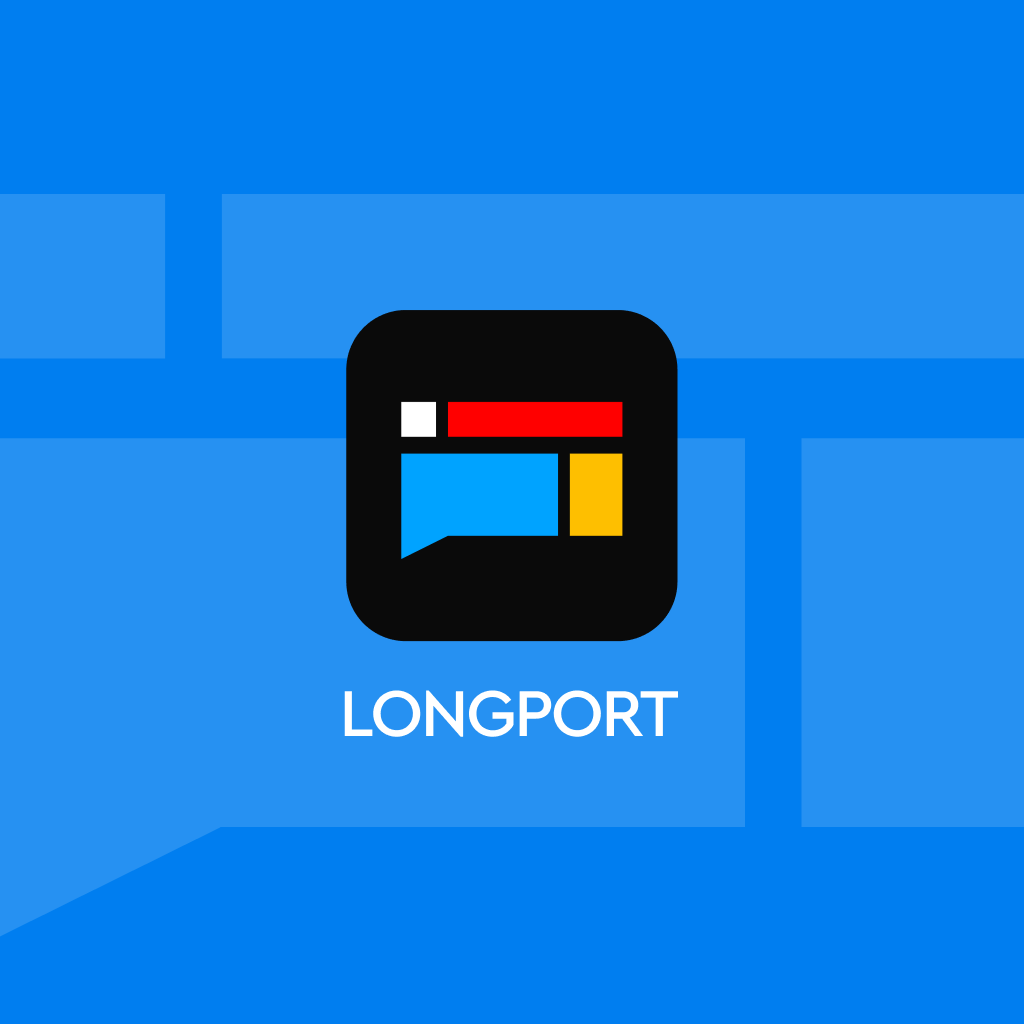
Wu Chao said: Domestic computing power is the most certain investment direction this year
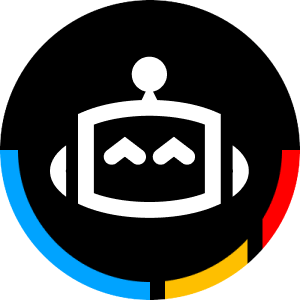
CITIC Construction Investment Securities Research Institute Director Wu Chao pointed out at the 2025 "Artificial Intelligence +" investment strategy meeting that domestic computing power is the most certain investment direction this year, and AI applications are expected to gradually take shape. He emphasized that the demand for computing power is still growing, with intelligent agents and edge AI being the focus of future applications. He suggested that the financial industry should focus on building intelligent agents rather than developing models, and pointed out that AI assistants, search, and video are the main profit scenarios
On February 25th, Wu Chao, the director of the research institute at CITIC Construction Investment Securities and chief analyst for the TMT industry, shared his views on artificial intelligence at the CITIC Construction Investment Securities 2025 "Artificial Intelligence +" investment strategy conference.
