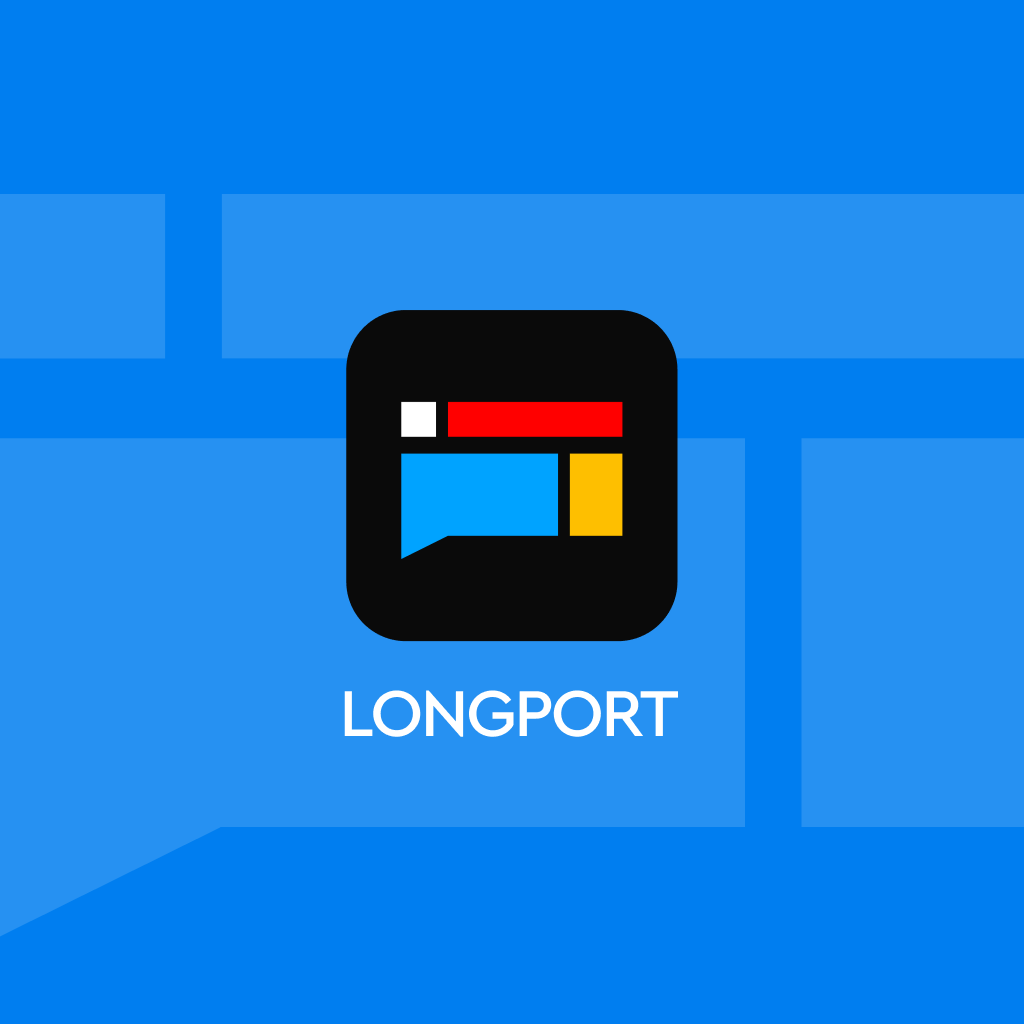
NVIDIA conference call full record: Jensen Huang stated, "The demand is extraordinary, and DeepSeek R1 has ignited global enthusiasm."
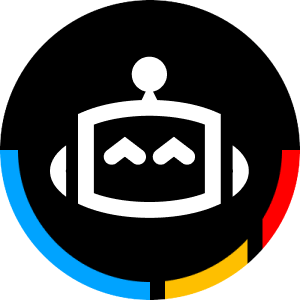
Jensen Huang stated that reasoning AI may require 100 times more computational power than one-time reasoning. Blackwell is designed for reasoning AI and can increase the throughput of reasoning AI models by up to 25 times while reducing costs by 20 times. Jensen Huang also mentioned that DeepSeek-R1 has sparked global enthusiasm; it is an outstanding innovation, but more importantly, it has open-sourced a world-class reasoning AI model
On Wednesday after the U.S. stock market closed, NVIDIA CEO Jensen Huang expressed excitement about the potential demand brought by AI inference during the Q4 fiscal year 2025 earnings call. This type of computational demand is expected to far exceed the current large language models (LLM) and may require millions of times more than existing computing capabilities.
Jensen Huang stated that what they are doing is not easy, but NVIDIA has performed well in enhancing supply capacity. AI software will be a part of all data centers, with positive signs continuing in the short, medium, and long term. He further confirmed that the supply chain issues for the Blackwell series chips have been completely resolved, and supply issues have not hindered the next training and subsequent product development. Additionally, the Blackwell Ultra is scheduled to be released in the second half of 2025.
Regarding DeepSeek, Jensen Huang mentioned that inference models like DeepSeek-R1, which apply inference time extension, can consume 100 times more computing power, and future inference models may consume even more computing power. Jensen Huang also stated that DeepSeek-R1 has sparked global enthusiasm, representing an outstanding innovation. More importantly, it has open-sourced a world-class inference AI model.
Jensen Huang also pointed out that everyone is at the starting point of a new technological transformation, and all software and services will be AI-related.
NVIDIA CFO Colette Kress noted that demand for gaming hardware remains strong during Thanksgiving and Christmas, but overall revenue is still affected by supply constraints. However, she expects supply issues to ease, leading to a "surge" in growth in the current quarter.
Regarding profitability, the CFO indicated that once Blackwell increases production, profits will improve, and she expects that by the end of 2025, profit margins will be in the mid-range of 70%-80%. However, she emphasized that the current priority is to deliver as many products as possible to customers. The growth rates for major customers and enterprise clients are roughly the same.
