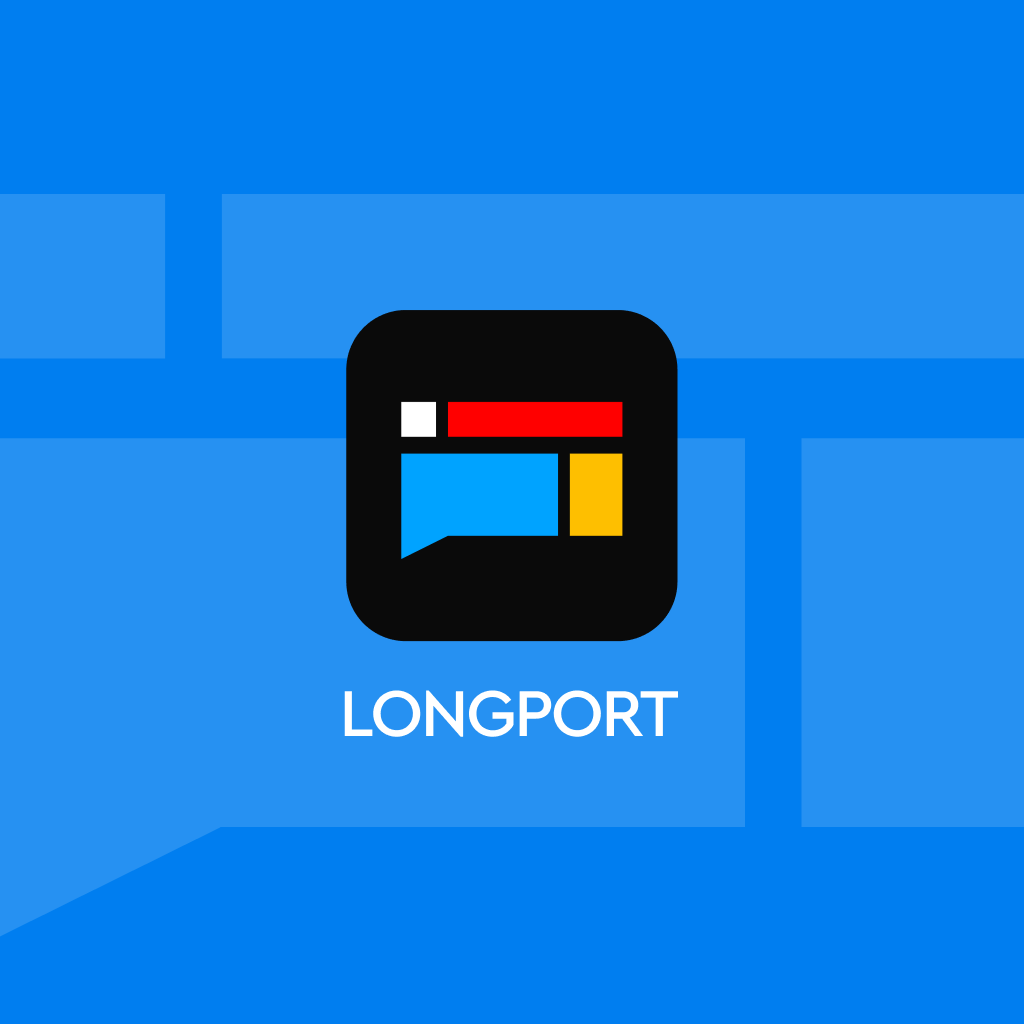
Nvidia (Minutes): The shipment ratio to China remains unchanged
NVIDIA (NVDA.O) released its Q4 fiscal year 2025 financial report (ending January 2025) after U.S. stock market hours on February 27, Beijing time. The details of the conference call are as follows:
Below is the minutes of NVIDIA's Q4 fiscal year 2025 earnings call. For financial interpretation, please refer to NVIDIA: Deepseek, has it pierced through Jensen Huang's "leather jacket"?
1. $NVIDIA(NVDA.US) Core Financial Information Review
- Core financial information: Q4 of fiscal year 2025 was a record quarter, with revenue reaching $39.3 billion, a 12% increase quarter-over-quarter and a 78% increase year-over-year, exceeding the expected $37.5 billion. Total revenue for fiscal year 2025 was $130.5 billion, an increase of 114% from the previous year.
