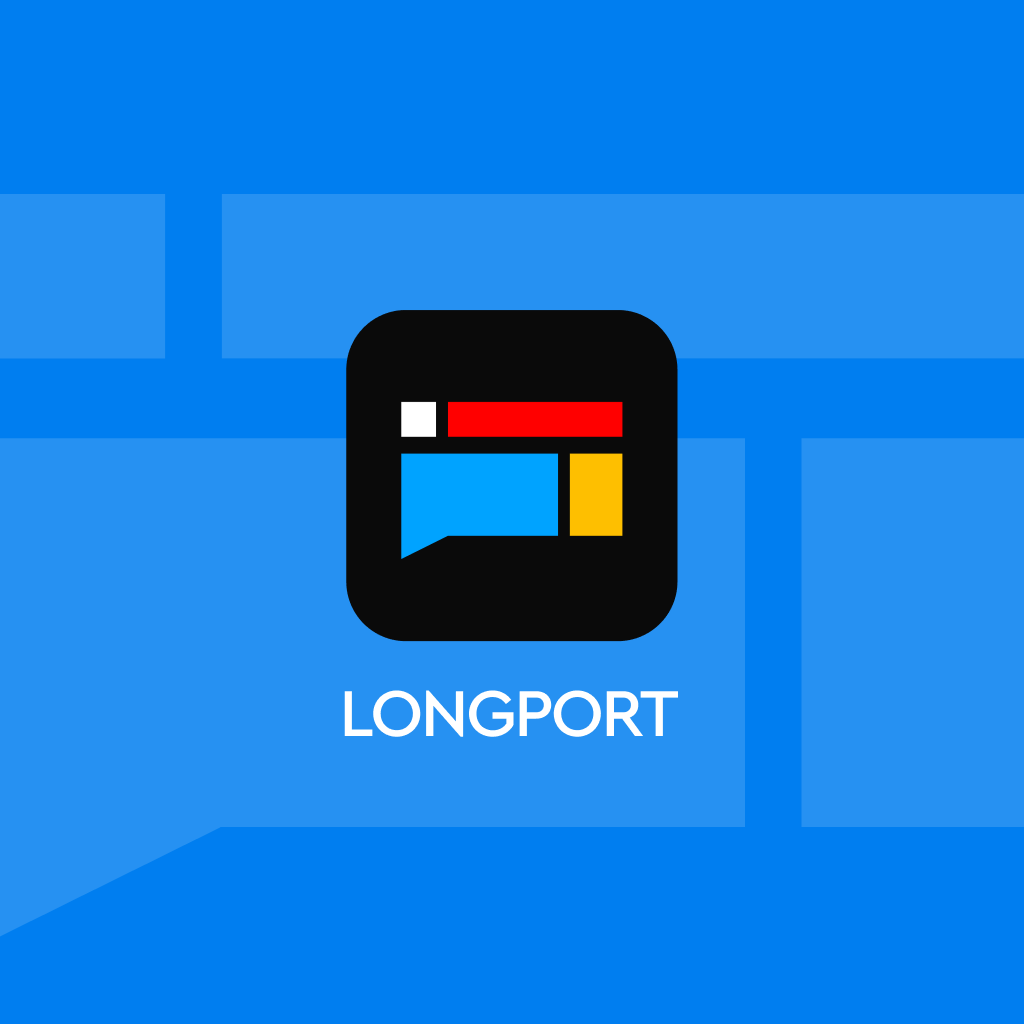
"Carbon-based" VS "Silicon-based": The "life-and-death race" of human-machine in the secondary market
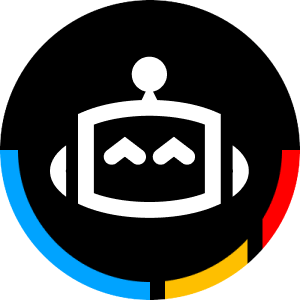
In the financial industry, artificial intelligence is rapidly changing traditional investment research processes and may replace 90% of fund managers and analysts in the next five to ten years. The efficient data processing and logical output capabilities of AI give it an advantage in generating investment recommendations. Although the application of AI is relatively mature in manufacturing and insurance, its penetration in areas such as sell-side research and investment banking still faces challenges
At midnight, when the diligent fund manager finished the last roadshow and read the latest research report, closing his laptop, his "digital counterpart" was quietly growing in some large model base.
