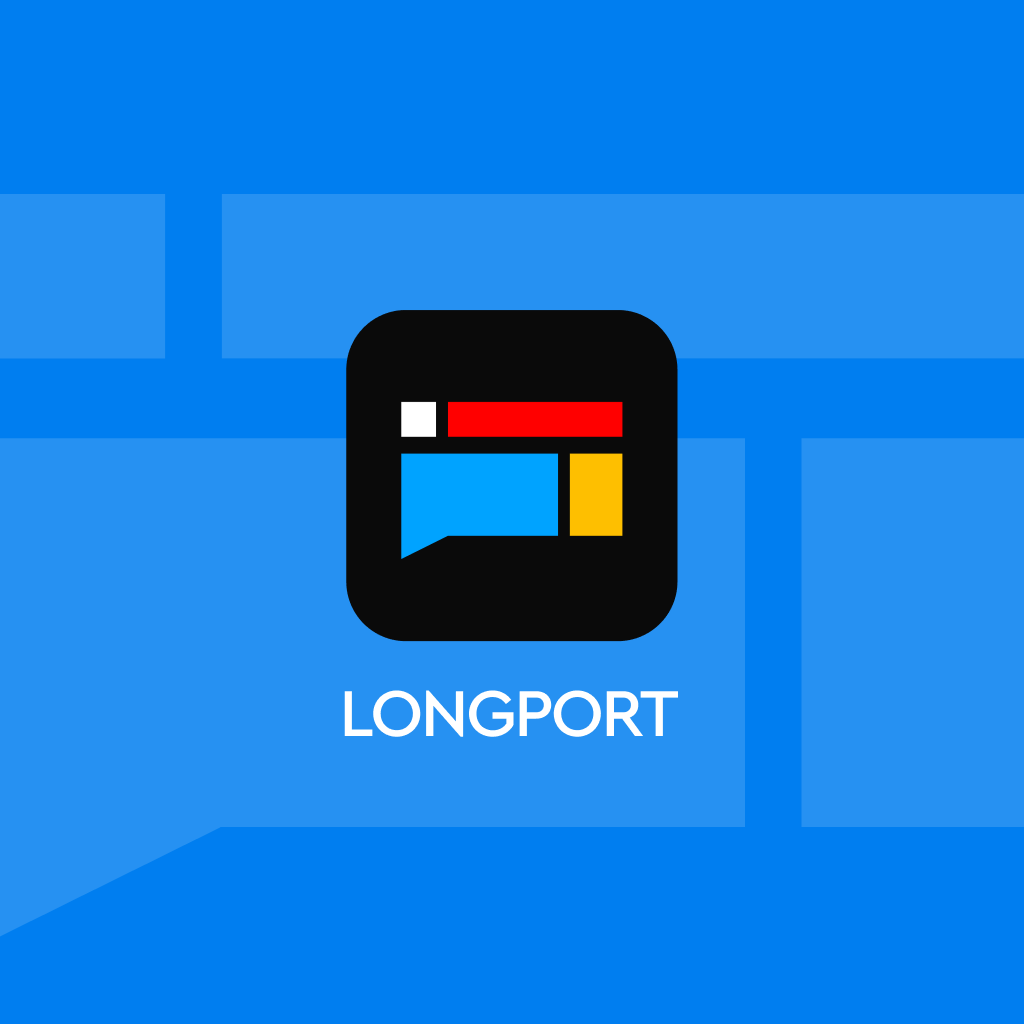
"Tech Sister" Wu Chao said: It is too early to talk about a bubble in tech stocks; the space for the artificial intelligence industry is no less than that of the internet
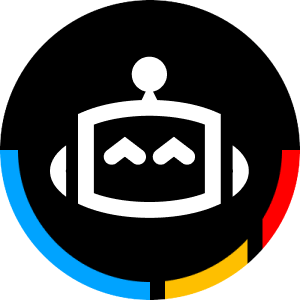
Wu Chao, the director of the research institute at CITIC Construction Investment Securities, stated that it is too early to discuss a bubble in technology stocks, as the prospects for the artificial intelligence industry are broad. She pointed out that the implementation of the AI industry chain requires time, and short-term adjustments are normal. DeepSeek's foundational model has boosted investment confidence, and competition will become more intense. It is expected that by 2025, the concentration of computing power and model layers will increase, and B-end applications will drive GDP growth, surpassing the mobile internet era. Performance reflections take time, with better results expected in the second quarter
The director of the research institute at CITIC Construction Investment Securities and chief analyst for the TMT industry, Wu Chao, is one of the most influential TMT industry analysts in the market.
