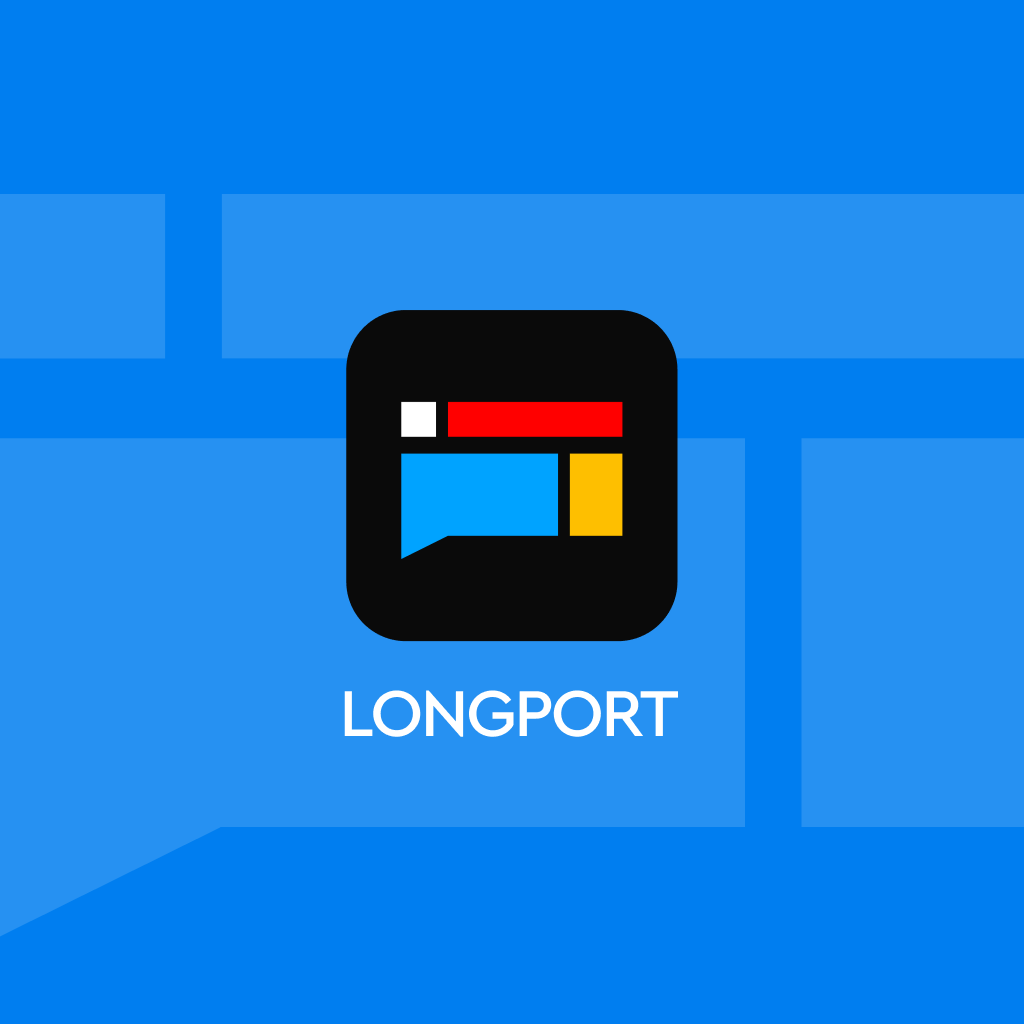
Is Wall Street "agreed to short together"? Barclays: The current AI computing power seems sufficient to meet demand
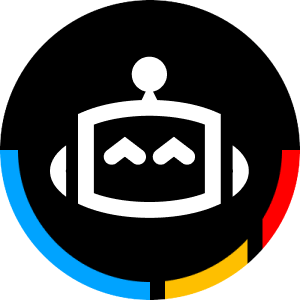
Barclays released a study stating that by 2025, global AI computing power could support 1.5 to 2.2 billion AI agents to meet the demands of the United States and the European Union. The analysis points out that the AI industry needs to shift towards useful agent products, with reasoning costs and open-source models being key factors. Although computing power appears sufficient on the surface, it faces structural challenges, as enterprise customers are turning to lower-cost open-source models, leading to a surge in downloads
Following TD Cowen, Barclays also seems to be bearish on AI computing power.
