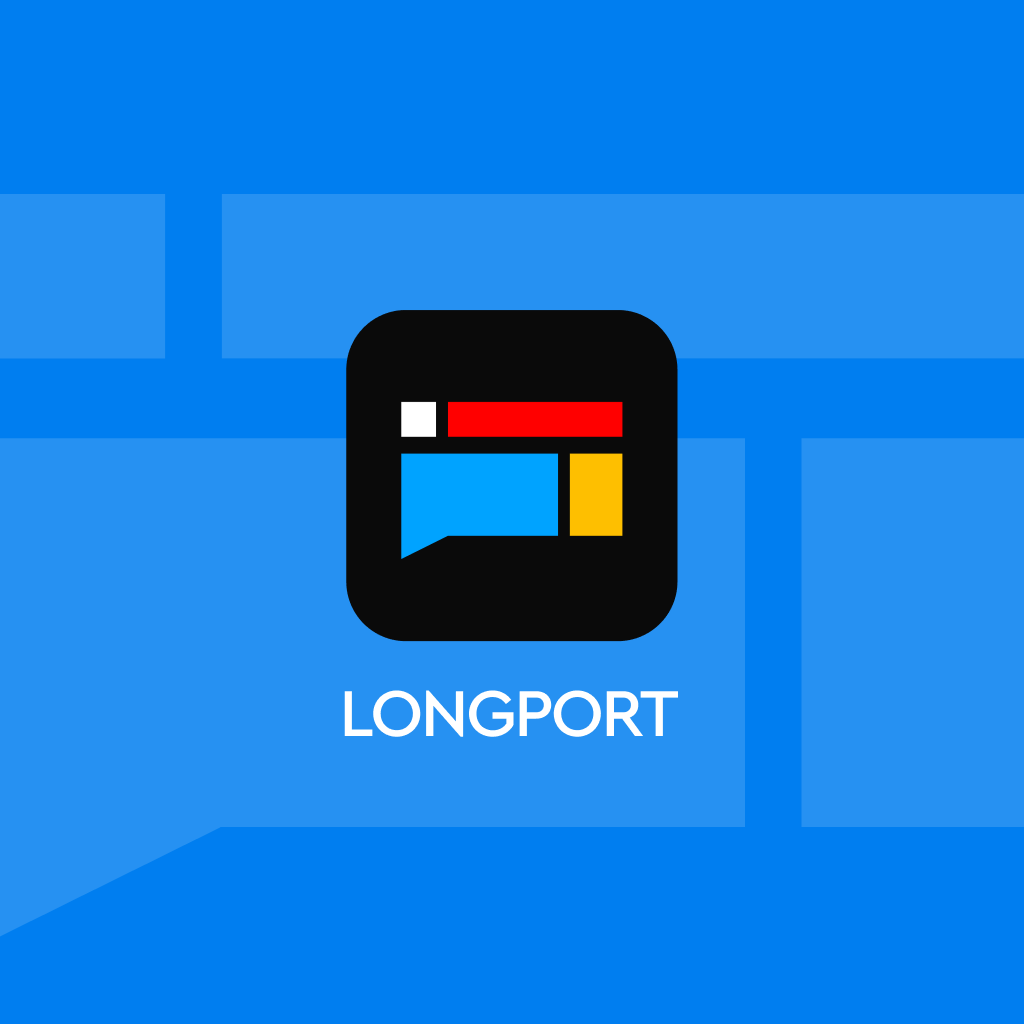
In-depth interpretation of Jensen Huang's GTC speech: Comprehensive "optimization for inference," "the more you buy, the more you save," NVIDIA is the cheapest!
