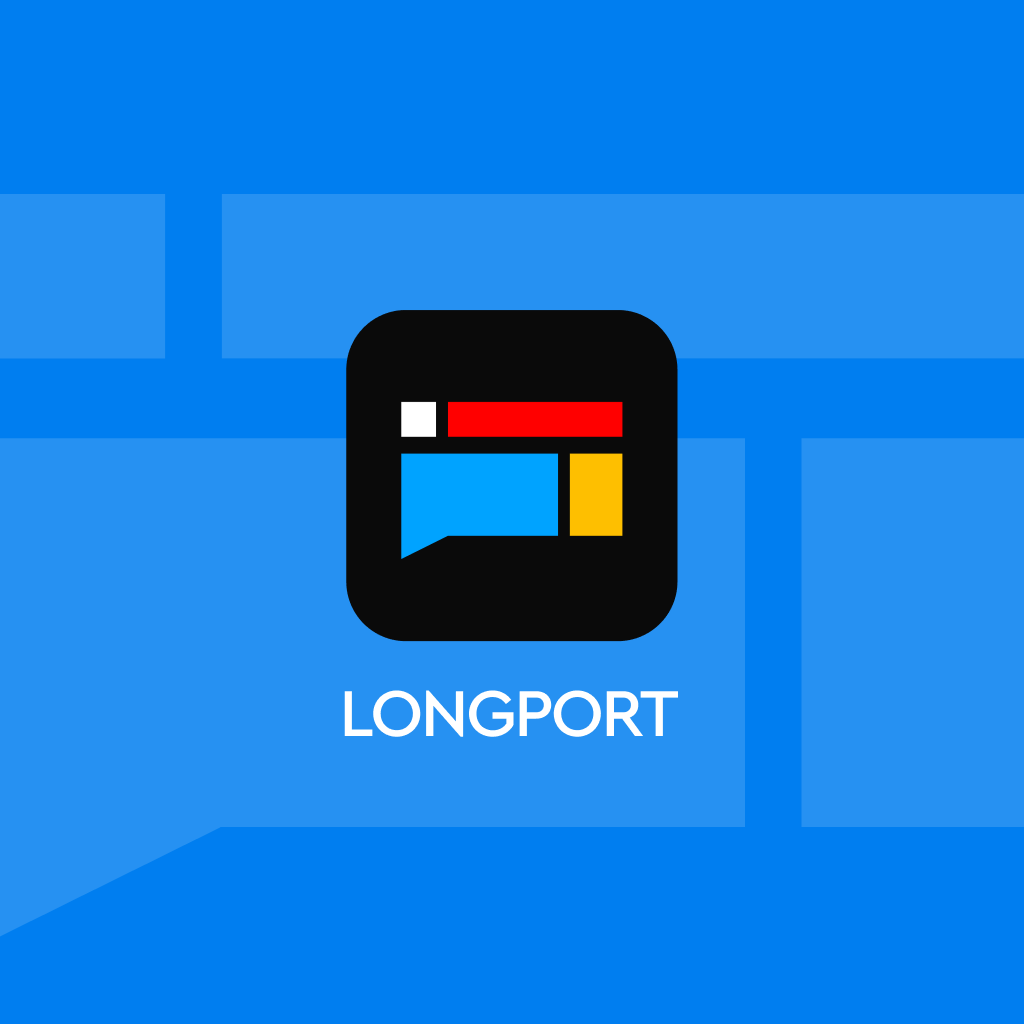
NVIDIA's next move, first optimization of DeepSeek-R1! B200 performance skyrockets 25 times, crushing H100
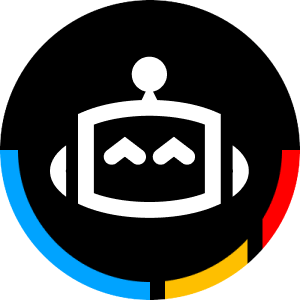
NVIDIA launched the DeepSeek-R1-FP4 optimization solution, achieving a 25-fold performance improvement for the B200, with an inference throughput of 21,088 tokens per second and a cost reduction of 20 times. The new model performed excellently in the MMLU benchmark test, reaching 99.8% of the performance of FP8 models. This optimization solution has been open-sourced on Hugging Face and is suitable for NVIDIA GPUs that support TensorRT-LLM, aiming for efficient and low-cost inference. Netizens expressed amazement, believing that FP4 technology will drive the future development of AI
When the magic of FP4 meets the powerful computing power of Blackwell, what kind of sparks will fly?