Her predictions and statements regarding pan-technology, TMT, and AI have garnered significant attention from the market and institutions. Recently, she spoke at an event.
She discussed various aspects of the AI industry chain, shared her views on the recent market performance of technology stocks, and whether there is currently a bubble, while looking at promising directions from the perspective of 2025 and even longer cycles.
Core viewpoints:
-
The foundational model of DeepSeek has a very low cost while being comparable in capability to the world's top large models, which greatly enhances or restores investment confidence in the Chinese technology sector.
-
However, we must also recognize that the implementation of our AI industry will not happen overnight. It will take time for capital expenditure to reflect in the corresponding infrastructure supply chain and company performance, so short-term adjustments are very normal.
-
Investment in foundational models has not decreased, and competition among large models will become more intense, with a faster iteration speed.
-
The competition in the AI industry is more concentrated than it was in the mobile internet era ten years ago. Back then, apart from China and the U.S., Japan, South Korea, and Europe had very developed mobile gaming industries, but now the latter has almost no opportunities.
-
From the investment framework of 2025, the certainty of computing power itself is still the highest. The further we go into computing power and model layers, the more concentrated it becomes, and the fewer companies can be accommodated.
-
The final application scenarios are shifting from the consumer side to more empowering production on the business side, including the automotive industry chain and high-end manufacturing. If significant applications emerge on the business side, the impact of AI on GDP and industry will far exceed the scale of ToC during the mobile internet era.
-
The first quarter reports will not reflect performance particularly clearly; the mid-year reports, especially the second quarter, will show better results. This is because the mapping from computing power to investment and the delivery of orders requires at least a six-month cycle. Some directions that everyone is paying attention to, such as upstream storage and PCB, may reflect earlier.
Using the first-person perspective, some content has been abbreviated.
Short-term adjustments are normal
With the emergence of DeepSeek, there has been significant attention on artificial intelligence and the entire TMT sector since the Spring Festival. Whether it's the performance of the Hang Seng Tech Index in Hong Kong from the beginning of the year to now or the overall performance of the TMT technology sector in the A-share market, both have significantly outperformed the market.
The emergence of large models like DeepSeek has driven enthusiasm for investment in the entire technology sector. Of course, there have also been some fluctuations recently.
I believe we should view stock investment and the fundamentals of the industry in two layers.
First, from the perspective of the overall industry's basic progress, the foundational model of DeepSeek has a very low cost while being comparable in capability to the world's top large models, such as OpenAI's o1 and o3 models. This indeed greatly enhances or restores investment confidence in the overall market, especially in the Chinese technology sector However, we must also recognize that the implementation of the artificial intelligence industry is not an overnight process. Although we see that major companies like Alibaba and Tencent are directly driving an increase in capital expenditure in the near future.
For example, Alibaba has guided that its capital expenditure over the next three years will exceed the total of the past 10 years, corresponding to an annual capital expenditure of about 100 billion. However, it takes time for the guidance on capital expenditure to translate into the corresponding supply chain for GPUs, servers, and even data center infrastructure, or the performance of related companies. These expenditures cannot be reflected in these companies' performance overnight, so it is very normal to see adjustments in the short term.
The first wave will definitely be the recovery of confidence, as well as the recovery of investment and infrastructure spending in the conceptual model and the overall industry. First, we will see actions to lift valuations. Before the Spring Festival, data centers, including major Chinese internet companies like Alibaba and Tencent, had their valuations basically below the historical 50th percentile.
However, after the valuation has been repaired to a certain extent, people will again expect whether EPS or performance will show mapping and continuous verification. This will return to the upcoming annual report period, including data related to the first quarter report.
At this stage, the fundamentals need another follow-up process. In such a vacuum period or relatively performance verification cycle, it is very normal for the entire sector to experience adjustments in the short term.
It’s Too Early to Call It a Bubble
Any judgment about a bubble must include the dimensions of time and space. Are we looking at the bubble in a 3-month cycle, a 3-year cycle, or even a 10-year cycle?
I have always held the view: If we compare it to the last decade of mobile internet, specifically from around 2010 when 3G was commercialized to 2020, I think 2025 is very similar to 2013.
First of all, whether it is the overall macro economy, the performance of the capital market, or the stock market, it is very similar to 2012 and 2013, with overall structural changes.
For example, the first profitable application of mobile internet, mobile games, only began to emerge in 2013, and to this day, the mobile gaming industry remains the most profitable direction of mobile internet.