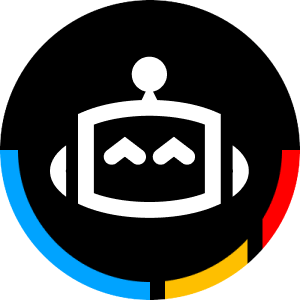
Semianalysis stated that at the GTC 2025 conference, the innovations introduced by NVIDIA, such as the inference Token extension, inference stack and Dynamo technology, and Co-Packaged Optics (CPO) technology, will significantly reduce the total cost of ownership for AI, greatly lowering the deployment costs of efficient inference systems and consolidating NVIDIA's leading position in the global AI ecosystem
On Tuesday, March 18 local time, NVIDIA CEO Jensen Huang delivered a keynote speech at the NVIDIA AI conference GTC 2025 held in San Jose, California. The well-known American semiconductor consulting firm Semianalysis provided an in-depth interpretation of Huang's GTC speech, detailing NVIDIA's latest progress in enhancing AI inference performance.
Market concerns revolve around the possibility that software optimizations like DeepSeek and significant cost savings from NVIDIA-led hardware advancements may lead to a decline in demand for AI hardware. However, price impacts demand; as AI costs decrease, the boundaries of AI capabilities are continuously broken, leading to increased demand.
With NVIDIA's improvements in inference efficiency in both hardware and software, the deployment costs for model inference and intelligent agents have significantly decreased, resulting in a diffusion effect of cost benefits, which in turn increases actual consumption, as NVIDIA's slogan states: "Buy more, save more."
The following are the core points of the article:
Inference Token Expansion: The synergy of pre-training, post-training, and inference-time expansion laws continuously enhances AI model capabilities.
Jensen Huang's Mathematical Rules: Including FLOPs sparsity rate, bidirectional bandwidth measurement, and new rules for calculating the number of GPUs based on the number of GPU chips in packaging.
GPU and System Roadmap: Introduced key specifications and performance improvements of Blackwell Ultra B300, Rubin, and Rubin Ultra, emphasizing breakthroughs in performance, memory, and network interconnect for the next generation of products.
Launched Inference Stack and Dynamo Technology: New features such as intelligent routers, GPU planners, improved NCCL, NIXL, and NVMe KVCache offload managers greatly enhance inference throughput and efficiency.
Co-Packaged Optics (CPO) Technology: Detailed the advantages of CPO in reducing power consumption, increasing switch base numbers, and network flattening, as well as its potential in future large-scale network deployments.
The article points out that these innovations will significantly reduce the total cost of ownership for AI, drastically lowering the deployment costs of efficient inference systems and solidifying NVIDIA's leading position in the global AI ecosystem.
Semianalysis In-Depth Interpretation Full Text for AI Translation
Inference Token Explosion
The advancement of artificial intelligence models is accelerating rapidly, with improvements in the past six months surpassing those of the previous six months. This trend is expected to continue, as three expansion laws—pre-training expansion, post-training expansion, and inference-time expansion—are working in synergy to drive this process.
This year's GTC (GPU Technology Conference) will focus on addressing new expansion paradigms.
Source: NVIDIA
Claude 3.7 has demonstrated remarkable performance in the field of software engineering. Deepseek v3 shows that the costs of the previous generation of models are plummeting, which will further drive their widespread applicationThe o1 and o3 models of OpenAI demonstrate that extending reasoning time and search capabilities significantly improves answer quality. As early shown by the pre-training law, there is no upper limit to increasing computational resources in the post-training phase. This year, Nvidia is committed to significantly improving inference cost efficiency, aiming for a 35-fold improvement in inference costs to support model training and deployment.
Last year's market slogan was "the more you buy, the more you save," but this year's slogan has changed to "the more you save, the more you buy." Nvidia's improvements in inference efficiency in both hardware and software have greatly reduced the deployment costs of model inference and intelligent agents, resulting in a diffusion effect of cost-effectiveness, which is a classic manifestation of the Jevons Paradox.
What the market is concerned about is that the significant cost savings brought by DeepSeek-style software optimization and Nvidia-led hardware advancements may lead to a decline in demand for AI hardware, potentially resulting in an oversupply of Tokens in the market. Prices will affect demand; as AI costs decrease, the boundaries of AI capabilities are continuously broken, leading to an increase in demand. Currently, AI capabilities are limited by inference costs, and as costs decrease, actual consumption may increase.
Concerns about Token deflation are similar to discussions about the declining connection costs of each data packet in fiber-optic internet, while overlooking the ultimate impact of websites and internet applications on our lives, society, and economy. The key difference is that bandwidth has an upper limit, whereas with significant improvements in capabilities and decreasing costs, the demand for AI can grow infinitely.
