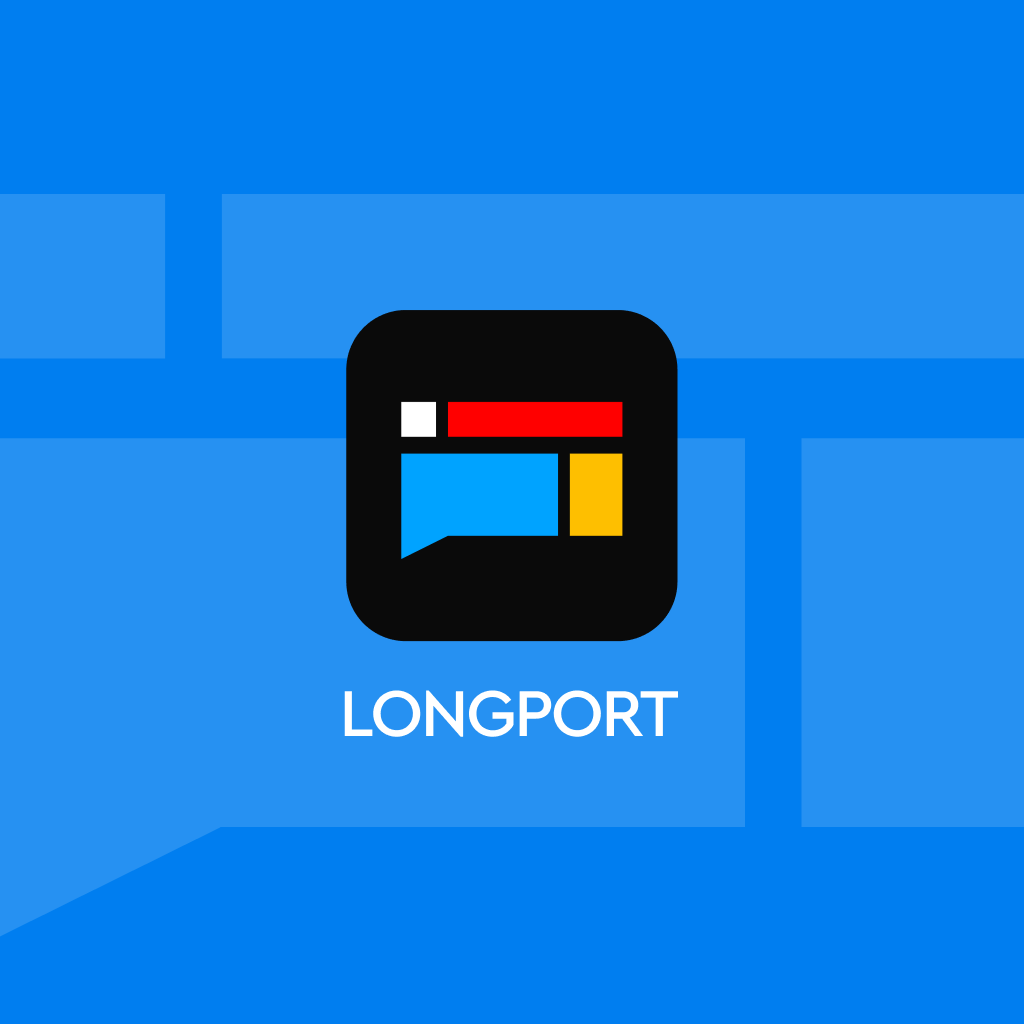
Embracing the era of physical AI, "Hangzhou Six Little Dragons" Quantum Core Technology launches the open-source spatial understanding model SpatialLM
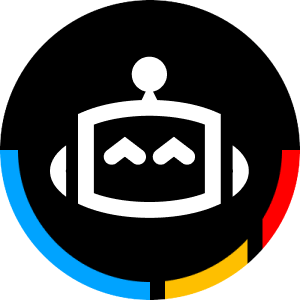
At the GTC 2025 Global Artificial Intelligence Conference held in the United States, NVIDIA CEO Jensen Huang introduced the future of physical AI. Hangzhou Qunhe Technology announced the open-source of its spatial understanding model SpatialLM, which can extract point cloud data from videos to generate physically accurate 3D scene layouts, reducing the training costs for robots. SpatialLM works in conjunction with the SpatialVerse platform, which will be released in 2024, enabling the transformation of real-world data into new scenes in virtual environments. Although there are similar models in the industry, SpatialLM has advantages in spatial information cognitive understanding
Recently, the GTC 2025 Global Artificial Intelligence Conference hosted by NVIDIA is being held in the United States, with physical AI becoming one of the key terms.
NVIDIA CEO Jensen Huang introduced the development history of AI research and development at GTC, from the initial conscious AI to the current generative AI, and it is expected to soon enter the era of agent-based AI, followed by the era of physical AI, which is the era of robots.
Before the arrival of the physical AI era, how to enable robots to possess the core capability of truly "understanding the world" is a key focus for the industry.
At this conference, "Hangzhou AI Six Little Dragons" Qunhe Technology announced the open-source release of its self-developed spatial understanding model SpatialLM, which is now available on platforms such as HuggingFace, GitHub, and Modao Community.
Qunhe Technology introduced to Xinfeng that based on point cloud data extracted from videos, the SpatialLM model can accurately perceive and understand the structured scene information within, and generate physically correct 3D scene layouts based on this video.
This allows enterprises to fine-tune the SpatialLM model for specific scenes, lowering the training threshold for embodied intelligence.
This model works synergistically with Qunhe Technology's spatial intelligence platform SpatialVerse, which will be released in 2024.
The SpatialLM model transforms data from the real world into rich scenes in a virtual environment through the conversion from video to structured scenes. Based on the synthetic data engine of SpatialVerse, a structured scene can generalize to generate billions of new scenes.
This means that a scene based on the real world can evolve into countless new scenes, significantly reducing the training costs for robots.
However, Qunhe Technology's SpatialLM is not unique, as similar parameter models have already been launched by companies in the industry.
In 2024, Meta released the SceneScript model, which can convert real-world scenes into geometric representations and expand scenes.
According to Qunhe Technology personnel, compared to SceneScript, the significant advantage of SpatialLM lies in its support for cognitive understanding of spatial information in the physical world and structured descriptions; in terms of input, SceneScript relies on Meta's customized glasses, while SpatialLM is designed for input from ordinary cameras and mobile phone videos, making it more versatile.
In terms of spatial and embodied intelligence training, Qunhe Technology has already established partnerships with leading technology companies in Silicon Valley.
In terms of capitalization, Qunhe Technology submitted an IPO application to the Hong Kong Stock Exchange in February this year, and is expected to become the first listed company among the "Hangzhou Six Little Dragons."
Risk Warning and Disclaimer
The market has risks, and investment should be cautious. This article does not constitute personal investment advice and does not take into account the specific investment goals, financial situation, or needs of individual users. Users should consider whether any opinions, views, or conclusions in this article are suitable for their specific circumstances. Investment based on this is at one's own risk