In terms of the Chinese market, Jensen Huang stated that Q4 revenue is generally flat compared to the previous quarter.
The following are key points from the conference call:
1. About the future of inference-specific clusters
Question: As the boundaries between training and inference become increasingly blurred, what will be the future development of inference-specific clusters? What impact will this have on NVIDIA and its customers?
Key points from Jensen Huang's response:
Future AI models will have multiple dimensions of expansion: pre-training, post-training (such as reinforcement learning, fine-tuning), and inference time extension.
Inference demand will grow significantly, especially for long-thinking inference AI models, whose computational demands may be several orders of magnitude higher than pre-training.
The Blackwell architecture is designed for inference AI, with inference performance improved by 25 times compared to Hopper and costs reduced by 20 times.
NVIDIA's architecture is highly versatile and can flexibly adapt to different AI workloads, efficiently running from pre-training to inference
2. About the Promotion of GB200 and System Complexity
Question: How is the situation of GB200 at CES? What are the complexities at the system level and the challenges of promotion? Has the enthusiasm for the NGL72 platform changed?
Key points from the unnamed spokesperson (possibly Jensen Huang):
The promotion of GB200 is progressing smoothly, despite facing complexity challenges, but demand is strong.
The production of Blackwell (GB200) involves 350 factories and 1.5 million components, achieving $11 billion in revenue last quarter.
Major cloud service providers (CSPs) such as Azure, GCP, AWS, and OCI have begun deploying the Blackwell system.
Enthusiasm for the NGL72 platform remains unchanged, and it is expected to continue driving the development of AI infrastructure.
3. About Gross Margin and Future Demand
Question: Is the first quarter the bottom for gross margin? How sustainable is future demand? Have innovations like DeepSeek changed the outlook for the future?
Key points from Colette Kress:
During the promotion of Blackwell, gross margin will be in the low 70s, and it is expected to recover to the mid-70s by the end of the year as full promotion occurs.
Currently focused on accelerating the manufacturing of the Blackwell system to meet customer demand.
Key points from Jensen Huang:
Capital investment in data centers continues to grow, and AI will become the primary workload for data centers.
Emerging fields such as enterprise AI, agent AI, and physical AI will drive long-term demand growth.
The activity and innovation of startups indicate that the AI market has huge potential, and demand will remain strong.
4. About the Launch of Blackwell Ultra
Question: What are the plans for the launch of the next-generation Blackwell Ultra? How to manage the promotion of both products while the current generation Blackwell is still being promoted?
Key points from Jensen Huang:
The Blackwell Ultra is planned to launch in the second half of the year, bringing improvements in networking, memory, and processors.
The company is working closely with customers and the supply chain to ensure a smooth transition.
Blackwell Ultra will seamlessly integrate with the existing system architecture, continuing to drive the development of AI infrastructure.
5. About the Balance Between Custom ASICs and Commercial GPUs
Question: How is the balance between custom ASICs and commercial GPUs? Are customers planning to build heterogeneous superclusters that use both GPUs and ASICs?
Key points from Jensen Huang:
NVIDIA's GPU architecture is highly versatile and suitable for various AI models and workloads
Custom ASICs are typically targeted at specific applications, while the flexibility and ecosystem of GPUs make them the preferred choice for most AI applications.
Performance and cost efficiency give GPUs a significant advantage in AI data centers, especially in inference and training workloads.
6. About Geographic Distribution and Growth
Question: Can the growth of the U.S. market compensate for potential declines in other regions? Will this change in geographic distribution affect growth?
Jensen Huang's key points in response:
There is strong global demand for AI technology, and the proportion of the Chinese market remains stable.
AI has become a mainstream technology, widely applied across various industries, from financial services to healthcare.
In the long term, AI will penetrate more industries, driving global economic growth.
7. About the Growth of Enterprise AI
Question: What is the growth trend of enterprise AI? Will it become a larger part of the consumer portfolio?
Jensen Huang's key points in response:
The enterprise AI market is growing rapidly, particularly in autonomous vehicles, robotics, and industrial applications.
Enterprises will increasingly adopt AI technology to enhance productivity and efficiency.
NVIDIA's full-stack AI solutions will support enterprises throughout the entire AI workflow, from pre-training to inference.
8. About Infrastructure Upgrade Cycles
Question: What is the upgrade cycle for deployed infrastructure? When will we see upgrade opportunities?
Jensen Huang's key points in response:
The current AI infrastructure is still using various NVIDIA products, such as Voltas, Pascals, and Amperes.
As AI technology evolves, enterprises will gradually update their infrastructure to leverage the latest GPU technologies.
NVIDIA's CUDA platform ensures compatibility across different generations of GPUs, making the upgrade process more flexible.
9. About Gross Margin and Tariff Impact
Question: What are the future trends for gross margin? How do tariffs affect gross margin?
An unnamed spokesperson (possibly Colette Kress) key points in response:
Gross margin is influenced by various factors, including the yield and configuration of Blackwell.
The company is working to improve gross margin, with expectations of improvement in the second half of the year.
The impact of tariffs is still uncertain, and the company will continue to comply with relevant regulations.
The following is the transcript of the conference call, translated by AI:
Colette Kress, Executive Vice President and Chief Financial Officer:
The fourth quarter was another record quarter.
Revenue was $39.3 billion, a 12% quarter-over-quarter increase and a 78% year-over-year increase, exceeding our expected $37.5 billion. For fiscal year 2025, revenue is projected to be $130.5 billion, a 114% increase over the previous fiscal year. Let's start with the data center. The data center revenue for fiscal year 2025 is $115.2 billion, doubling from the previous fiscal year In the fourth quarter, data center revenue reached a record $35.6 billion, a quarter-over-quarter increase of 16% and a year-over-year increase of 93%. With the launch of Blackwell and the continued growth of Hopper 200, sales of Blackwell in the fourth quarter exceeded our expectations. We achieved $11 billion in Blackwell revenue to meet strong demand.
