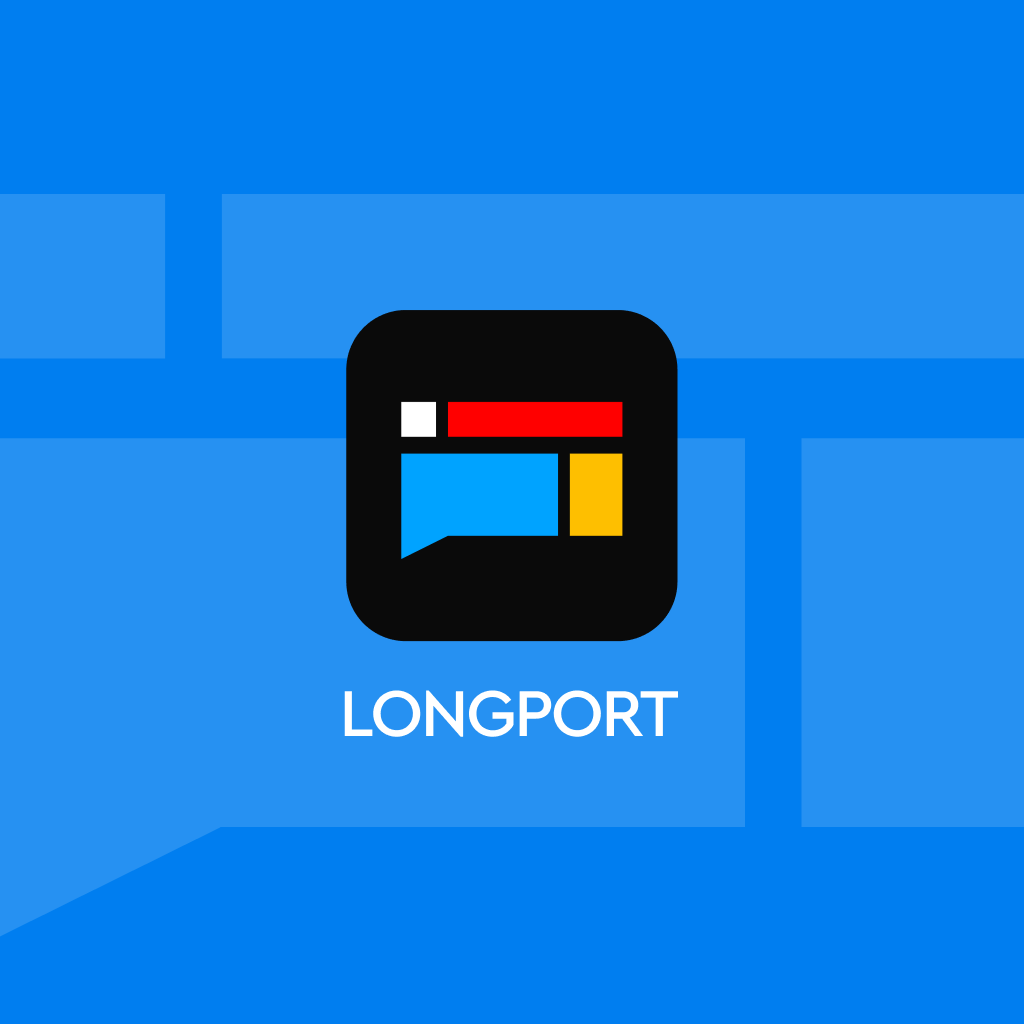
Is the "all-weather" strategy applicable in China?