The data provided by Nvidia supports the view of the Jevons Paradox. The number of Tokens in existing models exceeds 100 trillion, while the Token count of an inference model is 20 times that, and the computational load is 150 times higher.
Source: NVIDIA
The computation required during testing needs hundreds of thousands of Tokens per query, with hundreds of millions of queries each month. In the post-training expansion phase, where the model is "in school," each model needs to process trillions of Tokens, along with hundreds of thousands of post-training models. Additionally, AI with agent capabilities means multiple models will work together to solve increasingly complex problems.
Jensen Huang's Mathematics Changes Every Year
Every year, Jensen Huang introduces new mathematical rules. This year's situation is more complex, and we observe a third new rule of Jensen Huang's mathematics.
The first rule is that the FLOPs data released by Nvidia is measured at a 2:4 sparsity (which is actually not used), while the true performance metric is dense FLOPs—meaning that the H100 is reported at 989.4 TFLOPs under FP16, while the actual dense performance is about 1979.81 TFLOPs.
The second rule is that bandwidth should be measured in bidirectional bandwidth. The bandwidth of NVLink5 is reported as 1.8TB/s because its sending bandwidth is 900GB/s, plus a receiving bandwidth of 900GB/s. Although these figures are added in the specifications, in the networking field, the standard is measured in unidirectional bandwidthNow, the third mathematical rule from Jensen Huang has emerged: the number of GPUs will be counted based on the number of GPU chips in the package, rather than the number of packages. This naming convention will be adopted starting with the Rubin series. The first generation Vera Rubin rack will be referred to as NVL144, even though its system architecture is similar to the GB200 NVL72, but it uses the same Oberon rack and 72 GPU packages. This new counting method, while perplexing, is a change we must accept in Jensen Huang's world.
Now, let's review the roadmap.
GPU and System Roadmap
Source: NVIDIA
Blackwell Ultra B300
Source: NVIDIA
The Blackwell Ultra 300 has been previewed, and the details are essentially consistent with what we shared last Christmas. The main specifications are as follows: the GB300 will not be sold as a single board but will appear as a B300 GPU in a portable SXM module, also featuring a Grace CPU in a portable BGA form. In terms of performance, the B300 improves over 50% in FP4 FLOPs density compared to the B200. The memory capacity is upgraded to 288GB per package (8 x 12-Hi HBM3E stacks), but the bandwidth remains unchanged at 8 TB/s. The key to achieving this goal lies in reducing many (but not all) FP64 computation units and replacing them with FP4 and FP6 computation units. Double-precision workloads are primarily used for HPC and supercomputing, rather than AI. While this disappoints the HPC community, Nvidia is shifting its focus to emphasize the more important AI market.
The B300 HGX version is now referred to as B300 NVL16. This will adopt the previously known single GPU version "B300A," now simply called "B300." Since the single B300 does not have a high-speed D2D interface connecting the two GPU chips, there may be more communication overhead.
The B300 NVL16 will replace the B200 HGX form, featuring 16 packages and GPU chips on a single board. To achieve this, 2 single-chip packages are placed on each SXM module, totaling 8 SXM modules. It is unclear why Nvidia did not continue with the 8× dual-chip B300 and chose this approach; we suspect it is to improve yield from smaller CoWoS modules and packaging substrates. Notably, this packaging technology will use CoWoS-L instead of CoWoS-S, which is a significant decision. The maturity and capacity of CoWoS-S are the reasons for the single B300A, and this shift indicates that CoWoS-L has rapidly matured, with its yield stabilizing compared to the initial low levelsThese 16 GPUs will communicate via the NVLink protocol, similar to the B200 HGX, with two NVSwitch 5.0 ASICs located between the two arrays of the SXM module.
A new detail is that, unlike previous HGXs, the B300 NVL16 will no longer use Astera Labs' retimers. However, some hyperscale cloud service providers may choose to add PCIe switches. We disclosed this information to Core Research subscribers earlier this year.