- Data Center Division:
Q4 revenue reached a record $35.6 billion, a 16% increase quarter-over-quarter and a 93% increase year-over-year; FY2025 revenue was $115.2 billion, more than doubling year-over-year;
Blackwell sales exceeded expectations in Q4, reaching $11 billion, marking the fastest product ramp in the company's history, with full-scale production and rapid supply increase. Hopper 200 continues to grow. Q4 data center computing revenue increased 18% quarter-over-quarter and more than doubled year-over-year; Q4 computing revenue increased 18% quarter-over-quarter and more than doubled year-over-year;
Inference demand: Customers are competing to expand infrastructure for training next-generation cutting-edge models and unleashing AI capabilities, with large clusters often starting with 100,000 or more GPUs. There is strong demand for post-training and model customization, with inference demand accelerating, as the computational requirements for long-thought inference AI tasks are 100 times greater than single inference tasks.
CSPs contribution: Large CSPs accounted for about half of data center revenue in Q4, with sales nearly doubling year-over-year; the proportion of regional cloud hosting in data center revenue has increased, reflecting the construction of global AI factories and the growing demand for AI inference models and agents;
Consumer Internet: Driven by generative AI and deep learning use cases (such as recommendation systems, vision, language understanding, synthetic data generation, search, and agent-based AI), consumer internet revenue tripled year-over-year;
Enterprise revenue: Nearly doubled year-over-year due to the growing demand for model fine-tuning, RAG, agent AI workflows, and GPU-accelerated data processing;
- Gaming Division:
Q4 Revenue: $2.5 billion, a decrease of 22% quarter-over-quarter and 11% year-over-year. The decline in revenue is due to supply constraints, but demand remained strong throughout the holiday season. With an increase in supply, strong sequential growth is expected in the first quarter.
Annual Revenue: $11.4 billion, a year-over-year increase of 9%.
- Professional Visualization Business:
Q4 Revenue: $511 million, a quarter-over-quarter increase of 5% and a year-over-year increase of 10%.
Annual Revenue: $1.9 billion, a year-over-year increase of 21%. The main vertical industries driving demand include automotive and healthcare.
- Automotive Business Division:
Q4 Revenue: A record $570 million, a quarter-over-quarter increase of 27% and a year-over-year increase of 103%.
Annual Revenue: $1.7 billion, a year-over-year increase of 5%. The continued growth of autonomous vehicles, including automotive and robotic axles, drove strong revenue growth.
Business Progress: Continued growth in the field of autonomous vehicles, including automotive and robotic taxis. At CES, it was announced that Toyota will build the next generation of vehicles based on NVIDIA technology, and Aurora and Continental will deploy NVIDIA-powered autonomous trucks at scale. NVIDIA's end-to-end autonomous driving platform has passed industry safety assessments.
Networking Business Revenue: Q4 decreased by 3% quarter-over-quarter. However, the company is transitioning from small NVLink 8 and InfiniBand to larger and relinke72 and Spectrum X, and networking business is expected to recover growth in the first quarter.
Gross Margin:
Q4 GAAP Gross Margin: 73%.
Q4 non-GAAP Gross Margin: 73.5%. With the growth of Blackwell, gross margin is initially expected to be around 70%, and will return to around 70% later in the fiscal year.
- Operating Expenses:
Q4 GAAP Operating Expenses: Increased by 9% quarter-over-quarter.
Q4 non-GAAP Operating Expenses: Increased by 11% quarter-over-quarter, due to increased engineering development costs and computing infrastructure costs required for new product launches.
FY2026 Q1 Expected GAAP Operating Expenses: Approximately $5.2 billion.
FY2026 Q1 Expected non-GAAP Operating Expenses: Approximately $3.6 billion.
FY2026 Annual Operating Expenses: Expected to increase by around 30%.
- Shareholder Returns:
In Q4, $8.1 billion was returned to shareholders in the form of stock buybacks and cash dividends.
FY2026 Outlook: Total revenue is expected to be $43 billion, plus or minus 2%; GAAP gross margin is expected to be 70.6%, plus or minus 50 basis points; Non-GAAP gross margin is expected to be 71%, plus or minus 50 basis points; GAAP and Non-GAAP other income is expected to be approximately $100 million, excluding non-marketable and publicly held equity securities gains and losses; GAAP and Non-GAAP tax rates are expected to be 17%, plus or minus 1%, excluding any discrete items II. Detailed Content of NVIDIA's Earnings Call
2.1 Key Information from Executives
- Innovation and Market Leadership in Data Centers:
- Blackwell Architecture: This is the fastest product upgrade in the company's history, unprecedented in speed and scale. Production of Blackwell is in full swing across various configurations, and the range of customer adoption is rapidly expanding. It meets the demands of the entire artificial intelligence market, spanning from pre-training, post-training to inference, across various environments such as cloud, on-premises, and enterprise.