The investment representative organized the key points as follows:
-
In 2025, we still clearly favor two directions: domestic computing power will "naturally come to fruition," and AI applications will "take root." Domestic computing power is the most certain investment direction we see this year.
-
The beta of computing power still exists, meaning the demand for computing power has not ended, and there is still distance from a bubble. However, from a structural perspective, the proportion of inference demand will be larger.
-
This year actually feels more like a true 2013, as hardware has been laid out for a long time, penetration rates are starting to increase, and reliable operating systems have emerged, leading to the appearance of today's large models.
However, the exploration of applications is where the future holds the bulk of the industry. For the entire industry to be considered a mainstream industry, truly becoming productive, the value of applications must far exceed that of infrastructure investment.
-
Intelligent agents are the direction we are most optimistic about in applications this year. The second direction we favor is edge AI, and the third is the recently popular robots, also known as generative intelligence, although progress may not be as fast as the first two directions, but the potential is large.
-
Intelligence itself is an application. Intelligence requires a carrier, and currently, terminals and hardware carriers are unavoidable. Whether terminals will bring about a new innovation cycle due to the equalization of intelligent capabilities is something to look forward to.
-
In the future, everyone will have a powerful personal intelligent agent that matches their abilities and fields, serving as a personal assistant for daily work and life, which will be a visible model.
It is suggested that industries like finance should not focus on how to develop their own models at this moment, but rather on how to build their own intelligent agents.
-
Where might the first wave of AI be able to generate profits? We have briefly sorted out the top 20 applications... but in terms of activity and traffic, they are mainly concentrated in the following directions: AI assistants, AI search, and AI video.
-
Returning to the models themselves, in the future, open source and closed source will definitely coexist.
9. Large companies need to return to basic logic: greater computing power and more quality data.
10. Whether the capital expenditure of Chinese operators will end the downward cycle early due to changes in AI is something to observe closely in the next two months.
- Although the capital expenditure of Chinese internet companies has nearly doubled compared to the past, it still lags behind North America when adjusted for exchange rates. In the long term, there is still significant growth potential for the capital expenditure of Chinese cloud providers. The following is a summary of key content organized by the investment workbook class representative (WeChat ID: touzizuoyeben), shared with everyone:
Two Clear Directions for 2025
The deep integration of artificial intelligence with the medical industry, commercial retail industry, and various traditional manufacturing sectors is becoming evident. Today, we focus on the theme of the TMT industry strategy report for the entire year of 2025, which will be released at the end of 2024.
The report is divided into two parts: one is "Domestic Computing Power is Ready," and the other is "AI Applications Take Root."
Despite many new changes during the Spring Festival, our forward-looking views for 2025 have not changed; we still clearly favor two directions.
First, domestic computing power is one of the directions we are most optimistic about for 2025. The democratization of large models and the localized deployment of models have made the computing power sector, especially domestic computing power, the most certain direction for upstream investment, which we will discuss further later.
The other part is "AI Applications Take Root." Looking back at our strategic outlook for 2024 in 2023, we described the application trend at that time as "AI Applications Blooming," which was more thematic or sentiment-driven investment. This year, we use "Take Root" to express the view that applications are gradually moving towards substantial implementation.
Although the manifestation of revenue and profit may still take time, we have already begun to see real changes in download volumes, daily and monthly active users, and real usage scenarios, with more industry fundamental data available for tracking.
Next, I would like to take about half an hour to share our latest views from the three basic elements of the development of large models or the artificial intelligence industry—algorithms, computing power, and applications.
