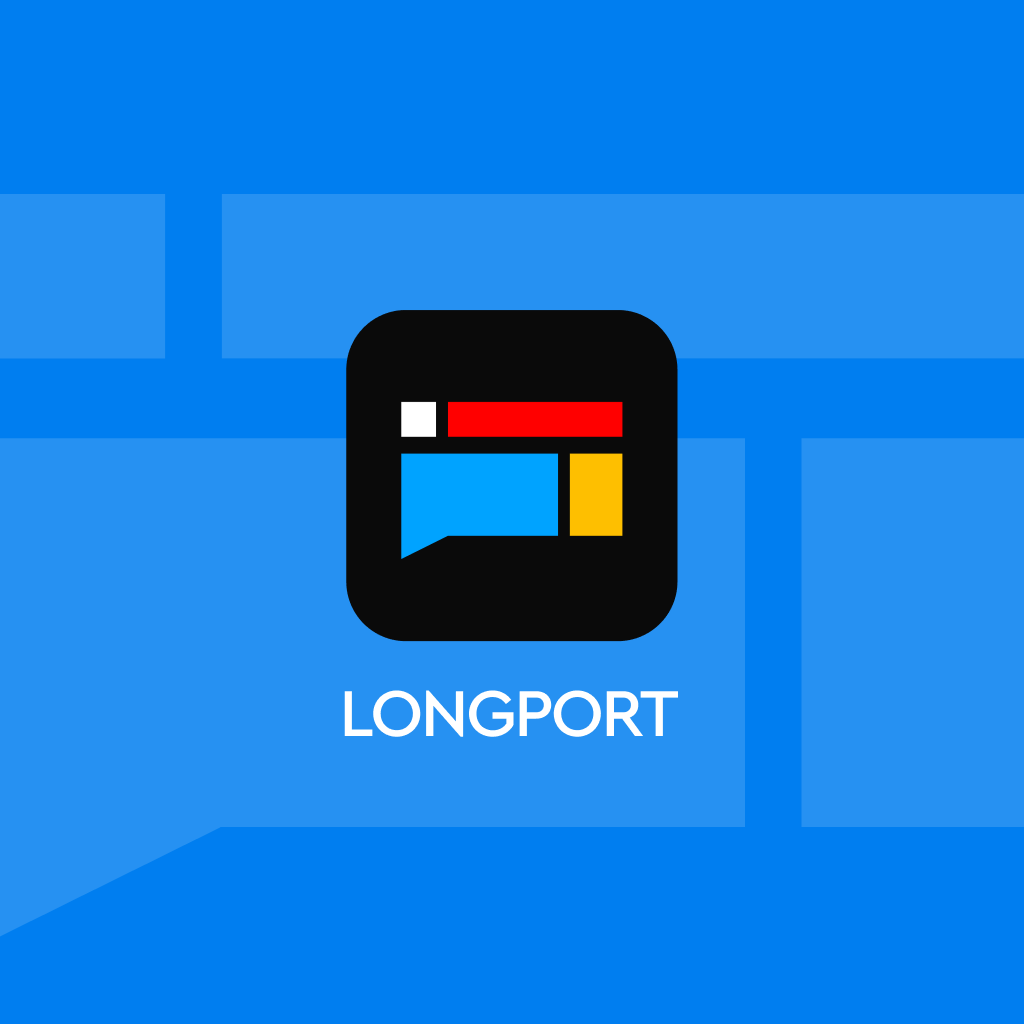
What does the shift of AI towards "reasoning models and the era of agents" mean for AI trading?
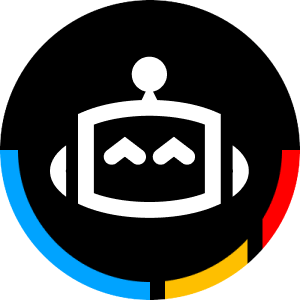
If the Scaling Law continues to be effective, we remain optimistic about AI system component suppliers (such as chips, network equipment, etc.), while being cautious towards those tech giants that have to continue investing massive capital expenditures. If pre-training scaling stagnates: we are optimistic about tech giants (as free cash flow will rebound) and focus on application stocks that have a large user base and can benefit from the decline in inference costs
Still clinging to the belief that "bigger is better" for AI models? Barclays, a Wall Street investment bank, has provided a groundbreaking prediction in its latest research report: The AI industry is undergoing a "Big Shift," where "reasoning models" and "Agents" will become the trendsetters of the new era, while the traditional large models that rely on brute force may soon become outdated!
