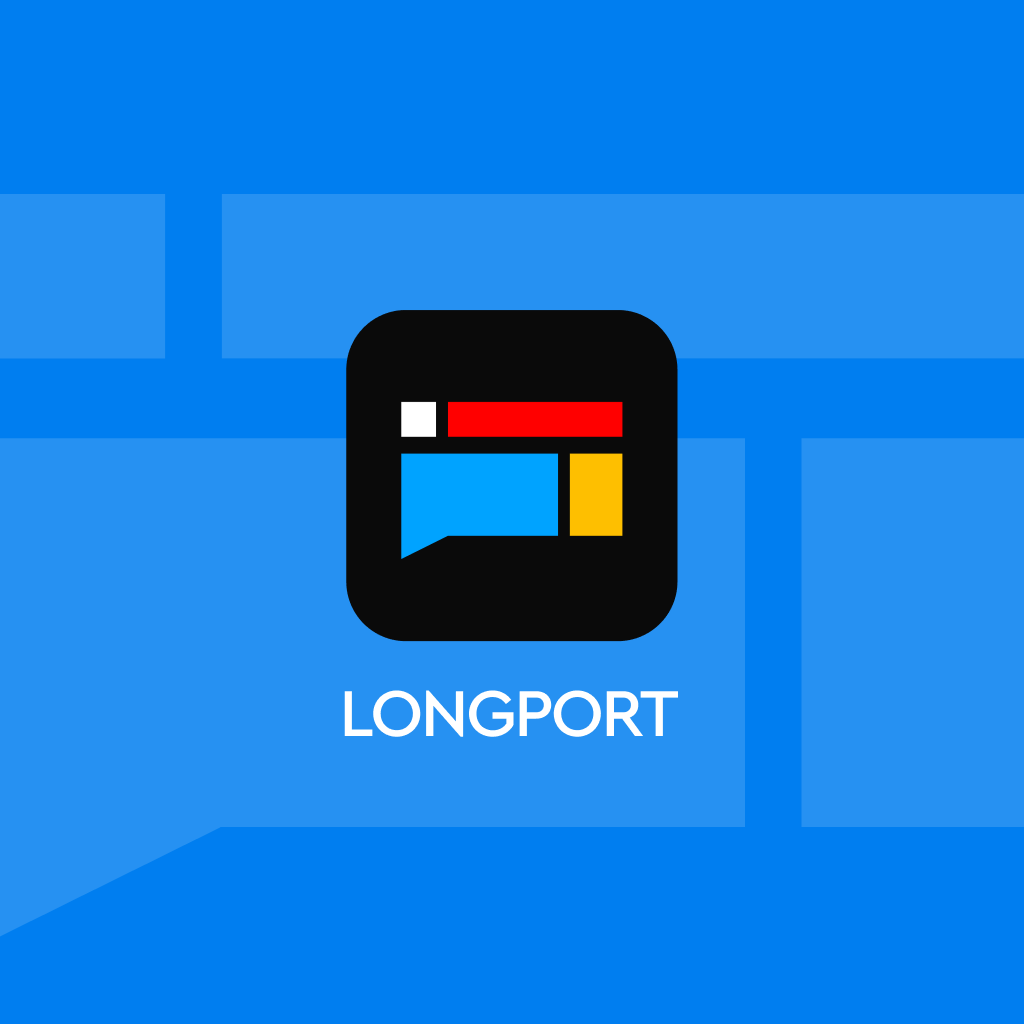
From Manus to MCP: Three New Trends in AI Over 25 Years
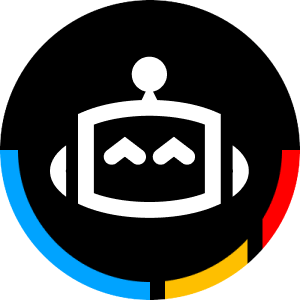
This article summarizes three major new trends in AI development over the past 25 years, with a focus on Manus's innovations and shortcomings. Based on the "virtual machine + multi-agent collaboration" model, Manus has broken through the limitations of traditional AI assistants, achieving a closed loop from demand input to outcome delivery. Although its design philosophy of "Less Structure, More Intelligence" lowers the user threshold, there is still the issue of "hallucination accumulation," which affects the accuracy of multiple Q&A sessions
Since the beginning of 2025, AI development has been in full swing, with important innovations such as DeepSeek R1, OpenAI CUA, and Manus emerging one after another, dazzling us.