From simple data processing tools to reading research reports and extracting key points, and then logically and systematically outputting some investment suggestions. Over the past year, AI programs have rapidly crossed several initial stages and have begun to shake the traditional investment research process that has lasted for fifty or sixty years.
While the young people on Financial Street are still fighting their biological clocks with nicotine and caffeine, AI algorithms, with their ever-awake "digital brains" and the ability to output tens of thousands of words in minutes, are about to start "challenging" their workstations.
Will they really replace him (her)?
"Yes, within ten years, 90% of fund managers and analysts in this market will disappear," predicted an investment director at a public fund institution.
But some research directors disagree with his prediction.
"It won't take ten years; five years will be enough."
Once the Most Solid "Fortress"
The replacement of ordinary human jobs by artificial intelligence did not start now; this trend has long been underway in traditional manufacturing.
In Geely's Xi'an factory's welding workshop, 886 welding robots complete body welding with an accuracy of 0.5 mm, combined with 650 AGV intelligent logistics vehicles, reducing a production line that originally required 300 people to less than 50.
Ansteel Group's "dark factory" is even more thorough; in the mining company's work area, intelligent inspection robots and cleaning robots flash lights and work orderly everywhere; in the cold-rolled color-coated work area, the entire production line is empty, with various robots working alongside unmanned overhead cranes.
Even in the financial industry, such as insurance, AI customer service systems and policy review systems have already been widely adopted in large institutions. Nowadays, when reporting car insurance claims, almost 100% of the first contact for customers is with artificial intelligence.
However, in the most important areas of the financial industry, such as sell-side research, proprietary asset management, and investment banking, the penetration of artificial intelligence has not seen significant breakthroughs over the years.
Since the 1970s, the workflow of a sell-side analyst has been fixed: collecting data and information—organizing data and information—integrating research reports—publishing and communicating through roadshows.
This process was introduced from overseas to China at the end of the last century, combined with the vast number of listed companies in the Chinese capital market, astonishing liquidity, and unprecedented numbers of institutional investors, creating a sell-side research market scale rarely seen overseas.
At its peak, a top domestic sell-side research institution could employ nearly a thousand people (including sales and service teams). A research group in a large industry could have more than ten analysts leading over a dozen interns, responsible for tracking and researching the movements of hundreds of key companies.
For a long time, the "digitalization" of domestic sell-side research institutions was mainly reflected in the use of EXCEL plugins and changing the communication method of report publication from phone calls to WeChat groups This industry is almost one of the few "job fortresses" that has not been substantially impacted by artificial intelligence to date.
What Factors Block AI?
What reasons keep AI outside the core business systems of finance?
After engaging with several large asset management institutions, Zhi Shi Tang has gathered the following viewpoints:
First, there are a large number of "non-standard" work details in investment research positions. Simple AI systems cannot replace these roles. The efficiency of training a smart young person is much faster than training an intelligent system.
Second, financial work has high compliance and security requirements. Financial business data involves a lot of sensitive information, and using AI to process this data may pose a risk of leakage, especially when data protection regulations are inadequate.
Third, financial work requires a high level of professionalism and maturity, with a low tolerance for error. Situations like AI "hallucinations" can lead to very serious consequences.
Fourth, the financial industry traditionally relies heavily on human experience and communication, which is closely tied to strict legal responsibilities. Especially in high-risk decision-making, people may trust human experts more than algorithms. This cultural resistance may delay the adoption of AI.
However, as Deepseeks grow day and night, are these once "barriers" really that solid?
The Once "Fortress" Has Started to Show Cracks
Although there are various moats, quietly, this fortress has long shown signs of cracks.