Three Stages of Large Models
First, let's return to the models themselves. Whether it was the breakout of ChatGPT at the end of 2022 or the breakout of DeepSeek during this year's Spring Festival, we have seen that models have been in a rapid iteration process over the past two years.
The first stage is what we call language models, or large language models (LLM). This generation of models, represented by ChatGPT, allows artificial intelligence to "hear and understand" like humans, while also excelling in text generation. The corresponding application scenarios mainly include customer service and text generation.
The second stage is represented by GPT-4 (first half of 2023) and LLama2 (July 2023). Models in this stage enable machines to learn to use tools like humans, such as agents. This stage is the core transformation period for large models in 2023, with application scenarios including programming and AI terminals.
The third stage is the so-called "slow thinking" or reasoning models. After pre-training in the first two stages, models are trained with ultra-large computing power and ultra-large data, enabling machines to possess language and tool usage capabilities In the third phase, taking OpenAI's o1 model and this year's DeepSeek model as examples, the training focus has shifted from the training side to the inference side. This not only significantly reduces training costs but also greatly enhances the model's logical and reasoning capabilities.
If everyone has recently used the DeepSeek model, they will clearly feel that compared to the previous two generations of models, it resembles a personal assistant more, providing logical and deep thinking abilities.
By the time of the third-generation model, its application scenarios are no longer limited to customer service and assistant agents, but extend to more serious scenarios, such as humanoid robots, autonomous driving, and more B-end production scenarios.
However, despite the fact that OpenAI's o1 model appeared as early as 2024, why does the emergence of DeepSeek still attract significant attention from the industry?
The essential difference lies in open source versus closed source. After the open-source release of the DeepSeek R1 model, its capability gains can be replicated, for example, through reinforcement learning and other methods. Open source not only reduces costs but, more importantly, enhances interpretability and traceability, making applications in more serious scenarios possible.
After the Spring Festival, major companies like Tencent and Alibaba quickly absorbed and aligned with the capabilities of DeepSeek, which reflects the changes occurring in the industry. Open source, in addition to being free and low-cost, also promotes localized deployment. In the future, model innovation will not stop and may even surpass the average human level in areas such as predictive capabilities and emotional intelligence.
By then, the application of AI in serious scenarios such as scientific research innovation, medical innovation, and industrial manufacturing will be more widespread, and this day may come faster than we imagine.
It can be seen that the iterations of models over the past two years are just the beginning. In the next ten or even twenty years, models may undergo more version updates, and their capability enhancements and impacts on application scenarios need to be viewed dynamically.
Taking large models as an analogy to operating systems in the mobile internet era, Android and iOS have undergone nearly 20 iterations over the past 20 years. Similarly, large models will continue to evolve. The emergence of DeepSeek lowers the cost for small and medium-sized enterprises to conduct vertical model training while intensifying competition among large companies in foundational model pre-training.
Large companies need larger model scales and data volumes to improve model performance, and this competition will become more intense. Therefore, the coexistence of model equity and competition among giants is an interesting phenomenon in the current industry.
Regarding the source of DeepSeek's capabilities, the V3 version launched in December 2024 achieved low-cost training through engineering innovations, such as using sparse MoE (Mixture of Experts architecture), 8-bit floating-point precision, and multi-token prediction technologies, benchmarking against the capabilities of the GPT-o1 model.
The R1 model further enhances learning on this basis, optimizing logical and language mixing issues, making it closer to human speaking logic and analytical frameworks. This reflects the combination of foundational pre-training and engineering innovation, akin to the mutual promotion of internal skill training and technical practice In fact, we have mentioned before that foundational pre-training is like practicing internal skills, while engineering innovation is akin to practicing techniques. The two often proceed in an intertwined manner. Once internal skills are mastered, techniques need to be practiced, and once the capabilities of techniques are widely mastered, there is a need to return to the cultivation of internal skills. The importance of foundational pre-training always exists.
DeepSeek will not lead to a decrease in computing power demand; Scaling Law remains effective
After the emergence of DeepSeek, there were concerns about the demand for computing power, fearing that the rapid decline in model training costs would lead to a decrease in computing power demand. However, our judgment is that the Scaling Law remains effective, and the demand for computing power will still return to foundational pre-training.