Inference Advantages: Compared to previous generations, Blackwell's AI inference models have higher token throughput and lower costs. Its revolutionary transformer engine is designed for LLM and mixed expert inference, and the envyLink Domain offers higher throughput than PCIe Gen 5, ensuring the necessary response times, throughput, and cost efficiency to handle the growing complexity of inference at scale.
- Ecosystem and Partnerships: The company's full-stack optimization and its vast ecosystem, which includes 5.9 million developers, continuously enhance customer economic benefits. Many leading companies and platforms across various industries are leveraging NVIDIA's infrastructure and software to develop a range of AI applications, such as Microsoft Bing utilizing NVIDIA TensorRT and acceleration libraries for significant speed and cost savings.
Expanding into Robotics and Automotive:
Robotics Applications: As AI development transcends the digital world, NVIDIA's infrastructure and software platform are increasingly being applied to the development of robotics and physical AI, particularly in the autonomous vehicle sector. Almost all AV companies are utilizing NVIDIA for development in data centers, automotive, or both.
Growth in the Automotive Vertical: The company's revenue in the automotive vertical is expected to grow significantly. At CES, Hyundai Motor Group announced the adoption of NVIDIA® technology to accelerate AV and robotics development as well as smart factory initiatives. Additionally, the NVIDIA Cosmos World Foundation model platform was launched, a physical AI platform that could revolutionize robotics technology, which has been adopted by ride-sharing giant Uber and others.
- Regional Market Dynamics:
U.S. Market: Driven by the preliminary rollout of Blackwell, the continuous growth of U.S. data center revenue is the strongest.
Global AI Ecosystem Development: Countries around the world are establishing their own AI ecosystems, leading to a surge in demand for computing infrastructure. For example, France's €100 billion AI investment and the EU's €200 billion AI plan indicate that global AI infrastructure is set to be redefined in the coming years.
Chinese Market: The proportion of China's data center sales to total data center revenue remains far below the levels at the onset of export controls. With no changes in regulations, it is expected that shipments to China will roughly maintain the current proportion, and the company will continue to comply with export controls while serving its customers
- Network Solutions and Future Growth:
Spectrum X and NVLink Switches: The company is transitioning from Spectrum X to NVLink 72, with increasing revenue from Spectrum X and NVLink switches becoming a new major growth point. The first batch of Stargate data centers will use Spectrum X, and Cisco has announced the integration of X into its network product portfolio to help enterprises build AI infrastructure, which will bring NVIDIA Ethernet into various industries thanks to Cisco's large enterprise footprint and global influence.
AI-Driven Network Demand: AI requires new types of networks, and NVIDIA provides a variety of network solutions, such as Link switch systems for scaling compute or horizontal scaling, quantum incentives for HPC supercomputers, and Spectrum acts for Ethernet environments.
- Gaming and Professional Visualization Innovations:
New GeForce 50 Series Graphics Processors: These GPUs are designed for gamers, creators, and developers, integrating AI and graphics technology to redefine visual computing. They are powered by the Blackwell architecture, achieving a 2x performance leap and featuring new AI-driven rendering capabilities such as neural shaders, digital human technology, geometry, and lighting. The new DLSS 4 can boost frame rates by up to 8 times through AI-driven frame generation.
Growth in Professional Visualization Business: NVIDIA technology and generative AI are reshaping design, engineering, and simulation workloads, increasingly applied in leading software platforms from companies like ANSYS, Cadence, and Siemens, driving demand for NVIDIA RTX workstations.
Upcoming Events and Conferences: Highlights upcoming events for the financial sector, including the TD Cowen Healthcare Conference in Boston, the Morgan Stanley Technology, Media, and Telecom Conference in San Francisco, and the GTC annual conference in San Jose, California, where Jensen Huang will deliver a keynote speech and provide a Q&A session for financial analysts.
2.2 Q&A
Q: The boundary between training and inference is becoming increasingly blurred. What does this mean for the future potential of inference-specific clusters, and what is the overall impact on NVIDIA and its customers?
A: There are now various scaling laws, including pre-training scaling laws, as multimodal and inference data are used for pre-training, which will continue to scale; post-training scaling, using reinforcement learning from human feedback, AI feedback, and verifiable rewards, where the computational load of post-training is higher than that of pre-training and can generate a large amount of synthetic data or synthetically generated tokens; test-time computation or inference, long thinking, etc., where the required inference computation is now 100 times more than the one-time examples and capabilities of early large language models, and the computational load of next-generation models may be thousands, tens of thousands, or even millions of times that of the current models. Designing such architectures is challenging because they need to run all models and perform well in training, and currently, most computation is inference, which is why NVIDIA's architecture is very popular Blackwell will incorporate reasoning models into its design philosophy, significantly enhancing training performance, with speeds tens of times faster for long reasoning, extended testing times, and a throughput increase of 25 times. NVIDIA's architecture is versatile and can configure data centers according to different needs, resulting in a higher concentration of unified architecture than ever before.