This is the fastest product rollout in our company's history, with unprecedented speed and scale. Production of Blackwell is in full swing, covering multiple configurations, and we are rapidly increasing supply and expanding customer adoption. Our data center computing revenue in the fourth quarter grew 18% quarter-over-quarter and more than doubled year-over-year. Customers are racing to expand infrastructure to train the next generation of cutting-edge models and unlock the next level of AI capabilities.
With Blackwell, clusters starting with 100,000 or more GPUs are becoming common. Multiple sets of infrastructure of this scale have already begun shipping. Customization and fine-tuning of trained models are driving demand for NVIDIA infrastructure and software, as developers and enterprises leverage techniques such as fine-tuning, reinforcement learning, and distillation to tailor models for specific domain use cases. Hugging Face alone hosts over 90,000 versions derived from the Llama base model.
The scale of customization and fine-tuning of trained models is enormous, with overall computational demands potentially several orders of magnitude higher than pre-training. Our inference demand is accelerating, driven by scaling during testing and new inference models (such as OpenAI's o3, DeepSeek-R1, and Grok 3). Long-form reasoning AI may require 100 times the computational power of one-time inference. Blackwell is designed for inference AI reasoning. Compared to Hopper 100, Blackwell can increase the throughput of inference AI models by up to 25 times while reducing costs by 20 times. It is revolutionary. The Transformer Engine is built for LLM and expert mixed inference. Its NVLink domain provides 14 times the throughput of PCIe Gen5, ensuring response time, throughput, and cost efficiency to handle increasingly complex inference scales.
Companies across various industries are leveraging NVIDIA's full-stack inference platform to enhance performance and reduce costs. NAP tripled the inference throughput of its screenshot feature using NVIDIA TensorRT and cut costs by 66%. Perplexity processes 435 million queries per month and reduced inference costs by three times using NVIDIA Triton inference server and TensorRT LLM. Microsoft Bing achieved a five-fold speed increase and significant total cost of ownership (TCO) savings in visual search across billions of images using NVIDIA TensorRT and accelerated libraries. There is huge demand for Blackwell in inference. Many early GB200 deployments have been designated for inference, which is a first for the new architecture. Blackwell covers the entire AI market from pre-training, post-training to inference, from cloud to on-premises to enterprise applications CUDA's programmable architecture accelerates every AI model and over 4,400 applications, ensuring protection of large infrastructure investments from obsolescence in a rapidly evolving market. Our performance and innovation speed are unparalleled.
We are committed to reducing inference costs by 200 times in just two years. We offer the lowest total cost of ownership (TCO) and the highest return on investment (ROI), along with full-stack optimization for NVIDIA and our vast ecosystem of 5.9 million developers who continuously improve the economic benefits for our customers. In the fourth quarter, large cloud service providers (CSPs) accounted for about half of our data center revenue, with these sales nearly doubling year-over-year. Large CSPs were among the first to deploy Blackwell, with Azure, GCP, AWS, and OCI introducing GB200 systems into cloud regions worldwide to meet the surge in customer demand for AI.
The share of regional clouds hosting NVIDIA GPUs in global data center revenue is rising, reflecting the ongoing construction of AI factories worldwide and the rapid growth in demand for AI inference models and agents. Of course, we have launched instances based on 100,000 GB200 clusters equipped with NVLink switches and Quantum-2 InfiniBand. Consumer internet revenue has tripled year-over-year, thanks to the expanding use cases for generative AI and deep learning, including recommendation systems, visual language understanding, synthetic data generation search, and agent AI. For example, XAI is adopting GB200 to train and infer its next-generation Grog AI model. Meta's cutting-edge Andromeda advertising engine runs on NVIDIA's Grace Hopper superchip, delivering a large volume of ads across applications like Instagram and Facebook. Andromeda leverages Grace Hopper's fast interconnect and large memory to triple inference throughput, enhance ad personalization, and achieve significant monetization and ROI growth.
Enterprise revenue has nearly doubled year-over-year, driven by accelerated demand for model fine-tuning, RAG, agent AI workflows, and GPU-accelerated data processing. We have launched the NVIDIA Llama Nemotron model family NIMs to help developers create and deploy AI agents across a range of applications, including customer support, fraud detection, and product supply chain and inventory management. Leading AI agent platform providers, including SAP and ServiceNow, are among the first to adopt the new models.
Leaders in the healthcare sector, such as IQVIA, Illumina, Mayo Clinic, and Arc Institute, are leveraging NVIDIA AI to accelerate drug discovery, enhance genomic research, and pioneer advanced medical services with generative and agent AI. As AI expands from the digital world into the physical world, NVIDIA's infrastructure and software platforms are increasingly being adopted to drive the development of robotics and physical AI In the field of robotics and autonomous vehicles, it is one of the earliest and largest applications, with almost every autonomous vehicle company developing NVIDIA technology in data centers, vehicles, or both.
NVIDIA's automotive vertical business revenue is expected to reach approximately $5 billion in this fiscal year. At CES, Hyundai Motor Group announced that it will adopt NVIDIA technology to accelerate the development of autonomous vehicles and robotics, as well as smart factory plans. Visual transformers, self-supervised learning, multimodal sensor fusion, and high-fidelity simulation are driving the development of autonomous vehicles and will require more than ten times the computing power. At CES, we announced the NVIDIA Cosmos World Foundation Model Platform. Just as language foundation models have revolutionized language AI, Cosmos is a physical AI designed to fundamentally change robotics. Leading robotics and automotive companies, including ride-sharing giant Uber, are among the first to adopt the platform.