Here, I will summarize my thoughts from the past month and make a few predictions about the trends in AI development in 2025.
(1) Manus: A Head Start in the Year of the Agent
After the launch of Manus, we quickly obtained an experience account and conducted thorough testing and evaluation.
To start with the conclusion: although Manus still has various shortcomings, its product design concept is full of creativity and deserves our full recognition.
The core architecture of Manus is based on the "virtual machine + multi-agent collaboration" model, dynamically allocating tasks and calling models by integrating APIs from multiple underlying large models (such as GPT-4, Claude 3, etc.).
Manus breaks the limitations of traditional AI assistants that only generate suggestions, achieving an end-to-end closed loop from "demand input" to "outcome delivery."
Manus proposes the interaction concept of "Less Structure, More Intelligence," lowering the user entry barrier through a no-code natural language interface.
At the same time, Manus uses an external markdown file to manage the task planning of agents and stores periodic work results as independent files, which is also a very interesting innovation.
(2) Shortcomings and Deficiencies of Manus
Manus provides a very interesting idea on the path of MultiAgent, but there are still some obvious shortcomings.
First is the issue of "illusion accumulation."
The essence of an agent is the series and parallel connection of multiple large model Q&A sessions. If the accuracy rate of a single large model Q&A is 90%, then after connecting 10 times, the probability of the agent answering correctly is 0.9^10, which is only about 1/3 In the following case, Manus's task is to conduct financial data analysis for a publicly listed company. Manus cleverly imported the data_api module, preparing to retrieve financial data from the interface provided by Yahoo.
However, in the process_financial_data function, Manus unexpectedly "hard-coded" data such as revenue and gross_profit directly into the code, which was quite surprising. Moreover, upon verification, some of the data here was incorrect.
If the original data is wrong, then no matter how in-depth the analysis is or how fancy the charts are, it loses its significance.
Manus's second problem is the lack of tools available for large models to call.
In the following example, Manus's task is to write a market analysis report PPT on "Xiaomi Su7."
Manus perfectly broke down the task and retrieved a large amount of news, but in the end, it could not generate a PPT because it could not call Office software.
Currently, the content output by Manus is mostly in plain text or web pages, and it cannot perfectly integrate with human workflows.
The third challenge Manus encountered is the internet ecology of high walls.
There is a lot of high-quality information on the internet that is stored within "fences."
For example, when we asked Manus to analyze and compare the cost-effectiveness of all AI smart glasses on the market, it cleverly found the corresponding product page on Taobao.
However, when Manus tried to open the specific product page to obtain detailed information such as price and performance, Taobao identified it as a robot and denied Manus's access.
Coincidentally, when we asked Manus to produce a business analysis report for a non-listed company, Manus accessed the CrunchBase database to obtain the company's latest financing progress.
However, Manus's access was identified as a robot by CrunchBase and was subsequently ruthlessly denied.
The internet seems open and transparent, but in reality, there are many situations similar to high walls, where high-quality information is often stored behind these walls, making it impossible for Manus to access directly, which undoubtedly hinders Manus's work effectiveness
Despite various problems and challenges, Manus still painted a huge prospect for MultiAgent, firing the first shot of the Agent era, which deserves our full recognition.
While Manus occupies everyone's attention, what technical reserves have overseas AI giants made?
(3) OpenAI CUA: An Agent that Can Operate Computers Independently
At the end of January this year, OpenAI released the AI entity Operator driven by its new model CUA (Computer-Using Agent).
The CUA model integrates the visual capabilities of GPT-4o and advanced reasoning abilities achieved through reinforcement learning, allowing it to break down tasks into multi-step plans and make adjustments and corrections when faced with challenges.
In short, CUA is an Agent that can operate a computer, and its operating principle is very straightforward and simple, as shown in the figure below.
First, CUA accepts two types of input simultaneously: one is text instructions, and the other is screenshots.
CUA processes both types of information at the same time and generates a series of action instructions, such as "click on the point at coordinates (300,200) on the screen, input XXX, and press enter."
After the computer receives the instructions and completes the operation, it returns the new screenshot and new task instructions to CUA, and this cycle continues until the final answer is obtained.
So, what level of computer operation capability does CUA currently have?
