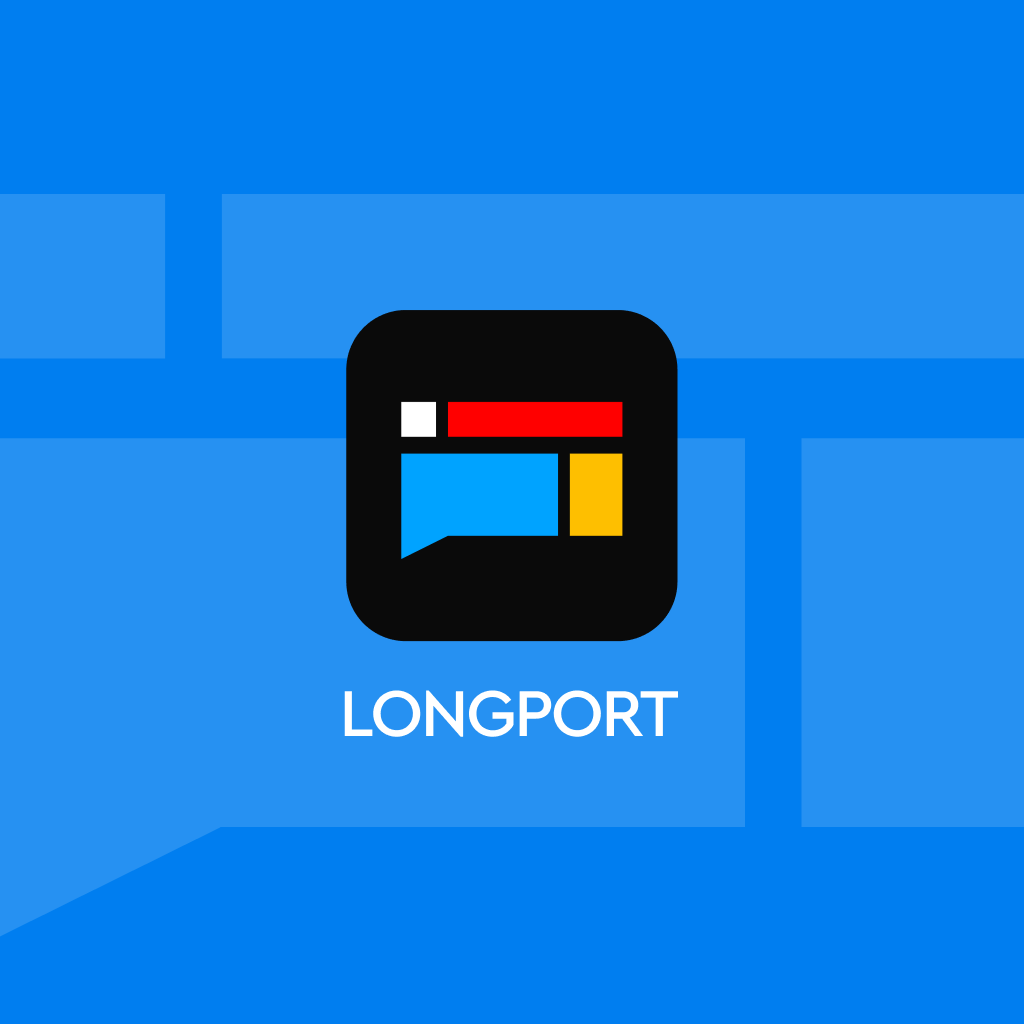
Alibaba released Qwen2.5-Omni, claiming to be proficient in all aspects of multimodal perception, including seeing, hearing, speaking, and writing
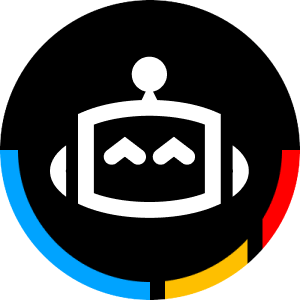
Alibaba has released Qwen2.5-Omni, its new generation multimodal flagship model capable of handling various input forms such as text, images, audio, and video, and generating text and natural speech synthesis output in real-time. The model adopts a new Thinker-Talker architecture, supporting real-time interaction and precise synchronization, demonstrating excellent audio capabilities and voice command following ability. Qwen2.5-Omni is now open-sourced on multiple platforms, allowing users to experience its powerful performance through a demo
Today, we released Qwen2.5-Omni, the next-generation end-to-end multimodal flagship model in the Qwen model family. This model is designed for comprehensive multimodal perception, capable of seamlessly handling various input forms such as text, images, audio, and video, and generating text and natural speech synthesis output simultaneously through real-time streaming responses.