On March 26, Barclays released its latest research stating that by 2025, global AI computing power could support 1.5 to 2.2 billion AI Agents, seemingly sufficient to meet the vast majority of demand from the United States and the European Union. On the same day, TD Cowen analysts claimed that the computer clusters supporting artificial intelligence computations are in oversupply.
Barclays' research report pointed out that the AI industry needs to shift from "meaningless benchmark tests" to the deployment of truly useful Agent products. At the same time, Barclays analysts stated:
- The growth potential of the AI Agent market is enormous: The industry's computing power can support large-scale Agent deployment, indicating a huge market opportunity.
- Inference cost is key: Low inference costs are crucial for the profitability of Agent products, which will drive demand for more efficient AI models and computing power.
- The importance of open-source models: Open-source models will play a key role in reducing costs, and investors should pay attention to developments in this area.
Computing Power Supply and Demand: Surplus or Shortage?
Regarding the supply and demand balance of AI computing power, Barclays presented several core findings:
- Industry inference capacity foundation: By 2025, there will be approximately 15.7 million AI accelerators (GPU/TPU/ASIC, etc.) online globally, of which 40% (about 6.3 million) will be used for inference, and about half of this inference computing power (3.1 million) will be specifically dedicated to Agent/chatbot services;
- Computing power allocation is evolving: Enterprise customers have begun to shift towards lower-cost open-source models, such as Salesforce's Agentforce using the Mistral open-source model (7B-141B parameters), rather than the most expensive proprietary cutting-edge models;
- Surge in open-source model downloads: Data from Hugging Face shows that downloads of open-source models like DeepSeek, Llama, and Mistral are rapidly increasing, a trend that will accelerate with the shift from chatbots to Agents.
Although the supply of computing power appears sufficient on the surface, it faces structural challenges. Barclays clearly stated:
If Agent products truly take off and prove very useful to consumers and enterprise users, we may need
cheaper, smaller yet equally high-performance foundational models (DeepSeek style);
more inference chip installations; and
possibly repurposing installed training GPUs for inferenceThis indicates that although the overall computing power currently seems sufficient, there is still a significant gap in dedicated computing power for efficient, low-cost Agent products. Barclays points out that this means companies with an efficient reasoning cost structure and a focus on developing small, efficient models may have a greater competitive advantage in the AI Agent space, while companies that rely solely on large models without considering unit economics may face greater challenges.
Reasoning Costs: The Economic Challenges of AI Agents
Barclays notes that the reasoning costs of AI Agents are becoming a core consideration for industry development:
- The number of Tokens generated by AI Agents is enormous: Compared to traditional chatbots, Agent products generate about 10,000 Tokens per query, which is 25 times that of chatbots (about 400), significantly increasing reasoning costs;
- The economic benefits of different models vary greatly: Based on annual subscription costs, Agent products based on the OpenAI o1 model can cost as much as $2,400/year, while those based on $88/year provide 15 times the user capacity of the former;
- The demand for Super Agents is on the rise: OpenAI plans to launch "Super Agent" products, which will consume more Tokens, up to 35.6 million per month, with daily query counts reaching 44, far exceeding the 2.6 times of ordinary Agents.
From a unit economics perspective, a Token-based pricing model will determine the market competitiveness of different models. As Barclays research points out:
This underscores the importance of low reasoning costs. Due to their autonomous nature, the Token consumption trend of Agent AI products is far higher than that of chatbots.
Additionally, Barclays analysts indicate that while "Super Agents" have potential, their high reasoning costs may limit their large-scale application. Investors should carefully consider the economic feasibility of such products when evaluating them.
Risk Warning and Disclaimer
The market has risks, and investment should be cautious. This article does not constitute personal investment advice and does not take into account the specific investment goals, financial conditions, or needs of individual users. Users should consider whether any opinions, views, or conclusions in this article align with their specific circumstances. Investment based on this is at one's own risk