In addition, in terms of hardware infrastructure, before 2013, mobile internet was still in the investment phase of communication industry infrastructure. The capital expenditure of the three major telecom operators on 3G network construction was in a growth cycle during those years. Correspondingly, the performance of equipment manufacturers and terminal hardware manufacturers involved in communication equipment was good.
However, after 2013, we began to see that after the communication infrastructure was nearly built, the application industry cycle of mobile internet began to emerge. 2013 was the year of games, 2014 was the year of internet healthcare and education, and after 2015, various OTOs, including e-commerce and short videos, began to rise.
In this cycle, if we must talk about so-called bubbles, it was actually from 2014 to 2016 that we welcomed the application explosion cycle, which also brought some bubbles. Moreover, behind this is a major technological transformation cycle In the most exaggerated times of 2014 and 2015, the TTM dynamic valuation of the entire TMT industry, involving the four sectors of telecommunications, electronics, computers, and media, was basically over 100 times, with computers being particularly crazy, peaking at around 140 times valuation.
So, looking back simply from today, if we assume that the space and scale of the current artificial intelligence technology or industrial transformation is equivalent to that of the past mobile internet, I believe no one would say that the entire trend of the artificial intelligence industry is a bubble.
I think this round of transformation is at least of the same magnitude as the mobile internet transformation in 2013, and even more important, just transitioning from the dividends of telecommunications to what is now referred to as computing dividends. In the past, we mainly focused on the capital expenditures and infrastructure of operators; now we focus on the capital expenditures of internet companies and CSP cloud vendors.
This year, Chinese CSP vendors, such as Alibaba, Tencent, and ByteDance, may have a combined capital expenditure of around 300 to 400 billion RMB, which is comparable to the investment scale of operators. However, looking at the American CSP vendors M7, their capital expenditure this year is estimated to be around 300 to 400 billion USD.
So, based on this foundation, if the scale of industrial revolution and innovation is similar, it feels quite like 2013. If we really want to talk about bubbles, the dynamic valuations of over 100 times in the media industry or computer sector are still far from it.
Now, tracking the industry, I think the application is inevitable. Every day, people open applications related to AI, paying attention to usage time and activity, just like tracking the traffic changes of gaming or e-commerce C-end applications back then, which can also track the rhythm of application landing. As long as applications can step by step, for example, releasing a hit product every three months, the valuation of the application sector can be maintained.
The current level of congestion is indeed high, but this is not a steady state; the entire industry is still in a very fast-changing cycle of transformation. Therefore, compared to the wave in 2013, I think it is still difficult to call it a bubble.
Large Models as Engines
Indeed, there has been a lot of discussion about DeepSeek in the short term, with various articles interpreting it, but from the perspective of industry research, I think we still need to view this matter objectively.
First of all, looking at it comprehensively, the dominance of the artificial intelligence industry is between China and the United States. This is even more concentrated than the mobile internet era ten years ago. Back then, apart from China and the U.S., Japan, South Korea, and Europe had very developed mobile gaming industries. The most famous games on the iPad at that time, such as Plants vs. Zombies and Angry Birds, were all developed by European game companies.
The large models that DeepSeek is working on are at the core of innovation. If we think of large models as an engine or a core production tool, they are actually the main tools in this wave of intelligent innovation.
I believe that DeepSeek's main contribution, or its contribution to restoring confidence in Chinese technology, has two aspects.
On one hand, in terms of model capability, DeepSeek has aligned its capabilities with the world's top models, such as OpenAI's o1 model, Llama3, and others This is very important.
In fact, large models emerged at the end of 2022, and over the past two years, China's models have lagged behind by about 6 to 12 months. The emergence of DeepSeek has at least shortened this time frame to less than half a year, and the overall pace of catching up will not fall too far behind, unlike when others were already at 3G while we were still at 2G.
The capabilities of basic tools are not aligned; discussing the so-called mobile internet is essentially building castles in the air.
Secondly, the applicability or cost of the models is also very important. DeepSeek not only aligns with top model capabilities but, more importantly, reduces costs. One is the cost of model training itself, and the other is the cost of obtaining each token response during Q&A, both of which have seen significant reductions.
Of course, this reduction is based on engineering innovations. Innovations in engineering are also innovations. It is also important to replicate the same things or capabilities.
Currently, our underlying computing power for so-called inference models is limited, but we have achieved replication with relatively limited resources (hardware computing cards) and at a lower cost. This is another significant contribution.