The answer is: reasoning performance skyrockets by 25 times, and costs plummet by 20 times!
With the explosive local deployment of DeepSeek-R1, NVIDIA has also personally entered the fray, open-sourcing the first optimization scheme based on the Blackwell architecture—DeepSeek-R1-FP4.
With the support of the new model, B200 achieved an inference throughput of up to 21,088 tokens per second, a 25-fold increase compared to H100's 844 tokens per second.
At the same time, the cost per token has also been reduced by 20 times.
By applying TensorRT DeepSeek optimization on the Blackwell architecture, NVIDIA has enabled models with FP4 production-level accuracy to achieve 99.8% of the performance of FP8 models in the MMLU general intelligence benchmark test.
DeepSeek-R1 Optimized for Blackwell GPU for the First Time
Currently, NVIDIA's FP4-optimized DeepSeek-R1 checkpoint has been open-sourced on Hugging Face.
Model address: https://huggingface.co/nvidia/DeepSeek-R1-FP4
Post-training Quantization
The model quantizes the weights and activations of the linear operators within the Transformer module to FP4, suitable for TensorRT-LLM inference.
This optimization reduces each parameter from 8 bits to 4 bits, thereby decreasing the disk space and GPU memory requirements by approximately 1.6 times.
Deploying with TensorRT-LLM
To deploy the quantized FP4 weight files using the TensorRT-LLM LLM API and generate text responses for given prompts, please refer to the following sample code:
Hardware requirements: NVIDIA GPUs that support TensorRT-LLM (such as B200) are needed, and 8 GPUs are required to achieve tensor_parallel_size=8 tensor parallelism Performance optimization: The code utilizes FP4 quantization, TensorRT engine, and parallel computing, aiming to achieve efficient and low-cost inference, suitable for production environments or high-throughput applications.
Netizens expressed amazement at the results of this optimization.
"FP4 magic keeps AI sharp for the future!" commented netizen Isha.
Netizen algorusty claimed that with this optimization, American suppliers can offer R1 at a price of $0.25 per million tokens.
"There will still be profits."
Netizen Phil linked this optimization with DeepSeek's open-source release this week.
"This demonstrates the potential of combining hardware with open-source models," he stated.
DeepSeek Fully Open Source
Now, DeepSeek's continuous 5-day "Open Source Week" has reached its 3rd day.
On Monday, they open-sourced FlashMLA. This is an efficient MLA decoding kernel specifically designed for NVIDIA Hopper GPU, optimized for variable-length sequences, and has officially been put into production.
On Tuesday, they open-sourced DeepEP, a communication library designed for Mixture of Experts (MoE) and Expert Parallel (EP) systems.
On Wednesday, they open-sourced DeepGEMM. This is a FP8 GEMM (General Matrix Multiplication) computation library that supports dense and MoE models, providing strong support for V3/R1 training and inference.
Overall, whether it's NVIDIA's open-sourced DeepSeek-R1-FP4 or the three repositories open-sourced by DeepSeek, they all promote efficient computation and deployment of AI models through optimization of NVIDIA GPUs and clusters.
Source: [New Intelligence](https://mp.weixin.qq.com/s?srcid=02268MmAPy2fnvm0QsqRod2U&scene=23&sharer_shareinfo=44ce734d3906f5fb8cf9988b3a3dc4c6&mid=2652570048&sn=f1d4e492d4c1d6bc8167cd46946b8f36&idx=1&sharer_shareinfo_first=44ce734d3906f5fb8cf9988b3a3dc4c6&__biz=MzI3MTA0MTk1MA%3D%3D&chks m=f0914b6d4aeeed5e91f6fdcb4d2ba3ebd3eed8c7330051aa08963dc8653e2b97b8e450267843&mpshare=1#rd), Original title: "NVIDIA's next move, first optimization of DeepSeek-R1! B200 performance skyrockets 25 times, crushing H100"
Risk warning and disclaimer
The market has risks, and investment requires caution. This article does not constitute personal investment advice and does not take into account the specific investment goals, financial situation, or needs of individual users. Users should consider whether any opinions, views, or conclusions in this article are suitable for their specific circumstances. Investment based on this is at one's own risk