According to Zhi Shi Tang, within some top asset management institutions, AI robots have already begun to play a role, but they are "secretive" about it externally.
In these institutions, AI robots have started to partially replace researchers in participating in online roadshows.
They often log into the system under the identity of a specific researcher in the actual team, then quietly "squat" in the corner of the meeting, meticulously recording all the spoken content and Q&A.
More advanced AIs may even summarize research points and extract "keywords" and "important content" based on the investment team's requirements.
Although the work currently "completed" by AI is still relatively rough, it has significantly reduced the consumption of human labor.
Especially in the face of the current massive number of roadshows and reverse roadshows from listed companies, the diligent, tireless, and multi-threaded advantages of AIs have already emerged.
Additionally, some teams have reported that in important thematic research, although AI researchers may not be as logical or good at inductive analysis as humans, their ability to gather relevant content from vast amounts of information and integrate it to a certain extent still demonstrates value, especially when the timeliness of the topic is high and the workload is enormous.
The Advantages and Disadvantages of "No Emotion"
In the trading and order placement stages, especially when combined with quantitative strategies, the application space for AI intelligent robots may be even larger.
For example, in the trading phase, AI intelligent robots can perform tasks such as splitting orders, placing orders, grabbing orders, and canceling orders in an "intelligent" manner, and they do so with high efficiency In addition, when designing trading strategies, AI robots are almost unaffected by the "emotions" of the previous trades, which provides advantages in many situations.
In some large quantitative private equity institutions, the types of "jobs" that AI can participate in may be more diverse—because, in terms of trading frequency and compliance requirements, they sometimes have more flexibility than public funds.
In this sense, the emergence of a groundbreaking AI general model like Deepseek in a "related" company of a top private equity institution is not unexpected, but rather logical.
Moreover, within the realm of subjective investment, AI may also have its own application scenarios.
“At some point in the future, it is possible that someone will train a trading robot in the style of Buffett using his trading cases,” said a fund manager.
So, is human labor still needed?
The Combination of Subjectivity and AI is the Current Demand
Although the incoming vision is surprising, the current reality seems to focus on how to combine the "subjective abilities" of experienced investment researchers with the "characteristics" of AI.
For example, how AI, represented by DeepSeek, can assist researchers in their studies and help fund managers in their investments.
In September 2024, Zheshang Fund explained how their AI helps fund managers make investments:
AI does not make predictions; its analytical actions are based on existing data for more comprehensive analysis.
For instance, when a certain stock starts to surge, AI dissects the investment logic of the fund to determine whether it is benefiting from an industry trend or its own value potential that is generating profits. The AI robot gradually learns what qualities excellent stocks possess and, based on its powerful data computing capabilities, makes various historical assumptions and tests to provide subsequent investment signals.
Moreover, according to their charts, the subjective research team can monitor over 10 industries, while the AI model can track the dynamics of hundreds of sub-industries.
In terms of landing core business scenarios, CICC Wealth stated that DeepSeek demonstrates significant efficiency advantages. Through the natural language processing and event reasoning capabilities of the large model, it can automate the parsing of unstructured texts such as policy interpretations, industry research reports, and announcements from listed companies, assisting investment advisors in efficiently completing market trend analyses and macro interpretations, significantly improving information processing efficiency and decision-making accuracy, with a daily processing volume exceeding 10,000 documents, enhancing efficiency by 90% compared to traditional manual processing.
Hua Tian Fu Fund recently announced that it has completed the privatization deployment of the DeepSeek series of open-source models, which will be applied in core business scenarios such as investment research, product sales, risk control compliance, and customer service.
Perhaps, that surging era is already approaching! Risk Warning and Disclaimer
The market has risks, and investment should be cautious. This article does not constitute personal investment advice and does not take into account the specific investment goals, financial situation, or needs of individual users. Users should consider whether any opinions, views, or conclusions in this article are suitable for their specific circumstances. Investment based on this is at one's own risk