Before the Spring Festival, large models were still in the research and development stage, and it was said that they were heard about more than used. However, after the Spring Festival, this contribution directly triggered large models to enter the production phase.
The alignment of capabilities and the reduction of costs are very important contributions of DeepSeek.
Still Far from the Endgame
I think we are still far from a clear endgame.
If we compare it to the recent new energy vehicle sector, after four or five years, it is still difficult to determine which automaker will emerge victorious. Looking further back at mobile phones, from the initial dozens of manufacturers to the later "China Cool Union," and then to the later HOV, it actually went through a long 10-year cycle before only these three remained.
I think large models are the same; it is hard to say that the current situation is the endgame. In the short term, within one or two months, many models have aligned with and absorbed DeepSeek's capabilities because it is an open-source model.
Of course, we have seen another approach in the United States, where their investment in foundational models has not decreased; they will not reduce their investment in basic pre-training due to the emergence of DeepSeek.
The rule that the larger the model, the better the effect still exists. Whether it is large companies in the United States or large companies in China, they are actually increasing capital expenditures. The competition for large models will become more intense, and the speed of iteration will be faster. This is one aspect of the impact.
On the other hand, for small and medium developers, such as those creating applications or specialized models, they can indeed apply and land their projects more quickly, starting to reduce costs and increase efficiency in the industry, yielding real financial returns and fostering healthy competition throughout the industry.
To summarize, judging "the East rises while the West falls" just because of the emergence of DeepSeek is definitely premature. However, from the perspective of the entire industry, it has narrowed the short-term gap between us and the United States. Looking further ahead, competition will still be very fierce and will depend on the speed of iteration of the model's capabilities in applications and the underlying computing power Of course, we also have strengths, such as data. Our large population base, combined with a sufficient number of digital scenarios in industrial verticals, gives us an advantage in data quality. How to combine our weaknesses and strengths with the entire industry is also a question.
I believe the most important contribution of DeepSeek, to put it in one sentence, is that it has activated the investment enthusiasm of the entire AI industry. Fundamentally, the influx of underlying capital is also very important. In the entire module, whether it's card production or large model creation, it requires a lot of money. If the underlying investors are unwilling to invest in this sector, whether in the primary or secondary market, it creates the greatest pressure. However, DeepSeek has activated this situation, which is very important for the industry itself.
The Highest Certainty of Computing Power
The general framework for investing in AI consists of three main parts: the upstream algorithm block, the midstream model and data module, and the application module. For ordinary investors, dividing it into these three parts is relatively simple and easy to understand. Overall, under the framework of 2025, the certainty of computing power itself is still the highest.
Although after the emergence of DeepSeek, various discussions suggest that the final training cost of DeepSeek is only about 6 million USD, does this mean that the cost of computing power has decreased, and perhaps the logic of "great effort leads to miracles" will no longer apply? Does this mean that there will be greater investment pressure on underlying companies like NVIDIA?
We divide our demand for computing power into two main parts: one part is model training, and the other part is applications, which are starting to move towards areas like AI healthcare, AI finance, and education.
After the Spring Festival, the global demand for basic training models is still rapidly increasing. If we understand DeepSeek's innovation as an engineering innovation, it has shown good performance in reasoning models, but the foundational model remains the basis for the capabilities of reasoning models.
DeepSeek's R1 model is also based on the previous R1-zero and V3 models, enhanced through reinforcement learning.
Therefore, if this moment is not the endgame, we need to continue leading large models, which are the core of the entire industry, whether open-source or closed-source. The original foundational models, or the pre-training sector, are still the root of global innovation.
Of course, there is a problem of diminishing marginal returns in this process. For example, GPT-3 has a dataset scale of hundreds of billions, while GPT-4's dataset scale has increased to 1.7-1.8 trillion, and the latest may have reached 10 trillion, potentially moving towards hundreds of trillions in the future. When the scale of the model increases by an order of magnitude, the model's capability may only increase by about 10% or 20%. But for large companies, even if the cost-performance ratio is relatively low, they will still continue to pursue this, which is our basic judgment.
After the release of the Grok3 model promoted by Musk, there was also discussion that the number of cards doubled, but the intelligence level only improved by 10-20%. Large companies will still continue this, which determines that the demand for the basic cards used for model training, whether single cards or clusters, will not decrease Second, the demand for inference will definitely increase significantly, and this judgment is also correct. Compared to the past two years, where everyone mainly focused on training large foundational models, primarily using NVIDIA's cards, NVIDIA's ecosystem, including NVlink, has determined that it is the best for training. However, looking ahead, the demand for inference will certainly rise sharply.