Q: What is the progress of GB 200's promotion at the system level? Are there still bottlenecks, and is there still enthusiasm for the NVL72 platform?
A: There is more enthusiasm than at CES, and more products have been delivered since CES. Manufacturing the Grace Blackwell rack involves 1.5 million components and is very complex, but production has successfully increased, achieving about $11 billion in revenue last quarter. Due to strong demand, customers are eager to receive products, and scaling up will continue. Many customers' Grace Blackwell systems are already online, and NVIDIA's own engineering, design, and software teams have also installed a large number. Overall, progress is good, and partners are performing well.
Q: Is the first quarter the bottom for gross margin? What factors give you confidence that strong demand will continue into next year? Has DeepSeek and its innovations changed this view?
A: During the promotion of Blackwell, the gross margin will be at a lower level of around 70%. The current focus is on accelerating manufacturing speed to deliver products to customers as soon as possible. Once Blackwell is fully promoted, it is expected to improve costs and gross margins, with gross margins expected to reach an intermediate level of over 70% later this year. The system is customizable, with various networking options and cooling methods, providing opportunities to enhance gross margins in the future, but the current focus is on completing manufacturing and delivering products as quickly as possible.
Q: How does NVIDIA assess market demand signals in the short, medium, and long term?
A: Short-term signals include purchase orders and forecasts; medium-term signals are the levels of infrastructure and capital expenditures (CapEx) compared to previous years; long-term signals are based on software transitioning from running on CPUs to running on GPUs and accelerated computing systems, which is the future trend of software. Currently, it only involves early areas of consumer artificial intelligence, search, some consumer generative AI, advertising, recommendation systems, etc. The next wave, such as enterprise agent AI, robotics with physical AI, and sovereign AI built for ecosystems in different regions, has just begun. DeepSeek and other innovations have not changed this view but rather reinforced our belief in the continuous development and opportunities in the AI field.
Q: Given that the current generation of Blackwell solutions is still ramping up, how should we understand the demand dynamics for the next generation Blackwell Ultra? How do customers and the supply chain manage the ramp-up of both products simultaneously? Will the team still be able to launch Blackwell Ultra as planned in the second half of this year? A: Blackwell Ultra will be launched in the second half of this year. The first generation of Blackwell products experienced issues that delayed it by two months, but it has now fully recovered and successfully increased production. Blackwell Ultra is advancing according to the annual plan, with new networks, memory, and processors, etc. Communication has been established with all partners and customers, who have the necessary information and will work together for a proper transition. The transition from Blackwell to Blackwell Ultra has the same system architecture, making it relatively easy, unlike the transition from Hopper to Blackwell, which involved significant changes in the system from NVLink 8 to NVLink 72, requiring alterations in chassis, system architecture, hardware, power transmission, etc., making it extremely challenging. Additionally, close collaboration with partners is underway to develop products following the release of Blackwell Ultra, which will be detailed at the GTC conference.
Q: How do you view the balance between customer-customized application-specific integrated circuits (ASICs) and commercial GPUs? Do customers plan to build heterogeneous superclusters that use both GPUs and ASICs simultaneously, or will these infrastructures remain relatively independent?
A: NVIDIA is fundamentally different from ASICs and has four advantages. First, the architecture is versatile, performing excellently across various models, including autoregressive models, diffusion-based models, vision-based models, and multimodal text models, with a rich software stack ecosystem that is the preferred target for many innovations and algorithms; Second, it has end-to-end capabilities, performing well in all stages from data processing, training data management, data training, reinforcement learning to inference, with a wide range of application scenarios suitable for cloud, on-premises deployment, and robotics; Third, it offers outstanding performance, with data center scale and power typically fixed, NVIDIA can achieve 2-8 times performance per watt, which can directly translate into revenue, as AI factories can monetize through generated tokens, making high token throughput extremely valuable for companies pursuing revenue and rapid return on investment; Fourth, the difficulty of building the software stack is high, with the architecture's ecosystem being ten times more complex than two years ago, integrating the entire ecosystem onto multi-chip is not easy, and NVIDIA not only has more advanced technology, better performance, and strong software capabilities but also deploys at an extremely fast pace.
Q: If regulations are implemented in other regions, can the U.S. market fill the gap? Is it feasible for the demand in the U.S. market to continue to surge? How can high-speed growth be maintained as business shifts to the U.S. market?