According to OpenAI's official evaluation, CUA has made significant performance improvements in operating computers and browsers compared to the previous generation SOTA.
However, there is still a considerable gap compared to humans. In other words, the top Agents still cannot operate a computer correctly like an adult, but I believe this situation will undergo a qualitative change within this year.
(4) Anthropic MCP: The TCP/IP Protocol of the AI Era
When analyzing the shortcomings of Manus earlier, the issue of "insufficient tools" was mentioned.
Anthropic has clearly recognized this problem and launched MCP at the end of last year to address it at its root MCP stands for Model Context Protocol, which defines the way context information is exchanged between applications and AI models, enabling developers to connect various data sources, tools, and functionalities to AI models in a consistent manner.
MCP for AI is somewhat analogous to TCP/IP for the internet.
MCP has three important characteristics:
An increasing number of tools and services are beginning to connect to MCP, showing a growing trend, including Google Maps, PGSQL, ClickHouse (OLAP database), Atlassian, Stripe, and so on.
On the Smithery platform, you can easily find tools and services corresponding to different functionalities. As more servers connect to the MCP protocol, the number of tools that AI can directly call will grow exponentially in the future, fundamentally breaking the ceiling of Agent capabilities.
(5) New Trends in AI Development by 2025: Post-Training, RL, Multi-Agent
Here, I summarize a few important trends in AI development for 2025 based on observations and thoughts from recent months.
First, pre-training is coming to an end, and post-training is becoming the focus.
This has actually become an industry consensus. At the end of last year, Ilya mentioned an important point at the NeurIPS conference: data is the fossil fuel of the AI era because we humans only have one internet.
Meanwhile, this year’s DeepSeek R1 paper mentioned that post-training will become an important component of the large model training pipeline.
Second, for post-training, reinforcement learning will become mainstream, while the importance of supervised learning is gradually declining. The most important insight brought by DeepSeek R1 is that pure RL may be the correct path to AGI.
With the increase in TTS, large models will self-emerge complex reasoning behaviors without deliberate guidance.
As shown in the right image below, the horizontal axis represents the iteration steps of large model RL, while the vertical axis represents the token length of a single Q&A. We can see that as the steps of large model RL increase, the large model autonomously transitions from "fast thinking" to "slow thinking," from initially answering 100 tokens each time to finally answering nearly 10,000 tokens each time.
The DeepSeek team refers to this phenomenon as "self-evolution" and believes it is "the emergence of sophisticated behaviors."
What specific complex behaviors are emerging? DeepSeek also provided answers, such as: self-verification, reflection, etc.
This discovery has significant implications for us. What role should supervised learning play in AI training in the future? Does supervised learning actually limit AI's problem-solving capabilities?
Shouldn't we allow AI to gain intelligence by imitating human thinking, but rather let AI develop a more native intelligence?
These questions await answers from the entire AI industry through practice.
Third, MultiAgent is a definitive trend.
If we compare AI to the human brain, large models are like the "prefrontal cortex" in the brain.
As we know, the prefrontal cortex is primarily responsible for high-level cognitive functions, such as attention allocation, reasoning, decision-making, etc.
However, having only the prefrontal cortex is insufficient for the brain to handle complex tasks. We need the temporal lobe to analyze auditory signals, the parietal lobe for reading and arithmetic, the cerebellum for motor coordination, and the hippocampus for memory indexing.
The definition of MultiAgent is precisely to coordinate between multiple different models, transitioning from a single "prefrontal cortex" to a "complete brain," thereby handling more complex real-world tasks.
In this blueprint, MCP plays a very important role: coordinating and unifying the data communication interface between large models and various tools.
(6) Conclusion: Hold on tight, the future is here!
2025 is the inaugural year of AI Agents, and the emergence of Manus has fired the first shot Whether it's OpenAI's CUA or Anthropic's MCP, both point to a common future where the pace of AI development will be very steep in the next two years.
Hold on tight, the future is here!
Author of this article: Fei Binjie, Source: Alpha Engineer, Original Title: "【Deep Dive】From Manus to MCP: Three New Trends in AI Over 25 Years"
Risk Warning and Disclaimer
The market has risks, and investment requires caution. This article does not constitute personal investment advice and does not take into account the specific investment goals, financial situation, or needs of individual users. Users should consider whether any opinions, views, or conclusions in this article are suitable for their specific circumstances. Investing based on this is at your own risk