On one hand, the technical routes for inference models, such as o1 and DeepSeek, are more effective and more popular, which will increase the demand for inference cards.
On the other hand, the rise of applications will also continue to increase the demand for inference. There are more and more application scenarios, and this incremental demand is not a problem, but we may need to assess whether there will be changes in supply.
In the past, only NVIDIA's cards could be used, but in the future, domestic and customized chips from overseas major manufacturers may also be put into use. Similar situations have occurred in the CPU era, and in the end, supply will definitely become more abundant. This is why I believe companies like NVIDIA will face pressure in the short term.
Overall, whether it is the demand for training or inference, in the short term, due to the explosion of technology and the rise of applications, the most certain factor is still the computing power itself.
Returning to the investment in computing power, the computing power we commonly understand is GPU, the core AI chip. However, this year, Alibaba and overseas major manufacturers' capital expenditures, amounting to around 300-400 billion RMB or 30-40 billion USD, have seen only about half used to buy GPUs, while the other half is used to turn GPUs into servers. This requires storage, connectivity, optical modules for optical-electrical conversion, and even higher requirements for high-speed transmission on the underlying PCB and copper-clad boards. In the past, data centers, referred to as IDC, required air conditioning, cooling, and liquid cooling.
Therefore, I believe computing power itself is a large sector, not just the most expensive (GPU); this is a concentrated investment position. If one believes that the pressure to purchase this part is relatively high, there are actually hundreds of companies in the entire computing power sector, providing many good investment opportunities.
Domestic computing power is also a direction we are optimistic about this year. If we look upstream at domestic production, corresponding to advanced process foundries, and further upstream to semiconductor equipment and materials, their certainty is also very high. In terms of computing power, the further upstream you go, the more concentrated it becomes, but it is also relatively easier to select companies and targets.
To summarize, Alpha, or in the short term, may not necessarily have Alpha, but the highest certainty will still be in the computing power sector. Because it will genuinely have performance, we can see the increase in capital expenditure, ultimately landing on orders and fundamentals; it is just a matter of time.
“Alpha” in Domestic Computing Power
Domestic computing power is the Alpha of the entire global computing power sector this year.
One reason is that major Chinese companies have only started to have significant capital expenditures this year, amounting to around 300-400 billion RMB, and we can see how much Alibaba, ByteDance, and Tencent account for This year, there will be something very interesting: the spending of Chinese internet companies and the capital expenditure of operators will be comparable for the first time, which is actually hard to imagine in history.
In the past, the capital expenditure of the three major operators dominated. During the last round of cloud computing, even at the peak in 2015 and 2016, the capital expenditure of operators was at least 4:1 compared to the internet; if the operators spent 400 billion, the internet would be 100 billion. Now, both sides are at the level of three to four hundred billion. I believe that China's current scale is very similar to that of the United States two years ago, so there is a time lag. Of course, we need to further study which companies are mainly in the supply chain of China's internet giants.
To elaborate a bit more, there were many concerns about domestic computing power in the past two years, such as to what extent the U.S. sanctions would go—whether it would be a complete ban or sanctions on certain stages. Some parts of this will determine the entire ecosystem in China, such as whether internet giants are willing to place orders for domestic computing power, which requires a lot of adaptation and integration with the entire ecosystem.
At this moment, this uncertainty will also become clearer.
Most importantly, everyone is concerned about domestic production capacity. If domestic production cannot deliver cards on a large scale, it would be a castle in the air; we cannot rely entirely on other means to achieve this. Overall, after years of effort, domestic advanced processes and related production capacity will gradually be released well from 2025 and 2026 onwards. This will truly mean moving from "0 to 1" to "1 to n." Being able to release production capacity is also very important.
Combining these three aspects, this year domestic production will be better overall. However, within the domestic industrial chain, we need to differentiate between GPU, CPU, servers, network equipment, optical communication, IDC data centers, and even PCB and storage, which are large modules. There are many directions to explore within this. Moreover, the entire sector is currently experiencing significant volatility; as long as we can see the industrial trends clearly, high prices are not an issue, and they will also become cheaper. Due to the impact of certain events, including the vacuum period of financial reports, some targets have experienced significant fluctuations. In this process, the key is still how to grasp the underlying trends of the industry and find good trading opportunities.
Application space mainly focuses on two aspects
Beyond computing power, the major area of focus is definitely applications.