Whether for training or inference, the certainty of computing power remains very high. This year, the structure of computing power demand has gradually shifted from being primarily training-focused last year to increasing inference demand. However, the overall certainty of computing power has not declined, which is the basic framework we are concerned about.
Let’s elaborate a bit on why the Scaling Law remains effective. Current training is basically divided into three parts: pre-training, post-training, and the reinvestment of computing power during the inference phase.
These three parts address the issues of internal skills, techniques, and integration, forming a spiral demand iteration, which is also the reason why the Scaling Law remains effective. More powerful foundational models are crucial for innovations in inference patterns, which is our basic judgment on computing power.
AI equity sparks heated discussions; open source and closed source will coexist in the future
The second issue is that after the emergence of the R1 model, "AI equity" has become a hot topic of discussion. What is equity? It means that everyone can access good models.
In the past, we could not use leading overseas models, and domestic models also had issues such as high costs and hallucinations. However, this year, the foundational model capabilities available to everyone have at least reached a benchmark starting from the R1 generation. For example, Tencent's products like Yuanbao and Qianwen can basically achieve similar effects.
Our views on large models can be divided into two aspects. On one hand, global major companies will quickly integrate services similar to Alibaba Cloud. This will also impact OpenAI's own strategy. For instance, during the Spring Festival, OpenAI launched o3-mini, which, although slightly more expensive than DeepSeek, has significantly reduced costs.
In the future, similar model products will continue to emerge. On the other hand, discussions about open source and closed source will persist. In the mobile internet era, Apple's iOS is a successful example of closed source, while Google's Android is a successful representative of open source. Today, returning to the models themselves, open source and closed source will definitely coexist in the future.
Of course, the source and iteration of capability innovation are the core. Global major companies will increase capital expenditures more significantly in order to achieve stronger capability iterations in foundational models or pre-training But what is the biggest problem with pre-training? While the foundational model is important, how do we create a gap? There are several key factors: first, training models with larger parameter scales, which makes the algorithms more complex; second, the scale of computing power, such as NVIDIA's 100,000-card cluster, which has recently even reached a 200,000-card cluster. The larger the cluster scale, the more it can support models with larger parameters, which brings us back to the issue of single-card capability.
Currently, the gap in single-card capability between domestic chips and NVIDIA is not significant, but if we want to solve the cluster issues of 100,000 cards or even 200,000 cards, the GPU itself remains a significant constraint.
In addition, high-quality data is also key. There are claims that by 2026, all internet data in human history will be learned by AI. At least DeepSeek has conducted what is called "distillation," aligning its own data.
Big companies need to return to basic logic: greater computing power and more high-quality data
So, where will the next capability gains come from? Data, especially high-quality data, remains the key to competition among big companies. To extend this further, where does high-quality data come from? Is there a need for more users, better scenarios, and application entry points?
This is also why those who are recently working on models are starting to think more about application issues. The continuous influx of users and application scenarios that bring high-quality new data is the fundamental source of gains for the next generation of models. From this perspective, big companies need to return to basic logic: greater computing power and more high-quality data. In the next generation of models, if anyone can surpass DeepSeek, it may need to be analyzed from this framework.
DeepSeek "activates" the AI industry chain, and some vertical applications are expected to stand out
On the other hand, open-source models have sparked a global replication craze. Various vertical models or small models combined with reinforcement learning can also achieve good results. For small and medium-sized enterprises, financial institutions, and state-owned enterprises, if they have absolute data or user advantages in vertical scenarios, combining open-source models for localized deployment and quickly integrating their own data for application scenario testing or training will become a trend.
In this environment, some vertical applications are expected to stand out, but it will take some time. The emergence of DeepSeek and the trend of low cost and high performance are best described as "activating" the entire AI industry chain. It has accelerated the speed of industrial circulation, innovation iteration, and application progress.
Therefore, using "activation" to describe DeepSeek's impact on the industry is quite appropriate. It will certainly make the entire industry operate faster.