From a regional perspective, due to the initial promotion of Blackwell, the revenue from data centers in the United States has seen the strongest sequential growth. Countries around the world are building their own AI ecosystems, leading to a surge in demand for computing infrastructure. France's €200 billion AI investment and the EU's €200 billion AI plan are just glimpses of the future years that will redefine global AI infrastructure construction.
In terms of the proportion of data center revenue, sales from Chinese data centers are still far below the levels at the beginning of export controls. In the absence of regulatory changes, we expect shipments in China to remain at current levels. The competition for data center solutions in the Chinese market is very fierce. We will continue to comply with export control regulations while serving our customers.
Network revenue decreased by 3% sequentially. Our network connected to GPU computing systems is very strong, exceeding 75%. We are transitioning from small NVLink 8 with InfiniBand to large NVLink 72 with Spectrum X. The increase in revenue from Spectrum X and NVLink switches represents an important new growth area. We expect the network to recover growth in the first quarter.
AI requires a new category of networks. NVIDIA provides NVLink switch systems for scaling up computing. For scaling out, we offer Quantum and InfiniBand for HPC supercomputers, as well as Spectrum X for Ethernet environments. Spectrum X enhances Ethernet for AI computing and has achieved great success. Companies like Microsoft Azure, OCI, and CoreWeave are using Spectrum X to build large AI factories. The first Stargate data center will use Spectrum X. Yesterday, Cisco announced the integration of Spectrum X into its networking portfolio to help enterprises build AI infrastructure. With its large enterprise customer base and global influence, Cisco will bring NVIDIA Ethernet to every industry Now turning to gaming and AI PCs. Gaming revenue was $2.5 billion, down 22% quarter-over-quarter and down 11% year-over-year. Full-year revenue was $11.4 billion, up 9% year-over-year, with strong demand throughout the holiday period. However, fourth-quarter shipments were impacted by supply constraints. We expect strong quarter-over-quarter growth in the first quarter as supply increases. The new GeForce RTX 50 series desktop and laptop GPUs have been launched. They are designed for gamers, creators, and developers, integrating AI and graphics technology to redefine visual computing. Powered by the Blackwell architecture, with fifth-generation Tensor cores and fourth-generation RT cores, and up to 3,400 AI POPs. These GPUs achieve a 2x performance leap and introduce new AI-driven rendering technologies, including neural shaders, digital human technology, geometry, and lighting. The new VLSS4 technology boosts frame rates by 8x through AI-driven frame generation, converting one rendered frame into three. It also applies the Transformer model in real-time for the first time in the industry, with 2x the parameters and 4x the computing power, supporting unparalleled visual fidelity. We also announced a range of GeForce Blackwell laptop GPUs equipped with the new NVIDIA Max-Q technology, which can extend battery life by an astonishing 40%. These laptops will be launched by top manufacturers worldwide starting in March. Next is our professional visualization business. Revenue was $511 million, up 5% quarter-over-quarter and up 10% year-over-year. Full-year revenue was $1.9 billion, up 21% year-over-year. Key industry verticals driving demand include automotive and healthcare. NVIDIA technology and generative AI are reshaping design, engineering, and simulation workloads. An increasing number of leading software platforms, such as Ansys, Cadence, and Siemens, are leveraging these technologies, driving demand for NVIDIA RTX workstations. Now turning to the automotive business. Revenue reached a record $570 million, up 27% quarter-over-quarter and up 103% year-over-year. Full-year revenue was $1.7 billion, up 55% year-over-year. Strong growth is attributed to the continued rise of autonomous vehicles (including cars and robotic taxis). At CES, we announced that Toyota, the world's largest automaker, will adopt NVIDIA Orin in its next-generation vehicles and run the safety-certified NVIDIA DRIVE OS. We announced that Aurora and Continental will deploy autonomous trucks at scale, powered by NVIDIA DRIVE 4. Finally, our end-to-end autonomous vehicle platform, NVIDIA DRIVE Hyperion, has passed industry safety assessments by Su-Su and Su-Ryland, both authorities in automotive safety and cybersecurity. NVIDIA is the first to receive comprehensive third-party evaluation for an autonomous vehicle platform
Alright, next is the remainder of the income statement. The GAAP gross margin is 73%, and the non-GAAP gross margin is 73.5%, which decreased quarter-over-quarter as we delivered the Blackwell architecture for the first time, as expected. As discussed in the last quarter, Blackwell is a customizable AI infrastructure with various types of NVIDIA-manufactured chips, multiple networking options, and configurations suitable for air and liquid-cooled data centers. We exceeded expectations in promoting Blackwell in the fourth quarter, increasing system availability and providing our customers with various configurations. With the promotion of Blackwell, we expect the gross margin to be in the low 70s. Initially, we focused on accelerating the manufacturing of Blackwell systems to meet strong customer demand for building Blackwell infrastructure. When Blackwell is fully promoted, we have many opportunities to reduce costs, improve gross margins, and restore them to the mid-70s later in this fiscal year. Quarter-over-quarter, GAAP operating expenses grew by 9%, and non-GAAP operating expenses grew by 11%, reflecting higher engineering development costs and increased computing and infrastructure costs associated with new product launches. In the fourth quarter, we returned $8.1 billion to shareholders in the form of stock buybacks and cash dividends.
Let me turn to the outlook for the first quarter. Capital revenue is expected to be $43 billion, with a fluctuation of 2% up or down. With strong demand continuing, we expect Blackwell to achieve significant growth in the first quarter. We anticipate both the data center and gaming businesses will see quarter-over-quarter growth. Within the data center, we expect both computing and networking to achieve quarter-over-quarter growth. GAAP and non-GAAP gross margins are expected to be 70.6% and 71%, respectively, with a fluctuation of 50 basis points up or down. GAAP and non-GAAP operating expenses are expected to be approximately $5.2 billion and $3.6 billion, respectively. We expect operating expenses for the full fiscal year 2026 to grow to the mid-30% range. GAAP and non-GAAP other income and expenses are expected to be approximately $400 million in income, excluding gains and losses from non-public and public equity securities. GAAP and non-GAAP tax rates are expected to be 17%, with a fluctuation of 1%, excluding any one-time items. More financial details are included in the CFO's commentary and other information available on our investor relations website, including a new financial information AI agent. In closing, let me highlight the upcoming financial events.