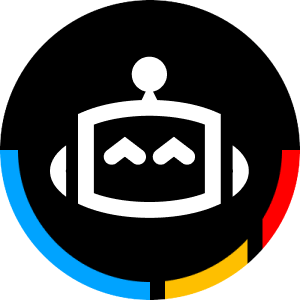
"All-Weather Strategy" is an asset allocation strategy aimed at maintaining stable returns for a portfolio under different economic conditions through diversification and dynamic adjustments. Its foundation is the concept of "risk parity," which emphasizes the balanced distribution of risk across various asset classes to reduce volatility and enhance returns. This strategy has proven effective in practice, especially in dealing with market uncertainties
Abstract
First, the "All Weather" strategy is a well-known asset allocation strategy first proposed by Bridgewater in 1996. Its main idea is not to rely on forward-looking predictions and timing, but to attempt to create a portfolio that can traverse cycles and be applicable under different economic conditions through diversified asset allocation and dynamic adjustments, thereby achieving relatively stable returns.
Second, before understanding the "All Weather" strategy, we need to explain a concept called "risk parity." The logical starting point of asset allocation revolves around "risk." Harry Markowitz proposed Modern Portfolio Theory (MPT) in 1952, with its core being the mean-variance model (MVO), which describes asset characteristics using two dimensions: return (mean) and risk (variance). From this framework, it is straightforward; asset allocation seeks to maximize returns per unit of risk, i.e., the optimal Sharpe ratio. However, the mean-variance model (MVO) does not perform well in practice due to three major flaws: it requires subjective input for expected returns, relies too heavily on historical data, and is highly sensitive to parameters. Based on these flaws, two improvement paths have evolved: one is the Black-Litterman model, which better combines subjective views and quantitative models through Bayesian theory to optimize estimates of expected asset returns; the other includes models like "risk parity," minimum variance models, and maximum diversification models, which abandon estimates of expected asset returns and shift to allocation based on "risk," which is less sensitive to parameters.
Third, risk parity is a framework based on "risk." Its core idea is to abandon the difficult-to-predict return benchmarks and only consider "parity" of risk, meaning that risk is evenly distributed across various asset classes, ensuring that each asset class contributes equally to the portfolio's volatility. Therefore, it is a "risk balancing strategy." Theoretically, risk parity reduces volatility by diversifying risk while increasing returns through leverage, thereby improving the Sharpe ratio. This strategy has also been proven effective in real-world operations. For example, using the simplest asset allocation model of a 60/40 stock-bond mix, applying risk parity and leverage can yield a portfolio with similar returns but lower volatility and a better risk-return ratio. In Qian's (2005) examination using data from 1983-2004, a stock-bond 77/23 portfolio with the same total volatility contribution had a Sharpe ratio far superior to that of the 60/40 stock-bond portfolio (0.87 > 0.67), and it was also higher than any single asset. After adding leverage, the risk parity portfolio maintained the same Sharpe ratio of 0.87 while achieving an annualized return of 8.4%, surpassing the 8.3% return rate of a pure stock index Fourth, the "All-Weather Strategy" is essentially a model that utilizes the concept of risk parity, but the two are not entirely equivalent. Risk parity is a mathematical model that balances risk without considering macroeconomic factors; it merely addresses issues such as parameter sensitivity and extreme positions compared to the mean-variance optimization (MVO) model. The "All-Weather" strategy resembles a macro strategy model that still starts from the macroeconomic cycle, but it does not attempt to identify and predict cycles; instead, it uses the concept of risk parity to avoid losses caused by misjudging the cycle. The steps of the All-Weather Strategy can be summarized as "two cores, four steps." The two cores are "risk parity + dynamic adjustment," and the four steps are "define states, select assets, introduce leverage for equal risk weighting, and dynamic rebalancing." "Defining states" refers to the model's assumption that asset volatility arises from unexpected growth or inflation, with four states being above-expectation growth, below-expectation growth, above-expectation inflation, and below-expectation inflation. "Selecting assets" means identifying superior assets in each of the four states, with each quadrant allocated 25% of the risk weight. With the introduction of leverage and equal risk weighting, different assets' risk-return characteristics are aligned through leverage. Finally, "dynamic risk rebalancing" refers to adjusting asset weights in the next period by recalculating the volatility and correlation of each asset to maintain risk parity.