A: The market share in China remains roughly the same as in the fourth quarter and previous quarters, about 50% of the pre-export control level, with the proportion remaining stable. AI has become mainstream software, widely applied across various fields such as delivery services, shopping services, education, healthcare, financial services, climate technology, and mineral exploration. We are currently in the early stages of transitioning from traditional computing to AI computing; the data centers and computers built over the past few decades were based on coding, general computing, and CPUs, while in the future, almost all software and services will integrate AI, accelerating computing based on machine learning. No technology or software tool can touch a larger share of global GDP like AI does, and from this perspective, NVIDIA is still in the early stages of development, with significant growth potential Q: Did the enterprise business of data centers grow by 2 times year-on-year in the January quarter? If so, does this mean its growth rate is faster than that of hyperscale data centers? Hyperscale data centers purchase equipment for internal and external workloads; can you explain the spending allocation for external and internal workloads in hyperscale data centers? With the emergence of new AI workflow applications, will enterprises occupy a larger share in the consumption mix? Will this affect the development of services and ecosystems?
A: Yes, the growth of enterprise business is very similar to that of large cloud service providers (CSPs). Both collaboration with CSPs and the enterprises' own construction are developing well, and both areas are important.
CSPs account for about half of the business, with both internal and external consumption. Companies work closely with them to optimize their internal workloads, as they have a large infrastructure of NVIDIA equipment available, which is versatile in areas such as AI, video processing, and data processing, with a long lifespan and low total cost of ownership.
In the long run, the growth scale of non-CSP enterprises will be larger than that of CSPs. Taking automotive companies as an example, smart agent AI and software planning systems used by employees can improve work efficiency, and AI is also needed in automotive manufacturing. In the future, every car will be a robotic car that collects data and improves through AI factories. Additionally, physical systems require new types of physical AI. The use of smart agent AI to transform the way companies work internally has just begun; this is the start of the agent AI era, and in the long run, this part will be larger.
Q: As we approach the second anniversary of the Hopper architecture's turning point in the field of general artificial intelligence in 2023, how do you view the deployed infrastructure from the perspective of replacement cycles? In cases like GB300 or others, when will there be opportunities for upgrades?
A: People are still using Voltus, Pascal, and Amperes architectures because of the strong programmability of CUDA. For example, a major current use case is data processing and data organization. These architectures can be utilized for data processing, data organization, and machine learning-based searches to create training datasets for training. All these architectures are compatible with CUDA, and if there is existing infrastructure, low-intensity workloads can be placed on the previously installed devices, which can still perform well.
Q: NVIDIA expects the gross margin to reach the mid-70% level by the end of the fiscal year, with the gross margin having bottomed out in the second quarter. The company needs to improve by 200 basis points each quarter in the second half of the year, and it is currently unclear what the broad impact of tariffs on the semiconductor industry will be. What gives the company confidence in the trend of gross margin improvement in the second half of the year? How is NVIDIA's development in the AI field and its future growth trend?
A: NVIDIA's gross margin situation is quite complex, with many opportunities to improve gross margin in areas such as materials for the Blackwell system. Blackwell has many different configurations that help enhance gross margin. After achieving some strong business growth for customers, the company can start working on improving gross margin, and if improvements can be made in the short term, actions will be taken as soon as possible. The current tariff situation is still unclear, and further understanding of the U.S. government's plans, including timing, scope, and extent, is needed. The company will comply with export control and tariff-related regulations Currently, the market demand for Blackwell is very strong. AI is evolving from the perception and generation phase to the reasoning phase, introducing a new scaling law known as reasoning time or test time scaling, where the computational demand of reasoning models is 100 times that of traditional models. The company currently has three scaling laws driving AI computational demand: in addition to traditional AI scaling laws, there is post-training skill enhancement (the computational demand for reinforcement learning, fine-tuning, and model distillation far exceeds that of pre-training) as well as reasoning time scaling and reasoning (the computational demand for a single query reaches 100 times). The Blackwell platform can easily achieve the transition from pre-training, post-training to test time scaling, with its FP4 transformer engine, NVLink 72 expansion architecture, and new software technologies enabling the processing of reasoning AI models to be 25 times faster than Hopper, and all configurations are fully in production. The company believes it is currently at the beginning of reasoning AI and reasoning time scaling, marking the start of the AI era, with multimodal AI, enterprise AI, sovereign AI, and physical AI on the horizon, and the company will achieve strong growth by 2025. In the future, data centers will allocate most of their capital expenditures to accelerate computing and AI, increasingly becoming AI factories, with every company choosing to lease or operate independently.
Risk Disclosure and Statement of this article: Dolphin Research Disclaimer and General Disclosure