We will participate in the TD Cowen Healthcare Conference in Boston on March 3, and the Morgan Stanley Technology, Media, and Telecom Conference in San Francisco on March 5. Please join us for our annual GTC conference starting on Monday, March 17, in San Jose, California. Jensen will deliver a news-rich keynote speech on March 18, and we will hold a Q&A session for our financial analysts on March 19. We look forward to seeing you at these events
We have scheduled the earnings call to discuss the results for the first quarter of fiscal year 2026 on May 28, 2025. We will now hand the call over to the operator to begin the Q&A session. It would be great if you could start.
Q&A Session
Host: Thank you. The next question comes from CJ Muse of Cantor Fitzgerald. Please go ahead.
CJ Muse: Yes, good afternoon. Thank you for taking my question.
What I would like to ask is, Jensen, as TEFCON computing and reinforcement learning show such tremendous potential, we are clearly seeing the boundaries between training and inference becoming increasingly blurred. What does this mean for the future development of clusters that may be specifically used for inference? What overall impact do you think this will have on NVIDIA and its customers? Thank you.
Jensen Huang, Founder, President, and CEO:
Yes, I appreciate you, CJ. There are now multiple scaling laws. The first is the pre-training scaling law.
And this will continue to scale because we have multimodal. We have data from inference that is now being used for pre-training. Then the second part is the post-training scaling law using reinforcement learning, human feedback, reinforcement learning AI feedback, and reinforcement learning verifiable rewards. In fact, the computational load for post-training is higher than for pre-training.
This is somewhat reasonable because you can generate a large amount of synthetic data or synthetically generated labels when using reinforcement learning. AI models are essentially generating labels to train AI models. That’s post-training. The third part, which you mentioned, is the computational or inference scaling during testing, long thinking, and reasoning.
They are essentially the same concept. There, you have chains of thought, you have search. The number of generated labels and the required inference computation has already exceeded that of the initial large language model's one-off examples and one-off capabilities by a hundred times. And this is just the beginning.
This is just the start. The next idea is that the next generation may have thousands or even more times the amount of inference, and we hope that future models can engage in extremely deep thinking, with models based on simulation and search potentially being tens of thousands or even millions of times higher than today. So, the question arises, how do you design such architectures? Some models are autoregressive. Some models are diffusion-based.
Sometimes you want your data center to perform decentralized inference. Sometimes it is compact. Therefore, it is difficult to determine the optimal configuration for a data center, which is also why NVIDIA's architecture is so popular. We run every kind of model.
We excel in training. Most of our computation today is actually inference. And Blackwell has taken all of this to a new level. We considered inference models when designing Blackwell.
When you look at training, its performance is many times higher. But what is truly astonishing is that for long thinking, testing scaling, and reasoning AI models, their speed is ten times faster, with 25 times higher throughput. So, Blackwell will perform excellently in every aspect When you have an architecture that can configure and use your data center based on whether you are doing more pre-training, post-training, or expanding your reasoning, our architecture is universal and easy to use in all these different ways. In fact, what we are seeing is a more centralized unified architecture than ever before.
Host:
The next question comes from Joe Moore of Morgan Stanley. Please go ahead.
Joe Moore: Actually, I'm from Morgan Stanley, thank you.
I would like to know about the GB200 at CES. You mentioned the complexity of rack-level systems and the challenges you face in your prepared remarks. Then, as you said, we have seen a lot of situations of general availability, so where do you stand in terms of promotion? At the system level, aside from the chip level, are there any bottlenecks to consider? Also, has your enthusiasm for the NGL72 platform changed?
Unnamed Speaker:
Well, I am more enthusiastic today than I was at CES. The reason is that we have shipped a lot since CES. We have 350 factories producing 1.5 million components needed for each Blackwell rack. Yes, it is extremely complex, and we have successfully and incredibly ramped up the capacity of Grace Blackwell, achieving $11 billion in revenue last quarter. We will have to continue to scale up because the demand is quite high, and customers are eager and impatient to get their Blackwell systems.
You may have seen quite a bit online about the celebration of the Grace Blackwell systems going live. Of course, we have as well. We have installed a considerable number of Grace Blackwells for our engineering, design, and software teams. CoreWeave has publicly announced the successful launch of their systems. Microsoft has also announced it. Of course, OpenAI has announced it as well, and you are starting to see many systems go live. I want to answer your question by saying that there is nothing easy about what we are doing, but we are doing well, and all of our partners are doing well too.
Host: The next question comes from Vivek Arya of Bank of America Securities. Please go ahead.
Vivek Arya:
Thank you for taking my question. I just wanted to know if you could confirm whether the first quarter is the bottom for gross margins. Then, Jensen, my question is, what is on your dashboard that gives you confidence that this strong demand will continue into next year, and has DeepSeek and any innovations they bring changed this view in any way? Thank you.
Colette Kress, Executive Vice President and Chief Financial Officer:
Let me first answer the question about gross margins. During our Blackwell rollout, our gross margins will be in the low 70s. Currently, we are focused on accelerating our manufacturing to ensure we can deliver products to customers as quickly as possible
Our Blackwell has been fully promoted, and once our Blackwell is fully promoted, we can reduce costs and improve our gross margin. Therefore, we expect to possibly reach the mid-70s by the end of the year. As you heard Jensen talk about the systems and their complexities. They are customizable, in some cases. They have multiple networking options. They have liquid cooling and water cooling. Therefore, we know we have the opportunity to improve these gross margins in the future. But for now, we will focus on completing manufacturing and delivering the products to our customers as soon as possible.