Fifth, reviewing the historical performance patterns of the "All-Weather Strategy." Dalio, R (2015) demonstrated that during the period of declining interest rates from 1981 to 2015, the All-Weather Strategy achieved an annualized return of 12.1%. Since 2015, there have been few public details about this strategy, but we can obtain performance results from overseas ETF websites (lazyportfolioetf.com) that have traceable data for a longer period (approximately 154 years since 1871). The lazyportfolioetf website shows that its compound returns over the past thirty years and ten years are 7.68% and 4.46%, respectively; volatility has increased from 7.48% to 8.45%, and the Sharpe ratio has decreased from 0.72 to 0.33. The internal composition shows that U.S. Treasury ETFs have been a drag, while U.S. stocks and gold have supported returns in recent years. Based on Dalio's empirical allocation ratios (30% U.S. stock exposure, 40% long-term U.S. Treasury, 15% medium-term U.S. Treasury, and 7.5% each for gold and other commodities), simulations indicate that the compound annual return of this portfolio over the past ten years is approximately 4.18%, which is roughly comparable to the lazyportfolioetf data. Both methods indicate that the maximum drawdown over the past thirty years occurred in 2022, with an estimated maximum drawdown of 23.8%, while the lazyportfolioetf website reports 20.58%, which is also roughly comparable. In simple terms, its effectiveness over the past ten years has significantly declined, but its effectiveness over a longer dimension remains high.
Sixth, further reviewing the historical performance patterns of broadly defined "risk parity-type funds." Observations of PanAgora Risk Parity, the S&P Risk Parity Index, etc., similarly reveal that their "golden age" began after the 2008 financial crisis, faced skepticism from 2013 to 2015 (underperforming the US 60/40 portfolio), and rebounded somewhat in 2016-2017 After 2018, it returned to a state of calm. The annual excess returns of the S&P 500 Risk Parity Index from 2009 to 2012 (compared to the US 60/40 portfolio) were 3.77%, 15.27%, 8.91%, and 5.86%, totaling 33.81%. In 2013, under expectations of tightening overseas monetary policy, the Risk Parity Index underperformed the US 60/40 portfolio by nearly 12 percentage points. From 2016 to 2017, with interest rates gently declining, the corresponding S&P 500 Risk Parity Index rebounded, recording annualized returns of 13.81% and 10.81%. After 2018, the pandemic shock and the Federal Reserve's restart of a new round of interest rate hikes made risk parity less advantageous. In summary, since risk parity relies on the premise of positive long-term asset returns, when all assets experience significant increases in volatility due to exogenous shocks or when interest rates rise unexpectedly, simple risk parity strategies are relatively fragile. Therefore, risk parity strategies are not a "one-size-fits-all" or "set it and forget it" approach; they still require suitable macro and market conditions.
Seventh, let’s further examine the domestic macroeconomic environment and financial market conditions, where the applicability of risk parity strategies seems to be increasing. First, as China's economy enters a new stage of high-quality development, the elasticity of economic growth, inflation, and monetary cycles has become smoother, and the past attributes of interest rate "cyclical goods" have diminished, leading to a convergence in the volatility of risk and risk-free assets; second, with the continuous development of domestic financial markets, there has been significant progress in asset selection diversification and financial instrument variety, which is conducive to the application of risk parity and all-weather strategies; third, with the rise in global macroeconomic uncertainty and changes in the characteristics of the wealth management market, there is a greater demand for investment to diversify risks (placing more emphasis on the "Sharpe ratio"), making the "volatility reduction" function of risk parity attractive.