The model is now open-sourced on Hugging Face, ModelScope, DashScope, and GitHub. You can experience the interactive features through our Demo or initiate voice or video chats directly via Qwen Chat, immersing yourself in the powerful performance of the new Qwen2.5-Omni model.
Key Features
-
All-in-one Innovative Architecture: We propose a brand-new Thinker-Talker architecture, an end-to-end multimodal model designed to support cross-modal understanding of text/image/audio/video while generating text and natural speech responses in a streaming manner. We introduced a new positional encoding technique called TMRoPE (Time-aligned Multimodal RoPE), achieving precise synchronization of video and audio inputs through time alignment.
-
Real-time Audio and Video Interaction: The architecture is designed to support fully real-time interaction, accommodating chunked input and instant output.
-
Naturally Smooth Speech Generation: It surpasses many existing streaming and non-streaming alternatives in terms of the naturalness and stability of speech generation.
-
Full Modal Performance Advantage: It demonstrates outstanding performance when benchmarked against single-modal models of equivalent scale. Qwen2.5-Omni outperforms similarly sized Qwen2-Audio in audio capabilities and maintains parity with Qwen2.5-VL-7B.
-
Exceptional End-to-End Voice Command Following Ability: Qwen2.5-Omni exhibits performance in end-to-end voice command following comparable to text input processing, excelling in benchmark tests such as MMLU general knowledge understanding and GSM8K mathematical reasoning.
Qwen2.5-Omni-7B demo
Model Architecture
Qwen2.5-Omni adopts a Thinker-Talker dual-core architecture. The Thinker module acts like the brain, responsible for processing multimodal inputs such as text, audio, and video, generating high-level semantic representations and corresponding text content; the Talker module resembles the vocal organs, receiving the semantic representations and text output from the Thinker in a streaming manner, smoothly synthesizing discrete speech units. The Thinker is based on a Transformer decoder architecture, integrating audio/image encoders for feature extraction; the Talker employs a dual-track autoregressive Transformer decoder design, directly receiving high-dimensional representations from the Thinker during training and inference, sharing all historical context information, forming an end-to-end unified model architecture
Model Architecture Diagram
Model Performance
Qwen2.5-Omni outperforms similarly sized unimodal models and closed-source models, such as Qwen2.5-VL-7B, Qwen2-Audio, and Gemini-1.5-pro, across various modalities including images, audio, and audiovisual.
In the multimodal task OmniBench, Qwen2.5-Omni achieved state-of-the-art performance. Additionally, in unimodal tasks, Qwen2.5-Omni excelled in multiple domains, including speech recognition (Common Voice), translation (CoVoST2), audio understanding (MMAU), image reasoning (MMMU, MMStar), video understanding (MVBench), and speech generation (Seed-tts-eval and subjective natural listening).
Model Performance Chart
Next Steps
We look forward to hearing your feedback and seeing the innovative applications you develop using Qwen2.5-Omni. In the near future, we will focus on enhancing the model's ability to follow voice commands and improving audiovisual collaborative understanding. More excitingly, we will continue to expand the boundaries of multimodal capabilities to develop into a comprehensive general model!
Ways to Experience
-
Qwen Chat: https://chat.qwenlm.ai
-
Hugging Face: https://huggingface.co/Qwen/Qwen2.5-Omni-7B
-
ModelScope: https://modelscope.cn/models/Qwen/Qwen2.5-Omni-7B
-
DashScope: https://help.aliyun.com/zh/model-studio/user-guide/qwen-omni
-
GitHub: https://github.com/QwenLM/Qwen2.5-Omni
-
Demo Experience: https://modelscope.cn/studios/Qwen/Qwen2.5-Omni-Demo
This article is sourced from: Tongyi Qianwen Qwen, original title: "Qwen2.5-Omni: Look, Listen, Speak, Write, Mastering Everything!".
Risk Warning and Disclaimer
The market has risks, and investment requires caution. This article does not constitute personal investment advice and does not take into account the specific investment objectives, financial conditions, or needs of individual users. Users should consider whether any opinions, views, or conclusions in this article are suitable for their specific circumstances. Investment based on this is at one's own risk