In the mobile internet era, we have a 1:7 rule: for every 1 yuan of capital expenditure at the bottom level, there is at least a 7 yuan return in applications, driving GDP. If we calculate the total capital expenditure of the three major operators back then and correspond it to the broad value of the internet, we can derive this rule. For an industry to be called an industry, it must be closed-loop.
Last year, some people also did similar calculations, stating that for every 1 yuan of basic computing power invested, the output might only be 0.7 yuan, which certainly cannot sustain a stable cycle.
I believe that as we move forward, the entire application, in the broad category of artificial intelligence applications, will definitely be a pyramid structure. The closer we get to the computing power and model layer, the more concentrated it becomes, and the fewer companies it can accommodate; however, the closer we get to applications, the more companies it can accommodate, the more scenarios there are, and the larger the industry becomes. ** This is a basic logic.
However, from an investment perspective, the most challenging aspect of applying it now is the difficulty in judging the endgame. For example, looking back from 2015, many gaming companies had increased tenfold in 2013, but 90% of them were unsuccessful, and only 10% made it through. In 2013, it was impossible to determine who would ultimately succeed. During this cycle, investing in indices or sectors is a relatively better solution, although some individual stocks may outperform in stages. After 2015, it was found that the most successful companies in the gaming sector were Tencent and NetEase, and then buying Hong Kong stocks became a strategy.
Therefore, the biggest difficulty in making applications now is the inability to judge the endgame. For instance, recently popular fields like future healthcare, robotics, and autonomous driving are hard to predict who will emerge successfully. This is a challenge in investment.
But if we must make a judgment, our team is optimistic about several application directions.
First, Agent Intelligence. People are considering whether it's AI advertising or companies like those in the US stock market that do SaaS and ToB software, or perhaps companies that own data could be the direction. However, I believe that overall, the capability of intelligence itself is an application in this wave of change.
For example, recently the most frequently opened applications are still Doubao or Tencent Yuanbao; these capabilities still require a carrier. This carrier doesn't necessarily have to be a brand new app; it could still be something existing, where knowledge is attached to intelligent capabilities, allowing users to process data more efficiently, etc.
Thus, in applications, intelligent agents are a very important direction this year. Looking at it now, hardware companies developing intelligent agents have great potential, such as Apple, Xiaomi, and many mobile phone companies, etc. Mobile phones naturally have data entry points and can integrate various apps within. The same goes for PCs. In other words, hardware is a sector that is hard to bypass, and there will be companies within hardware that do well in developing intelligent agents. Therefore, as a fallback, while you may not be able to buy Apple, you can invest in Apple's supply chain, which can at least drive the entire industry's innovation cycle. Extending from the intelligent agent direction, AI terminals including mobile phones and PCs, and even companies making glasses, are definitely a direction.
Of course, some large model companies, due to capital expenditure and high requirements for cards, cannot continue to compete in foundational models; they will also turn to the development of intelligent agents. Recently, some startups that have performed well with large models have begun to pivot towards building intelligent agents for enterprises or creating third-party intelligent agents, which is also a trend. Additionally, some A-share software companies have advantages in specific vertical data, such as in finance, education, and healthcare scenarios. Once the intelligent agents are built, combined with private domain data, there are opportunities.
Second, looking long-term, autonomous driving is definitely a very important application scenario, which can be viewed as an application scenario of AI or an extension of automobiles. Various signs indicate that the enhancement of large model capabilities, especially with the emergence of reasoning models, significantly improves usability in serious scenarios like autonomous driving and road applications In this way, autonomous driving is not probability theory, but rather a clear logical reasoning. This year, companies like BYD have also launched intelligent driving.
The benefit of driverless technology is that, although it is not very mature now, its market is large enough. For huge investments, either the implementation must be quick, or the long-term potential must be significant. When researching tech stocks, the most important thing is to see a large long-term potential. If a market was originally 10 billion and now becomes 12 billion, the market's expectation gap will quickly narrow. However, if the original potential was 20 billion and it can grow to 200 billion in the future, then people will be willing to wait, and risk appetite will increase.
To extend this further, the recently popular robots follow the same reasoning. Cars are essentially robots; this is actually a framework. In terms of applications, I mainly focus on soft intelligent agents, hard driverless technology, and embodied intelligent robots.
Risk Warning and Disclaimer
The market has risks, and investments should be made cautiously. This article does not constitute personal investment advice and does not take into account the specific investment objectives, financial situation, or needs of individual users. Users should consider whether any opinions, views, or conclusions in this article are suitable for their specific circumstances. Investing based on this is at one's own risk