Investment in four areas: computing power, applications, edge, and data
Next, let's elaborate a bit. Investment can generally be divided into four parts: first, the impact on computing power itself; second, the impact on applications; third, the impact on the edge; and fourth, the impact on data. The basic framework can be viewed in these four areas.
First, let's talk about computing power. We will find that everyone is genuinely starting to increase capital expenditures, which is reflected in the fundamentals, primarily in the computing power sector. Of course, computing power is not just about GPUs; it also includes the entire industry chain, such as the recently high-profile servers, data centers, and related support within data centers The second major area is applications. In terms of applications, North America and China are slightly different, which we will elaborate on later.
The third area is the endpoint. The determinism of hardware is, on one hand, upstream, where the computing power on the server side is a strategic infrastructure. On the other hand, on the application side, although many software aspects are still difficult to grasp, such as in advertising and video fields, terminal hardware, such as smartphones, cars, and smart homes, remains an unavoidable data entry point. It may not have the most advanced models, but it certainly has the freshest data and the most timely application scenarios. Therefore, the endpoint remains a direction with relatively high certainty this year.
Another aspect that is easily overlooked is data. As we mentioned earlier, the future innovation capability of foundational models mainly comes from the data itself, especially B-end data, which has proprietary and unique characteristics. This is also an important investment direction.
This is just an intermediate state; future overseas next-generation model capabilities will definitely gain enhancement.
Looking at the overseas o3 model, starting from the o1 model, its development path is quite similar to DeepSeek, both showing better performance on the inference or logic side. However, overall, this is just an intermediate state, and future overseas next-generation models will definitely gain enhancement in capability.
What needs to be discussed is whether they will take the open-source or closed-source path, which could be a significant influencing factor. Clearly, this is not the endgame yet.
The era of Agents is coming.
Additionally, agents are also a concept we mentioned frequently last year, and we still have high hopes for this direction in 2025.
What is the core difference between agents and a single powerful model? If we compare general artificial intelligence or a powerful foundational model to a person, then an agent is like an organization, such as a unit, group, or team.
We have recently seen that in many companies' localized deployments, they do not just choose one model; I can choose five models, and I will take whichever model is better. Besides different models, more importantly, is the data, including structured data, unstructured data, and internal business processes. The combination of these two can better address business needs.
We suggest that industries like finance should not currently consider how to develop their own models, but rather how to build their own agents.
In this process, the advantages of data and the effects of application loops will be better. Recently, we have also seen some third-party software that allows everyone to build their own agents based on personal knowledge bases or databases, ultimately becoming personal assistants.
In the future, everyone will have a powerful personal agent that matches their abilities and fields, serving as a private assistant for daily work and life, which will be a visible model.
Hong Kong stock technology stocks and foreign capital are very focused on Chinese technology assets, and the reason lies here.
This table is very important as it shows the global access volume of large models.
There is no doubt that GPT remains in first place, and DeepSeek has entered the top three. The yellow section represents domestic models, and overall, China and the U.S. each account for half. This also indirectly confirms why foreign capital is very concerned about Chinese technology assets in the Hong Kong stock market. From the results, the gap between us and overseas models in terms of traffic and capability is narrowing.
Three Major Changes in Global Models
Another noteworthy point is the significant increase in usability brought about by the decrease in model prices. The leveling of models or the drop in prices has activated the multiplier effect across the entire industry, leading to more and more people starting to deploy applications.
I often give an example: the 3G network was commercially available in 2009, but mobile games, as the first profitable application of the mobile internet, did not emerge until 2013, taking four to five years in between. The reason was not that the network was inadequate or that the phones were lacking, but because the data was too expensive. In that era, it was impossible to play games or watch videos using expensive data. Therefore, the decrease in costs is the core key point that enables applications to thrive, equally important as the model's own capabilities.
To summarize, the changes in global models are mainly reflected in the following aspects:
First, starting from 2025, there will be a true transition from research and development to production environments, meaning that everyone will be able to genuinely use them, and work scenarios can be integrated, making innovation more active.
Second, the technical routes and capabilities of large models are rapidly iterating, with a crossover of open-source and closed-source leading.