Jensen Huang, Founder, President, and CEO:
We know a few things, Vivek. We have a fairly clear understanding of the capital investments being made in data centers. We know that from now on, most software will be based on machine learning. Therefore, accelerated computing and generative AI, inferencing AI will be the types of architectures your data center will want.
Of course, we have forecasts and plans from top partners. We also know that there are many innovative and exciting startups continuously emerging as new opportunities for developing the next generation of AI breakthroughs, whether it be agent AI, inferencing AI, or physical AI. The number of startups remains quite active, and each startup requires a considerable amount of computing infrastructure. Therefore, I think whether it’s short-term signals or mid-term signals, short-term signals are certainly things like purchase orders and forecasts, while mid-term signals will be the scale of infrastructure and capital expenditures compared to the past.
Then long-term signals relate to the fact that we know fundamentally, software has shifted from hand-coded running on CPUs to machine learning and AI-based software running on GPUs and accelerated computing systems. Therefore, we have a fairly clear understanding that this will be the future of software, and perhaps another way to think about it is that we are really just touching the early stages of consumer AI and search, as well as some consumer generative AI, advertising, and recommendation systems. The next wave is coming, with enterprise agent AI, robotic physical AI, and different regions building sovereign AI for their ecosystems. All of this is just beginning, and we are able to see them.
We are able to see them because we are clearly at the center of these developments, and we can see a lot of activity happening in all these different places that will occur. Therefore, whether it’s short-term, mid-term, or long-term signals, we have a fairly clear understanding.
Host: The next question comes from Harlan Sur of Morgan Stanley. Please go ahead.
Harlan Sur:
Yes, good afternoon. Thank you for taking my question. Your next-generation Blackwell Ultra is planned for launch in the second half of the year, in line with the team’s annual product cadence. Jensen, given that you are still promoting the current generation of Blackwell solutions, could you help us understand the demand dynamics for Ultra? How are your customers and supply chain managing the rollout of both products simultaneously, and is the team still on track to execute the launch of Blackwell Ultra in the second half of the year?
Jensen Huang, Founder, President, and CEO:
Yes.
Blackwell Ultra will be launched in the second half of the year. As you know, the first generation of Blackwell had some minor issues that may have delayed us by two months. Of course, we have fully recovered. The team has done an outstanding job in the recovery process, and all our supply chain partners, along with so many people, helped us recover at lightning speed. Therefore, we have successfully increased the production capacity of Blackwell. But that hasn't stopped the next train. The next train follows an annual rhythm, and Blackwell Ultra will be equipped with new networks, new memory, and of course new processors, among other things, all of which will be launched. We have worked with all partners and customers to plan this.
They have all the necessary information. We will work with everyone for a proper transition. This time, the system architecture between Blackwell and Blackwell Ultra is completely the same. The transition from Hopper to Blackwell was much more difficult because we shifted from a system based on NVLink 8 to one based on NVLink 72. Therefore, everything, including the chassis, system architecture, hardware, power transmission, etc., had to change. This was quite a challenging transition. But the next transition will be seamless. Blackwell Ultra will be seamlessly integrated.
We have also revealed and closely collaborated with all partners to prepare for the subsequent transition. The subsequent transition is called Vera Rubin. All our partners are keeping pace with this transition. Therefore, we are preparing for this transition, emphasizing again that we will bring a huge leap forward.
So please come to GTC, and I will talk to you about Blackwell Ultra, Vera Rubin, and then showcase the one that follows. Very exciting new products. So please come to GTC.
Host: The next question comes from Timothy Arcuri of UBS. Please go ahead.
Timothy Arcuri:
Thank you very much. Jensen, we often hear about custom ASICs. Can you talk about the balance between custom ASICs and commercial GPUs? We hear that some heterogeneous superclusters use both GPUs and ASICs simultaneously. Is this something that customers are planning to build, or will these infrastructures remain relatively independent? Thank you.
Jensen Huang, Founder, President, and CEO:
Well, what we build is very different from ASICs.
In some ways, we are completely different, and in some areas, we have overlaps. We differ in several aspects. NVIDIA's architecture is general-purpose. Whether you are optimizing for autoregressive models, diffusion-based models, vision-based models, multimodal models, or text-based models, we excel at it. We are good at all of this because of our flexible architecture and rich software stack ecosystem, making us the preferred target for most exciting innovations and algorithms Therefore, by definition, we are much more general than narrow ASICs. We are really good from start to finish, from data processing, training data curation, to training data, and of course, reinforcement learning for post-training, all the way to inference scaling during testing. So, we are general, we are end-to-end, we are everywhere. And because our architecture is not limited to just one cloud, we can operate in any cloud, on-premises, and in robots. Our architecture is much more general than just one cloud; it is a great goal and the preferred target for anyone starting a new company. So, we are everywhere. The third thing I want to say is that our performance and our pace are incredibly fast.
Please remember that these data centers are always fixed in size. They are fixed in size or fixed in power. If our performance per watt is 2 times, 4 times, or even 8 times higher, this is not uncommon, and it will directly translate into revenue. So, if you have a 100-megawatt data center, if the performance or throughput of a 100-megawatt or gigawatt data center is 4 times or 8 times higher, then the revenue of that gigawatt data center is 8 times higher. This is different from past data centers because AI factories are directly monetizable through the tokens they generate. Therefore, the token throughput of our architecture is so fast, which is very valuable for all companies building these systems for revenue generation purposes and capturing rapid return on investment (ROI). So, I think the third reason is performance. Then, I want to say that the software stack is very complex. Building ASICs is not simpler than what we do. We had to build new architectures, and the ecosystem built on our architecture is 10 times more complex than it was two years ago. This is evident because the amount of software built on our architecture has grown exponentially, and AI is rapidly evolving. So, building the entire ecosystem on multiple chips is challenging.