Another important detail is that the B300 will introduce the CX-8 NIC, which provides four 200G channels, with a total throughput of 800G, offering next-generation network speeds for InfiniBand, doubling the performance of the existing CX-7 NIC.
Rubin Technical Specifications
Source: NVIDIA
Source: Semianalysis
Rubin will utilize TSMC's 3nm process, featuring two reticle-size compute chips, each equipped with two I/O Tiles, incorporating all NVLink, PCIe, and NVLink C2C IP to free up more space on the main chip for computation.
Rubin offers an incredible 50 PFLOPs of dense FP4 computing performance, more than tripling the generational performance of the B300. How does Nvidia achieve this? They expand through several key vectors:
As mentioned, the area freed up by the I/O chips may increase by 20%-30%, allowing for more stream processors and tensor cores.
Rubin will adopt a 3nm process, possibly using a custom Nvidia 3NP or standard N3P. The transition from 3NP to 4NP significantly enhances logic density, but SRAM sees little reduction.
Rubin will have a higher TDP—estimated at around 1800W—which may even drive higher clock frequencies.
Structurally, Nvidia's generational expansion of the tensor core systolic array will further increase: from Hopper's 32×32 to Blackwell's 64×64, Rubin may expand to 128×128. A larger systolic array provides better data reuse and lower control complexity while being more efficient in area and power consumption. Although programming difficulty increases, Nvidia achieves extremely high parameter yield with built-in redundancy and repair mechanisms, ensuring overall performance even if individual compute units fail. This is different from TPUs, which do not have the same fault tolerance with their ultra-large tensor cores
Source: Semianalysis
Rubin will continue to use the Oberon rack architecture, similar to the GB200/300 NVL72, and will be equipped with the Vera CPU—the 3nm successor to Grace. It is important to note that the Vera CPU will use Nvidia's fully custom cores, while Grace heavily relies on Arm's Neoverse CSS cores. Nvidia has also developed a custom interconnect system that allows a single CPU core to access more memory bandwidth, which is difficult for AMD and Intel to compete with.
This is the origin of the new naming convention. The new rack will be named VR200 NVL144. Although the system architecture is similar to the previous GB200 NVL72, Nvidia is changing the way we count GPU numbers because each package contains 2 compute chips, totaling 144 compute chips (72 packages × 2 compute chips/package)!
As for AMD, its marketing team needs to be aware that there is an omission in AMD's claim that the MI300X family can scale to 64 GPUs (8 packages per system × 8 XCD chipsets per package), which is a key market opportunity.
HBM and Interconnect
Nvidia's HBM capacity will remain at 288GB generation after generation, but will upgrade to HBM4: 8 stacks, each 12-Hi, with a layer density of 24GB/layer. The application of HBM4 allows for an increase in total bandwidth, with a total bandwidth of 13TB/s primarily benefiting from the bus width doubling to 2048 bits, with a pin speed of 6.5Gbps, compliant with JEDEC standards.
Source: Semianalysis
The speed of NVLink sixth generation has doubled to 3.6TB/s (bidirectional), resulting from the doubling of the number of channels, with Nvidia still using 224G SerDes.
Returning to the Oberon rack, the backplane still uses a copper backplane, but we believe the number of cables has also increased accordingly to accommodate the doubling of the number of GPU channels.
On the NVSwitch front, the new generation NVSwitch ASIC will also achieve a doubling of total bandwidth by doubling the number of channels, further enhancing the performance of the switch.
Rubin Ultra Specifications
Source: NVIDIA
Rubin Ultra is a stage with significant performance improvements. Nvidia will directly use 16 HBM stacks in one package, increasing from 8 to 16. The entire rack will consist of 4 mask-sized GPUs, with 2 I/O chips in the middle. The computing area is doubled, and the computing performance is also doubled to 100 PFLOPs of dense FP4 performance. The HBM capacity is increased to 1024GB, more than 3.5 times that of the standard Rubin. It adopts a dual-stacking design, while both density and layers are also increased. To achieve 1TB of memory, there will be 16 HBM4E stacks in the package, each stack containing 16 layers of 32Gb DRAM core chips.