Third, the gap between domestic models is narrowing, usability has significantly improved, and the trend of model leveling is very evident.
Computing Power Beta Still Exists, and the Proportion of Inference Demand Will Be Greater
Based on these judgments, I would like to share some specific directions we are optimistic about in terms of investment.
This can actually be divided into two parts: one is computing power, and the other is applications.
Overall, the beta of computing power still exists, meaning that the demand for computing power has not ended, and there is still distance from bubble formation. However, structurally, the proportion of inference demand will be greater.
In the past two years, we mainly saw training demand, but the overall trend is shifting from training to inference, with inference becoming the focus. Of course, everyone will also pay attention to the essential differences between training and inference, which I will elaborate on later.
Many People Have Shifted from "Impossible to Deploy" to "Possible to Deploy"
First, let's return to the essence of computing power. What exactly is computing power? Opening the server room, GPUs are undoubtedly the core of the entire industry. We need to deploy GPUs onto servers, and the cost of localized deployment has significantly decreased.
For example, DeepSeek's 70B model requires just one server, with costs around one to two million. Last year, the cost of localized deployment was at least in the tens of millions, but this year it has dropped to the million level. This is a qualitative difference. Many people have shifted from "impossible deployment" to "possible deployment." However, there are still not enough servers; a complete data center environment is needed, including copper connections, liquid cooling, power supply, etc., and then placed into the data center (IDC). Of course, the data center also requires supporting equipment such as optical modules and PCBs, ultimately forming the foundational environment for computing power.
Therefore, in addition to the GPU itself (the most direct or important link), the rise of localized deployment and applications, along with the subsequent demand for cloud computing and the overall deployment needs, will also drive the development of the entire industry. This is the trend we have recently observed.
Chinese cloud vendors still have significant capital expenditure space, with a gap compared to North America due to exchange rates
Looking at capital expenditure, this is also a major focus for everyone. Let's examine the capital expenditure situation of overseas cloud service providers (CSPs). It can be observed that Q1 2023 marks a very clear turning point.
After OpenAI launched ChatGPT at the end of 2022, starting in 2023, the capital expenditure of cloud vendors has been rising each quarter. It is expected that by 2025, the capital expenditure of North American cloud vendors will be around $300 billion to $400 billion.
Looking domestically, Alibaba's capital expenditure has grown rapidly, with both the total over the past decade and the annual average at least doubling.
More importantly, in addition to Alibaba, the capital expenditures of internet companies such as Tencent, ByteDance, Baidu, and Meituan are also increasing.
From the turning point perspective, the overall data for Q1 2023 indicates that the capital expenditure of Chinese internet companies is expected to be around 300 billion to 400 billion yuan. Although this has nearly doubled compared to the past, it still falls short of North America by an exchange rate.
During the mobile internet era, I reviewed cloud computing data in 2015. At that time, the capital expenditure of Chinese cloud vendors was about 70% of that of North America. Now, we are only about one-seventh of North America.
In the long term, there is still significant growth potential for the capital expenditure of Chinese cloud vendors.
In the next two months, pay attention to whether the capital expenditure of Chinese operators will end the downward cycle early due to AI changes
Looking at operators, the capital expenditure of Chinese operators has always been substantial. In the past, the three major operators had an annual capital expenditure of about 400 billion yuan. However, this year, there is a very interesting phenomenon: the capital expenditure of Chinese cloud vendors and operators may reach a balance, both around 300 billion yuan.
This phenomenon appeared in the U.S. in 2018 when the capital expenditure of internet companies surpassed that of traditional operators. Since then, the two have formed an intersection.
Whether the capital expenditure of Chinese operators will end the downward cycle early due to AI changes is something to closely observe in the next two months.
Because the capital expenditure of operators is itself in a downward cycle, if it ends early, then the capital expenditure of cloud vendors and operators will become an important source of upstream capital expenditure for computing power. This is also the source of certainty for subsequent investment opportunities.