So, I want to say that there are these four reasons, and finally, I want to say that just because a chip is designed does not mean it will be deployed. You have seen this happen many times; many chips are discarded. But when it comes to deployment, business decisions need to be made. This business decision is about deploying a new engine, a new processor in a limited AI factory, which is limited in size, power, and time. Our technology is not only more advanced and performs better, but it also has better software capabilities, and importantly, our deployment capability is very fast. So, these things are not easy, as everyone knows now. So, there are many different reasons why we are doing well and why we can succeed.
Host: The next question is from Ben Reitz of Melius Research. Please go ahead.
Ben Reitz:
Yes. Hi, I’m Ben Reitz. Hey, thank you for taking my question. Hey, Jensen, this is a geography-related question. You have excellently explained some of the underlying factors supporting demand However, the United States has grown by about $5 billion quarter-on-quarter, and I think there are concerns about whether the U.S. can fill the gap when facing regulatory restrictions in other regions. I just want to know, as we move through this year, if this kind of growth in the U.S. continues, whether it is appropriate, and whether it supports your growth rate. How can you grow so quickly despite this mixed transition towards the U.S.? Your guidance seems to suggest that China may grow quarter-on-quarter, so I wonder if you could elaborate on this dynamic and possibly weigh it. Thank you.
Jensen Huang, Founder, President, and CEO:
The proportion from China is about the same as in the fourth quarter and previous quarters. It's about half of what it was before the export controls.
But its proportion is about the same. Regarding geographical regions, the key point is that AI is software. It is modern software. It is incredibly modern software, but it is software. And AI has been mainstreamed. AI is used in every consumer service. If you are buying a pint of milk, it is delivered to you by AI. So, almost every consumer service is centered around AI. Every student will use AI as a tutor. Medical services use AI, financial services use AI. No fintech company would not use AI. Every fintech company will. Climate tech companies use AI. Mineral exploration now uses AI. Every higher education institution, every university uses AI. So, I think it is quite safe to say that AI has been mainstreamed, and it has been integrated into every application. Our hope is, of course, that this technology can advance society safely and beneficially.
Then, the last point, I think we are at the beginning of this new era. When I say "beginning," I mean that behind us are decades of built data centers and computers that were built for manual coding, general computing, and CPUs. Looking ahead, I think it is quite safe to say that the future world will have almost all software integrated with AI. All software and all services will ultimately be based on machine learning. The data flywheel will become part of improving software and services.
Future computers will be accelerated. Future computers will be based on AI. We have really only been on this journey for a few years, modernizing those computers that have taken decades to build. So, I am quite certain that we are at the beginning of this new era.
And finally, no technology has ever had the opportunity to address a larger portion of the world's GDP like AI has. No software tool has ever had that. So, this is a software tool that can now address a larger portion of the world's GDP than at any time in history. So, the way we think about growth and the way we think about whether something is big or small must be in this context. When you step back and look at it from this perspective, we are really just at the beginning of this new era.
Host: The next question comes from Aaron Rakers of Wells Fargo. Please go ahead. Aaron, your line is open. The next question comes from Mark Lipacis of Evercore ISI Please continue.
Mark Lipacis:
Hi, I'm Mark Lipacis. Thank you for taking my questions. I have a clarification question and a question. Colette, regarding the clarification question, did you say that enterprise data center growth year-over-year doubled in January? If so, does that mean its growth rate is faster than that of hyperscale cloud service providers (CSPs)? Then, Jensen, my question is that hyperscale cloud service providers are the largest buyers of your solutions, but the equipment they purchase is used for both internal workloads and external workloads, with external workloads being the cloud services used by enterprises.
So, the question is, can you give us a rough idea of the spending allocation between internal workloads and external workloads for hyperscale cloud service providers? With the emergence of these new AI workloads and applications, do you expect enterprises to become a larger part of the consumption mix? Will this affect the way you develop services and ecosystems? Thank you.
Colette Kress, Executive Vice President and Chief Financial Officer:
Certainly, thank you for your question about our enterprise business. Yes, it has doubled, very similar to what we see with large cloud service providers. Keep in mind that both areas are important.
Working with cloud service providers allows for work on large language models as well as their own inference work, but remember, this is also where enterprises come in. So, your enterprise is working both with your cloud service providers and building their own systems. They are both, right, growing quite well.
Jensen Huang, Founder, President, and Chief Executive Officer:
Cloud service providers account for about half of our business.
Cloud service providers have both internal consumption and external consumption, as you mentioned. Of course, we work closely with them to optimize internal workloads because they have a large number of NVIDIA devices that they can leverage. And since we can use them for AI on one side, video processing on the other, and also for data processing like Spark, we are versatile.
Therefore, if our devices have a longer lifespan, then the total cost of ownership (TCO) will also be lower. Now, the second question is, how do we view the future growth of enterprises that are not cloud service providers (CSPs)? The answer is, I believe that in the long term, the enterprise segment will occupy a larger share. The reasons are as follows:
If you look at today's computer industry and the parts of the computer industry that remain unserved, it is mainly industrial. So, let me give you an example. When we talk about enterprises, take automotive companies as an example, as they produce both software products and hardware products. So, in the case of automotive companies, employees will be what we refer to as the enterprise segment, using AI and software planning systems and tools. We have some very exciting things to share with everyone at GTC, and these agent systems are designed to enhance employee productivity for designing, marketing, planning, and operating the company These are agent AIs. On the other hand, the cars they manufacture also need AI. They need an AI system to train these cars, manage this vast fleet of vehicles, with 1 billion cars on the road today and another 1 billion in the future, each car will be a robotic car, all of which will collect data. We will use AI factories to improve them, just as they have car factories today, in the future they will have both car factories and AI factories.