We believe that this package will be split into two interconnectors placed on the substrate to avoid using one oversized interconnector (almost 8 times the mask size). The two GPU chips in the middle will be interconnected via thin I/O chips, with communication achieved through the substrate. This requires an oversized ABF substrate, whose size exceeds the current JEDEC package size limits (both width and height are 120mm).
The system has a total of 365TB of high-speed storage, with each Vera CPU having 1.2TB LPDDR, totaling 86TB (72 CPUs), leaving about 2TB of LPDDR for each GPU package as additional secondary memory. This is an implementation of custom HBM base functionality. The LPDDR memory controller is integrated into the base core, serving the additional secondary memory, which is located on the board's LPCAMM module, working in conjunction with the secondary memory carried by the Vera CPU.
Source: Semianalysis
This is also when we will see the launch of the Kyber rack architecture.
Kyber Rack Architecture
The key new feature of the Kyber rack architecture is that Nvidia increases density by rotating the rack 90 degrees. For the NVL576 (144 GPU package) configuration, this is another significant enhancement for large-scale network expansion.
Source: NVIDIA
Let's take a look at the key differences between the Oberon rack and the Kyber rack:
Source: Semianalysis
· The computing tray is rotated 90 degrees, forming a shape similar to a cartridge, thus achieving higher rack density.
· Each rack consists of 4 silos, with each silo including two layers of 18 computing cards
For NVL576, each compute card contains one R300 GPU and one Vera CPU.
Each silo has a total of 36 R300 GPUs and 36 Vera CPUs.
This brings the world scale of NVLink to 144 GPUs (576 chips).
The PCB backplane replaces the copper wire backplane as a key component for the expansion link between the GPU and NVSwitch.
This change is mainly due to the difficulty of routing cables in a smaller footprint.
Source: NVIDIA
There are signs that a variant of the Kyber rack with VR300 NVL1,152 (288 GPU packages) has appeared in the supply chain. If calculated based on the number of wafers mentioned in the GTC keynote, you will see the 288 GPU packages marked in red. We believe this could be a future SKU, with rack density and NVLink world scale doubling from the showcased NVL576 (144 packages) to NVL1,152 (288 packages).
Additionally, there is a brand new seventh-generation NVSwitch, which is noteworthy. This is the first introduction of NVSwitch in the mid-platform, enhancing both the total bandwidth and base, scalable to 576 GPU chips (144 packages) within a single domain. However, the topology may no longer be a fully interconnected single-level multi-plane structure, but may shift to a two-level multi-plane network topology with oversubscription, or even adopt a non-CloS topology.
Blackwell Ultra Improved Exponential Hardware Units
Various attention mechanisms (such as flash-attention, MLA, MQA, and GQA) require matrix multiplication (GEMM) and softmax functions (row reduction and element-wise exponential operations).
In GPUs, GEMM operations are primarily executed by tensor cores. Although the performance of tensor cores has continuously improved with each generation, the enhancement of the multifunctional unit (MUFU) responsible for softmax calculations has been relatively small.
In bf16 (bfloat16) Hopper, calculating the softmax for the attention layer requires 50% of the GEMM cycles. This necessitates kernel engineers to "hide" the latency of softmax through overlapping computations, making kernel writing exceptionally challenging.
Source: Tri Dao @ CUDA Mode Hackathon 2024
In FP8 (floating point 8-bit) Hopper, the cycles required for softmax calculations in the attention layer are the same as those for GEMM. Without any overlap, the computation time for the attention layer would double, requiring approximately 1536 cycles to compute matrix multiplication, plus another 1536 cycles to compute softmaxThis is the key to improving throughput through overlapping technology. Since the cycles required for softmax and GEMM are the same, engineers need to design perfectly overlapping kernels, but achieving this ideal state is difficult in reality. According to Amdahl's Law, perfect overlap is hard to achieve, which compromises hardware performance.