Shipping volume and ecosystem construction are key challenges, focus on these when selecting targets
Returning to domestic computing power, computing power itself is a beta, while domestic computing power is an alpha, meaning there is marginal increment.
Our team once created a table analyzing several key points of domestic GPUs: first is shipping volume, i.e., production capacity; second is the ecosystem, similar to NVIDIA's CUDA or the X86 of yesteryear, whether it can operate within the ecosystem; finally, product strength. Currently, the single-card capability of domestic GPUs is not inferior to NVIDIA products, but the upcoming key challenges lie in tape-out (shipping volume) and ecosystem construction. This is what everyone needs to focus on when choosing investment targets.
Transitioning from training era to inference era, these manufacturers will have more opportunities
As we mentioned earlier, with training demand gradually shifting to inference demand, the requirements for single-card computing power in inference cards are no longer the only key indicator. Storage capacity, communication capability, and cluster capability may be more important.
Compared to the training era, the inference era may present more opportunities for domestic cards, startups, and dedicated inference card manufacturers, including ASICs, etc.
This is a change in the supply side of training versus inference that everyone needs to pay attention to.
Therefore, the friendliness and adaptation efficiency of domestic cards in the inference era may be higher, which is our judgment.
Edge computing power is very important; if the cloud side is likened to a large supermarket, the edge side is the small shop at the entrance
Looking at the edge side. If we compare the cloud side or server side to a large supermarket, then the edge side is the small shop at the entrance.
Edge computing power is very important; mainstream global PC manufacturers, mobile phone manufacturers, and even robot manufacturers are rapidly enhancing edge computing power. The same goes for automobiles. We believe that this year, mobile phones remain a promising direction.
Will the terminal bring about a new innovation cycle due to the equalization of intelligent capabilities? This is worth looking forward to
Recently, there has been much discussion about whether a very powerful AI application will soon emerge, and what kind of APP or software it will be.
Our team has also discussed this, and what is relatively certain is that intelligence itself is an application, just like when the internet first emerged, the internet itself was an application.
Intelligence needs a carrier, and terminals and hardware carriers are currently indispensable. For example, Apple, although it has not developed a powerful large model, can choose to cooperate with OpenAI (overseas) or with Alibaba (domestically). Whether terminals will bring about a new innovation cycle due to the equalization of intelligent capabilities is very much worth looking forward to, which is our judgment on the terminal side.
Four major changes in computing power, domestic computing power is the most certain investment direction this year
To summarize briefly, the changes in computing power mainly include the following points:
First, overall demand remains strong, and the proportion of inference demand will gradually increase, but this does not mean that training is unimportant; these two need to be viewed separately;
Second, the integration of storage and computing, and the deep fusion of software and hardware algorithms is an important technological trend, and DeepSeek has also made similar innovations; Third, edge computing power is still worth looking forward to;
Fourth, domestic production is accelerating. We believe that domestic computing power is the most certain investment direction this year.
What are the potential directions for the first wave of AI to make money?
Finally, let me quickly talk about applications in two minutes.
From an overseas perspective, in the 2 to 3 quarters following the release of GPT, AI applications began to flourish in various fields, including office, video, advertising, and e-commerce, all showing good performance. At that time, some B-end applications in North America, such as AppLovin, Shopify, and Palantir, exceeded expectations in performance.
For us, we need to benchmark against overseas but cannot be rigid in our approach, as the SaaS business model in China differs from that abroad. This at least gives us a thought: Where might the first wave of AI be able to make money? We have briefly sorted out the top 20 applications.
Overall, the rankings fluctuate quickly, similar to the game rankings in 2013, changing weekly. However, in terms of activity and traffic, they are mainly concentrated in the following directions: AI assistants, AI search, and AI video. They perform well on the consumer side.
Overall, B-end commercialization is progressing faster, with more obvious performance results; the C-end is reflected in traffic growth and user numbers, with performance realization being relatively slower.
The most promising application is AI Agent, followed by edge AI, and lastly robots
Next, I have selected several relatively promising application directions in China for the near future.
First is the AI Agent, which aligns well with China's characteristics. Whether for enterprises or individuals, the most valuable aspect is to consolidate capabilities.