Inside the car is a robotic system, so as you can see, there are three computers involved. One computer helps people, one computer builds AI for machines, it can be a horse, it can be a tractor, it can be a lawnmower, it can be a person or a robot being developed, it can be a building, it can be a warehouse. These physical systems need a new AI, which we call physical AI. They need to understand not only the meaning of words and language but also the physical significance of the world, friction and inertia, the permanence of objects and causality, and all these things that are common sense to us, but AI needs to learn these physical effects, so we call it physical AI.
The whole part of using agent AI to fundamentally change the way companies operate internally is just beginning. We are now at the start of the agent AI era, and you hear many people talking about it. We have some very exciting things happening, and then there is physical AI, and then there are robotic systems, so these three computers are all new. My feeling is that in the long run, this will be one of the biggest parts, which makes sense to some extent because most of the world's GDP is represented by heavy industry or industrial enterprises and the companies that serve them.
Host: The next question comes from Aaron Rakers of Wells Fargo. Please go ahead.
Aaron Rakers:
Yes, thank you for the opportunity to ask a question again. Jensen, as we approach the second anniversary of the Hopper inflection point, how do you see this inflection point in 2023, along with the rise of generative AI? When we consider the road ahead of you, how do you view the infrastructure that has already been deployed in terms of replacement cycles, whether it's the GB300 or the Rubin cycle? When do we start to see potential upgrade opportunities? I'm just curious how you view this issue.
Jensen Huang, Founder, President, and CEO:
Yes, I really appreciate it. First of all, people are still using Voltas and Pascals and Amperes.
The reason is that CUDA is so programmable that you can use it. Well, one of the main use cases is data processing and data curation. You find a situation where an AI model is not very good. You present this situation to a visual language model.
Suppose, suppose it is a car. You present this situation to a visual language model. The visual language model actually looks at this situation and says, this is not what I'm good at. Then, you take this response, this prompt, and then you prompt an AI model to find other similar situations in your entire data lake, whatever that situation may be Then you use AI for domain randomization and generate many other examples. You can then train the model from these examples. So you can use Amperes for data processing, data curation, and machine learning-based search.
Then you create a training dataset, and you present this dataset to your Hopper system for training. So, each of these architectures is fully compatible, and they are all CUDA compatible. Therefore, everything can run anywhere. However, if you have already deployed infrastructure, you can place less intensive workloads on the existing installations.
All of our GPUs are fully utilized.
Host: We have time for one more question, and this question comes from Atif Malik of Citibank. Please go ahead.
Atif Malik:
Hi, thank you for taking my question. I have a follow-up question regarding gross margins, Colette. Colette, I know there are many variables, including Blackwell's output, NVLink 72, and the mix of Ethernet. You previously somewhat dodged the question of whether the first quarter is the bottom. However, to reach the mid-70s range you provided by the end of the year, there must be a 200 basis point growth each quarter. We still do not know the impact of tariffs on the broader semiconductor industry.
So, what gives you confidence in this trajectory for the second half of the year?
Unnamed Speaker:
Yes, thank you for your question. Our gross margins are quite complex, considering the materials we use in the Blackwell system and everything else. We have many opportunities to better improve our gross margins over time. Keep in mind that we have many different configurations on Blackwell, which will help us achieve this.
So, working together, after we provide some really strong promotions for our customers, we can start this work. If possible, we will start as soon as we can. If we can improve it in the short term, we will do so. Tariffs, for now, are a bit of an unknown factor.
Until we have further understanding of the U.S. government's plans, whether in terms of timing, location, or how much, it remains unknown. So for now, we are waiting. But of course, we will always comply with regulations such as export controls or tariffs.
Host:
Ladies and gentlemen, this concludes our Q&A session for today. I apologize. Let's turn the time over to Jensen.
Jensen Huang, Founder, President, and CEO:
I just want to thank everyone. Thank you, Colette. The demand for Blackwell is extraordinary. AI is evolving from perception and generative AI to reasoning AI.
With the development of reasoning AI, we have observed another expansion law, which is the expansion of reasoning time or testing time. The more computational effort the model puts into thinking, the smarter the answers become. Models like OpenAI's ROT3 and DeepSeek-R1 are reasoning models that apply reasoning time expansion Inference models can consume 100 times more computational power. Future inference models may consume even more computational power. DeepSeek-R1 has sparked global enthusiasm. This is an outstanding innovation. But more importantly, it has open-sourced a world-class inference AI model. Almost every AI developer is applying R1 or thinking about chain and reinforcement learning techniques to enhance their model's performance. We now have three scaling laws. The scaling laws of AI still exist. Base models are being enhanced through multimodal augmentation, and pre-training is still on the rise. But pre-training alone is no longer sufficient.
We also have two additional scaling dimensions. Post-training scaling, where reinforcement learning, fine-tuning, and model distillation require several orders of magnitude more computational power than pre-training alone. Inference time scaling and inference, where a single query can demand 100 times more computational power. We designed Blackwell for this moment, a single platform that can easily transition from pre-training to post-training and testing time scaling. Blackwell's FP4 Transformer engine and NVLink 72 scaling architecture, along with new software technologies, enable Blackwell to process inference AI models 25 times faster than Hopper. Blackwell and all its configurations are fully in production. Each Grace Blackwell NVLink 72 rack is an engineering marvel. 1.5 million components produced in 350 manufacturing sites, made by nearly 100,000 factory operators.
AI is developing at lightning speed. We are at the beginning of inference AI and inference time scaling. But we are only at the start of the AI era. Multimodal AI, enterprise AI, sovereign AI, and physical AI are just around the corner. We will see strong growth in 2025. Looking ahead, data centers will allocate most of their capital expenditures to accelerated computing and AI. Data centers will increasingly become AI factories. Every company will have its own or rented one. I want to thank everyone for attending our meeting today. Please join us in a few weeks at GTC. We will discuss Blackwell Ultra, Rubin, and other new computing, networking, inference AI, physical AI products, and much more. Thank you