In the world of Hopper GPUs, this challenge is particularly evident, and the first generation of Blackwell faces similar issues. Nvidia addressed this problem with Blackwell Ultra, redesigning the SM (Streaming Multiprocessor) and adding new instructions, resulting in a 2.5 times speedup in MUFU calculations for the softmax portion. This reduces the reliance on perfect overlapping computations, giving CUDA developers more room for error when writing attention kernels.
Source: Tri Dao @ CUDA Mode Hackathon 2024
This is where Nvidia's new inference stack and Dynamo technology shine.
Inference Stack and Dynamo
At last year's GTC, Nvidia discussed how the large-scale GPU expansion of the GB200 NVL72 increased inference throughput by 15 times compared to the H200 under FP8.
Source: NVIDIA
Nvidia has not slowed down but has accelerated the improvement of inference throughput in both hardware and software domains.
The Blackwell Ultra GB300 NVL72 improves FP4 dense performance by 50% compared to the GB200 NVL72, while HBM capacity also increases by 50%, both of which will enhance inference throughput. The roadmap also includes multiple upgrades in network speed within the Rubin series, which will significantly improve inference performance.
The next leap in hardware for inference throughput will come from the expanded network scale in Rubin Ultra, which will increase from 144 GPU chips (or packages) in Rubin to 576 GPU chips, which is just part of the hardware improvements.
On the software side, Nvidia has launched Nvidia Dynamo—an open AI engine stack designed to simplify inference deployment and scaling. Dynamo has the potential to disrupt existing VLLM and SGLang, offering more features and higher performance. Combined with hardware innovations, Dynamo will further shift the curve between inference throughput and interactivity to the right, especially improving scenarios that require higher interactivity.
Source: NVIDIA
Dynamo has introduced several key new features:
·Smart Router: The smart router intelligently allocates each token in multi-GPU inference deployments, ensuring balanced load during both the preloading and decoding phases to avoid bottlenecks.
·GPU Planner: The GPU planner can automatically adjust preloading and decoding nodes, dynamically increasing or reallocating GPU resources based on intraday demand fluctuations, further achieving load balancing.
·Improved NCCL Collective for Inference: The new algorithm of the NVIDIA Collective Communications Library (NCCL) reduces small message transmission latency by four times, significantly improving inference throughput.
·NIXL (NVIDIA Inference Transfer Engine): NIXL utilizes InfiniBand GPU-Async Initialized (IBGDA) technology to directly transfer control flow and data flow from the GPU to the NIC without going through the CPU, greatly reducing latency.
·NVMe KV-Cache Offload Manager: This module allows KV Cache to be stored offline on NVMe devices, avoiding repeated calculations in multi-turn conversations, thereby accelerating responses and freeing up preloading node capacity.
Smart Router
The smart router can intelligently route each token simultaneously to preloading (prefill) and decoding GPUs in multi-GPU inference deployments. During the preloading phase, it ensures that incoming tokens are evenly distributed across the GPUs responsible for preloading, thus avoiding bottlenecks caused by traffic overload in any specific expert parameter module.
Similarly, during the decoding phase, it is crucial to ensure that sequence lengths and requests are reasonably allocated and balanced among the GPUs responsible for decoding. For those expert parameter modules with a high processing load, the GPU planner can also replicate them to further maintain load balance.
In addition, the smart router can achieve load balancing among all model replicas, which is an advantage that many inference engines like vLLM do not possess.
Source: NVIDIA
GPU Planner
The GPU planner is an automatic scaler for preloading and decoding nodes, capable of launching additional nodes based on the natural fluctuations in demand throughout the day. It can implement a certain degree of load balancing among multiple expert parameter modules based on expert models (MoE), whether during preloading or decoding phases. The GPU planner will activate additional GPUs to provide more computing power for high-load expert parameter modules and can dynamically reallocate resources between preloading and decoding nodes as needed, maximizing resource utilizationIn addition, it also supports adjusting the GPU ratio used for decoding and preloading—this is particularly important for applications like Deep Research, which require preloading a large amount of contextual information while the actual generated content is relatively small.
Source: NVIDIA
Improved NCCL Collective Communication
A new set of low-latency communication algorithms added to the Nvidia Collective Communications Library (NCCL) can reduce the latency of small message transmission by 4 times, significantly enhancing overall inference throughput.