In the context of model convergence, how to differentiate applications? Building one's own AI agent is very important. Models will continue to iterate, and in the future, there may be multiple models, but unique data, knowledge bases, and knowledge graphs are the core value of enterprises. Therefore, the AI agent is the direction we are most optimistic about in applications this year.
But the question arises, who will create the AI agent? We need to follow up further.
Currently, we see that hardware manufacturers like Huawei, Xiaomi, and Apple want to create AI agents. Some large model manufacturers that find it difficult to compete in foundational models will also turn to vertical AI agents. Some internet companies are also developing in this direction. For example, Tencent's Yuanbao currently integrates DeepSeek and may introduce other models in the future.
In the competition for AI agents, who can stand out still needs further assessment.
The second direction we are optimistic about is edge AI. We have listed emerging devices like glasses, but overall, the largest scenario for edge computing remains mobile phones, followed by cars, PCs, as well as glasses, toys, etc. The hardware carrier has strong stickiness to scenarios and data. In terms of investment, if it is difficult to select whole machine companies, one can focus on upstream areas such as storage, components, or parts The third is the recently popular robot, also known as JuSheng Intelligent. With the improvement of model logic capabilities, the market space for robots and autonomous driving is the largest and most promising. However, progress may not be as fast as the previous directions, but the potential is significant.
Four Investment Directions
On the last page, a brief summary. These targets do not represent recommendations; they are just some directions we have listed, and they are not exhaustive.
Large models remain the core driver of industrial innovation. From an investment perspective, we are most optimistic about four directions:
First and foremost is computing power, including GPU, servers, optical communication, and other fields;
Of course, computing power itself, as we just discussed about domestic computing power, may be beyond just GPUs. However, I think it can be slightly extended upwards, such as in advanced process foundries, even semiconductor equipment, and related data center PCBs and storage.
The further upstream you go, the more concentrated it becomes. While it may not have as much elasticity, its certainty is extremely high, and selecting targets becomes easier. Because the entire upstream is relatively heavy asset-based and has gone through significant filtering over the past few years.
It will not become more numerous like downstream companies but will become fewer. This is what we see as the main targets in the upstream sector.
Second is AI hardware devices, such as mobile phones, PCs, speakers, etc.;
Third is data layer-related services;
Fourth is the application side, with many companies listed. The reason for listing many is that we are unsure what to choose; overall, it may change relatively quickly, and there should be some interesting new companies emerging in each module.
A simple summary is that on the application side, from an overseas perspective, B-end applications have already begun to have very good implementation at the reporting level. The C-end is still seeing a continuous increase in application traffic. Specific directions include AI Agents, AI terminals, JuSheng Intelligent, and autonomous driving.
This year is actually more like a true 2013
Finally, to say one more thing, overall, for the past two years, we have been discussing the rise of artificial intelligence, and now we are entering whether it is in a bubble stage.
I used a chart to review the mobile internet era, and last year had a similar viewpoint. This year is actually more like a true 2013, hardware has been laid out for a long time, penetration rates are starting to increase, and reliable operating systems have emerged, leading to the appearance of today's large models.
However, for the exploration of applications, the future is the main focus of the entire industry. For the entire industry to ultimately be considered a mainstream industry, truly landing as productive force, the value of applications must far exceed infrastructure investment. In the era of mobile internet, the ratio of infrastructure investment to application output value is approximately 1:7. But now looking at AI, if you invest one dollar, the output value is about seventy cents, less than one-tenth.
So, this matter may just be beginning. Importantly, during the active period of new technological transformation, we must continuously iterate our research framework and validate investment targets. At least, this matter should be viewed in dimensions of five years or ten years.
Source: Investment Workbook Pro Author: Wang Li
For more insights from industry leaders, please follow↓↓↓
Risk Warning and Disclaimer
The market has risks, and investment requires caution. This article does not constitute personal investment advice and does not take into account the specific investment goals, financial conditions, or needs of individual users. Users should consider whether any opinions, views, or conclusions in this article are suitable for their specific circumstances. Investing based on this is at your own risk