At the core of this transformation is the evolution of AI models from "rote memorization" to "analogical reasoning." In the past, we pursued larger models, more parameters, and massive training data, firmly believing that "quantitative changes lead to qualitative changes." However, Barclays points out that this path may have reached its end.
The endless demand for computing power, high costs, and difficult-to-match returns... The "arms race" of traditional large models has left many tech giants struggling. More critically, do users really need such "large" models? In many scenarios, a smaller model that is "smarter" and better at reasoning can provide more accurate and efficient services.
What does this mean? What does this AI revolution signify for investors?
The Era of "Reasoning" Has Arrived, Small Models Can Achieve Big Things!
Barclays points out in the report that the AI industry is undergoing a series of significant transformations:
- Transitioning from traditional large language models (LLMs) to new reasoning models;
- Moving from chatbots to Agents;
- Shifting from pre-training to test-time compute;
- Changing from general-purpose training chips to custom reasoning chips;
- Evolving from centralized massive clusters to smaller, edge-computing clusters.
In simple terms, AI models are no longer solely focused on being "big," but are placing greater emphasis on being "smart." By incorporating "reasoning" capabilities, models can engage in multi-step thinking and iterative validation when processing tasks, leading to more accurate results.
This "Chain-of-Thought" (CoT) mode makes AI models more like "experts" rather than "bookworms." More importantly, it achieves a better match between cost and benefit: the more complex the task, the more computing power is needed, and vice versa.
Barclays notes that models like OpenAI's o1 and o3, as well as DeepSeek's R1 model, have already demonstrated the powerful potential of reasoning models in practice. They not only surpassed traditional large models in multiple benchmark tests but also had lower training costs and higher reasoning efficiency.
The Rise of Agents: A New Paradigm for AI Applications
In addition to reasoning models, Barclays also emphasizes the importance of Agents in the application layer of AI. Agents can be understood as a more intelligent and autonomous AI application that can not only answer questions but also execute tasks and complete transactions.
If chatbots are still in the "conversation" phase, then Agents have already begun to "act." They can be applied in various scenarios, such as customer service, financial analysis, software development, etc., greatly enhancing productivity and efficiency.
Barclays predicts that as reasoning models and Agent technology mature, AI applications will experience explosive growth. The fact that ChatGPT's user base increased by 100 million in just two months is the best proof of this
Different "Money" Prospects: Chinchilla Scaling Law May Come to an End
This AI transformation has had a profound impact on the "money" prospects of the entire industry. Barclays has proposed two possible scenarios:
- Chinchilla Scaling Law Continues to Hold: The model size continues to expand, with an increasing number of parameters and training data. By 2028, the most advanced models may reach 65 trillion parameters and be trained on 325 trillion tokens.
- Pre-training Scaling Stalls: The model size no longer grows beyond the GPT-5 level, and the industry shifts towards inference models and computation during testing.
In the second scenario, Barclays predicts that by 2028, the total computational demand of the AI industry will still reach USD 1 trillion, but far lower than the situation where the Chinchilla Scaling Law continues to hold.
More importantly, inference costs will drop significantly. This means that tech giants will no longer need to "burn money" to train ultra-large models but can allocate more resources to optimizing and applying inference models.
Computational Gap Remains Huge, Demand for Inference Chips Soars
Although pre-training scaling may stall, Barclays believes that the demand for computational power in the AI industry remains enormous. Even in the "stall" scenario, by 2028, the world will still face a gap of 250 billion exaFLOPS in inference computing power.
This means that the demand for inference chips will continue to grow. Barclays expects that by 2028, inference computing will account for more than 50% of the entire AI industry.
Rise of Open Source AI, but Hard to Shake the Status Quo in the Short Term
Barclays has also noted the rapid development of open source AI. Open source models like Llama and DeepSeek are attracting more and more developers and users.
However, Barclays believes that open source AI will not have a significant impact on the entire industry in the short term. The reason is that the cost of fine-tuning or streamlining open source models is relatively low, far less than training a large model like GPT-4.5 or Claude 4.
AI Investment Logic Changes Dramatically: Betting on "Efficiency" Rather than "Scale"
So, what does this AI transformation mean for investors? Barclays provides clear advice:
- If Chinchilla Scaling Law continues to hold: Continue to favor AI system component suppliers (such as chips, networking equipment, etc.), and be cautious about tech giants that must continue to invest huge capital expenditures.
- If pre-training scaling stalls: Favor tech giants (as free cash flow will rebound) and pay attention to application stocks that have a large user base and can benefit from the drop in inference costs.
In summary, regardless of where the AI industry is headed, investors should focus more on "efficiency" rather than "scale." Companies that can provide AI services at lower costs and higher efficiency will have an advantage in future competition