At this year's GTC, Sylvain detailed these improvements in his speech, focusing on how single and double all-reduce algorithms achieve this effect.
Since AMD's RCCL library is essentially a copy of Nvidia's NCCL, Sylvain's reconstruction of NCCL will continue to expand CUDA's moat while forcing AMD to spend significant engineering resources synchronizing with Nvidia's major reconstruction results, allowing Nvidia to use this time to continue advancing the cutting-edge development of collective communication software stacks and algorithms.
Source: NVIDIA
NIXL — Nvidia Inference Transport Engine
To achieve data transmission between preloading nodes and decoding nodes, a low-latency, high-bandwidth communication transport library is needed. NIXL uses InfiniBand GPU-Async Initialized (IBGDA) technology.
Currently in NCCL, the control flow goes through a CPU proxy thread, while the data flow is transmitted directly to the network card without going through CPU buffering. With IBGDA, both control flow and data flow can be transmitted directly from the GPU to the network card without CPU intermediaries, significantly reducing latency.
Additionally, NIXL can abstract the complexity of transferring data between CXL, local NVMe, remote NVMe, CPU memory, remote GPU memory, and GPUs, simplifying the data movement process.
Source: NVIDIA
NVMe KVCache Offload Manager
The KVCache offload manager improves overall efficiency during the preloading phase by storing the KV cache generated in previous user conversations to NVMe devices instead of discarding them directly
Source: NVIDIA
When users engage in multi-turn conversations with large language models (LLMs), the model needs to consider the previous Q&A as input tokens. Traditionally, reasoning systems would discard the KV cache used to generate these Q&As, leading to the need for recalculation and repeating the same computational process.
However, with the adoption of NVMe KVCache offloading, when users temporarily leave, the KV cache is offloaded to NVMe storage; when users ask questions again, the system can quickly retrieve the KV cache from NVMe, eliminating the overhead of recalculation.
This not only frees up the computational capacity of preloaded nodes, allowing them to handle more input traffic, but also improves the user experience by significantly shortening the time from the start of the conversation to receiving the first token.
Source: NVIDIA
According to DeepSeek's GitHub notes on the sixth day of the open-source week, researchers disclosed that their disk KV cache hit rate is 56.3%, indicating that the typical KV cache hit rate in multi-turn conversations can reach 50%-60%, which significantly enhances the efficiency of preloaded deployments. Although recalculation may be cheaper than loading in shorter conversations, the overall cost savings brought by the NVMe storage solution are substantial.
Friends tracking DeepSeek's open-source week should be familiar with the above technology. These technologies are an excellent way to quickly understand NVIDIA Dynamo's innovative achievements, and NVIDIA will also release more documentation about Dynamo.
All these new features collectively achieve a significant acceleration in reasoning performance. NVIDIA has even discussed how performance can be further improved when Dynamo is deployed on existing H100 nodes. Essentially, Dynamo makes DeepSeek's innovative achievements accessible to the entire community, not just those with top-tier reasoning deployment engineering capabilities; all users can deploy efficient reasoning systems.
Finally, because Dynamo can broadly handle distributed reasoning and expert parallelism, it is particularly beneficial for single replication and higher interactivity deployments. Of course, to fully leverage Dynamo's capabilities, a large number of nodes must be in place to achieve significant performance improvements.
Source: NVIDIA
These technologies collectively bring about a tremendous improvement in reasoning performance. NVIDIA mentioned that significant performance improvements can also be achieved when Dynamo is deployed on existing H100 nodes. In other words, Dynamo allows the best outcomes of open-source reasoning technology to benefit all users, not just those with a strong engineering background in top AI laboratoriesThis enables more enterprises to deploy efficient inference systems, reducing overall costs and enhancing the interactivity and scalability of applications.
Decrease in Total Cost of Ownership for AI
After discussing Blackwell, Jensen Huang emphasized that these innovations have made him the "chief revenue destroyer." He pointed out that Blackwell has achieved a 68-fold performance improvement over Hopper, resulting in an 87% cost reduction. Rubin is expected to achieve a performance improvement 900 times higher than Hopper, with a cost reduction of 99.97%.
Clearly, Nvidia is relentlessly driving technological advancement—as Jensen Huang stated: "When Blackwell starts shipping at scale, you won't even be able to give away Hopper for free."
Source: NVIDIA
We emphasized the importance of deploying computing power early in the product cycle in our "AI Neocloud Action Guide" last October, which is precisely why the rental prices for H100 are expected to accelerate their decline starting in mid-2024. We have consistently called for the entire ecosystem to prioritize the deployment of next-generation systems, such as B200 and GB200 NVL72, rather than continuing to procure H100 or H200.
Our AI cloud Total Cost of Ownership (TCO) model has demonstrated to clients the leap in productivity across generations of chips and how this leap drives changes in AI Neocloud rental prices, subsequently affecting the net present value for chip owners. To date, our H100 rental price forecast model released in early 2024 has achieved an accuracy rate of 98%.
Source: AI TCO Model
Co-Packaged Optics (CPO) Technology
Source: NVIDIA
In the keynote speech, Nvidia announced its first co-packaged optics (CPO) solution, deploying it in expanded switches. Through CPO, transceivers are replaced by external laser sources (ELS), working in conjunction with optical engines (OE) placed directly next to the chip wafer to achieve data communication. Now, optical fibers are directly inserted into ports on the switch, routing signals to the optical engine without relying on traditional transceiver ports.
Source: NVIDIA
The main advantage of CPO is the significant reduction in power consumption. With the elimination of the need for digital signal processors (DSPs) on switches and the use of lower-power laser light sources, substantial power savings have been achieved. Using linear pluggable optical modules (LPO) can also achieve similar effects, but CPO allows for a higher switch base, thereby flattening the network structure—enabling the entire cluster to implement a two-layer network using CPO instead of the traditional three-layer network. This not only reduces costs but also saves power, with energy-saving effects nearly as significant as reducing transceiver power consumption.
Our analysis shows that for a 400k* GB200 NVL72 deployment, transitioning from a DSP-based three-layer network to a CPO-based two-layer network can save up to 12% in total cluster power consumption, reducing transceiver power consumption from 10% of computing resources to just 1%.
Source: Semianalysis
Nvidia today launched several CPO-based switches, including the CPO version of the Quantum X-800 3400 switch, which debuted last year at GTC 2024, featuring 144 800G ports and a total throughput of 115T, equipped with 144 MPO ports and 18 ELS. This switch is set to launch in the second half of 2025. Another Spectrum-X switch offers 512 800G ports, also suitable for high-speed, flattened network topologies, with this Ethernet CPO switch planned for release in the second half of 2026.
Source: NVIDIA
Although today's release is already groundbreaking, we believe that Nvidia is merely warming up in the CPO field. In the long run, the greatest contribution of CPO in scaled networks lies in its ability to significantly enhance the base and aggregate bandwidth of GPU expansion networks, enabling faster and flatter network topologies, opening up a scaled world far beyond 576 GPUs. We will soon publish a more detailed article exploring Nvidia's CPO solutions.
Nvidia Still Reigns, Targeting Your Computing Costs
Today, Information published an article stating that the pricing of Amazon's Trainium chips is only 25% of the H100 price. Meanwhile, Jensen Huang claimed, "When Blackwell starts shipping at scale, you won't even be able to give away H100s for free." We find this statement highly significant. Technological advancements are driving down total ownership costs, and apart from TPUs, we can see replicas of Nvidia's roadmap everywhere. Jensen Huang is pushing the boundaries of technology continuously. New architectures, rack designs, algorithm improvements, and CPO technology all set Nvidia apart from its competitorsNvidia is leading in almost every field, and when competitors catch up, they continue to break through in another direction. As Nvidia maintains its annual upgrade pace, we expect this momentum to continue. Some talk about ASICs being the future of computing, but we have already seen that platform advantages, like in the CPU era, are hard to surpass. Nvidia is rebuilding this platform through GPUs, and we anticipate they will continue to stay at the forefront.
As Jensen Huang said, "Good luck keeping up with this chief revenue disruptor."