Eighth, we have made preliminary attempts to build a parity model. We conducted tests on three sets of basic global asset indices (six categories), basic ETFs (seven categories), and extended ETFs (eleven categories). Consistent with the MVO test results, since 2015, the best risk-return ratio has been in Chinese bonds (especially credit bonds), followed by gold, US stocks, and then dividend assets, CSI 300, and the Hang Seng Index. If we further narrow it down to since 2021, gold ranks best, with dividends following closely. The variance-covariance matrix of returns between assets is a core input parameter for the risk parity model. Whether looking at long-term or rolling correlations, Chinese assets not only show a negative correlation between stocks and bonds, but also a negative correlation between bonds and Hong Kong stocks with Chinese bonds; dividend assets, which have some "bond-like" characteristics, generally maintain a positive correlation with the broader market, although this positive correlation has significantly weakened after 2020. Chinese concept internet stocks have a strong and stable positive correlation with the domestic CSI 300 ETF; the China REITs total return index has not shown high correlation with pure stock indices or pure government bonds since 2024 Ninth, from the backtesting results, the risk parity model has a higher Sharpe ratio, win rate, and payout compared to the simple equal-weight and simple volatility inverse-weighted models, with this advantage becoming more pronounced after 2022. Since 2009, the Sharpe ratio, win rate, and payout of the risk parity scheme for major asset classes have been 1.79, 58.3%, and 1.06 (profit-loss ratio), respectively, which is higher than the equal-weight scheme's 0.62, 53.0%, and 1.03; from 2022 to February 2025, the Sharpe ratio, win rate, and payout of the risk parity scheme for major asset classes have been 3.37, 62.1%, and 1.35, while the corresponding equal-weight scheme has been 1.07, 51.4%, and 1.17, and the volatility-weighted scheme has been 2.47, 58.9%, and 1.24. During the same period, the risk parity scheme for the basic ETF asset pool has been 2.04, 60.6%, and 1.05, while the equal-weight scheme has been 1.51, 54.8%, and 1.08; the risk parity scheme for the expanded ETF asset pool has been 0.89, 57.4%, and 0.99, while the equal-weight scheme has been 0.88, 52.6%, and 1.07. The years when the strategy performed relatively poorly were 2011, 2013, 2018, and 2022. In domestic testing, the historical scenarios unfavorable to risk parity include multi-asset linked corrections (most typically, simultaneous declines in stocks and bonds, or stocks and commodities) and excessive volatility in a particular asset. In terms of weights, the relatively stable net value of the unleveraged basic risk parity still benefits from the relative overweight in bond assets. Compared to the proportions given by Dalio, the total proportion of bond assets in domestic risk parity reaches 74.4%, making it a more dominant and core underlying asset.
Tenth, we further introduce leverage. As mentioned earlier, while the unleveraged risk parity portfolio has a high Sharpe ratio, its returns are not attractive. There are two ways to introduce leverage: one is to leverage the assets first and then apply parity; the other is to apply parity first and then leverage the portfolio. The former is the Bridgewater approach, while the latter is simpler and more direct, which is to directly leverage the portfolio after parity by obtaining funds from the capital market (pledging bond assets for repurchase) to continue buying the parity portfolio. The cost of funds is related to the market financing cost, which is more closely correlated with short-term interest rates. This paper adopts a method of leveraging and considers the domestic situation, limiting leverage to the range of 80% to 140%. We tested the effects with target volatilities of 2%, 3%, 4%, 5%, and 6%. In the three asset pools, the annualized return and annualized volatility of the portfolio increased with the target volatility, while the Sharpe ratio slightly declined. Under the basic ETF risk parity scheme, since 2015, the annualized return of the portfolio increased from 5.1% to 6.4% as the target volatility rose from no target to a target volatility of 6%, and the annualized volatility increased from 2.9% to 4.8%, with the Sharpe ratio decreasing from 1.07 to 0.92. If we further shorten the period to since 2022, as the target volatility increases, the annualized return of the portfolio rises from 6.0% to 8.3%, the annualized volatility increases from 2.0% to 3.3%, while the Sharpe ratio only decreases from 2.04 to 1.93 Eleventh, a brief summary of the re-understanding of the All Weather strategy. Each financial crisis prompts investors to reflect on the importance of risk awareness, making risk management more crucial. The risk parity model and the All Weather strategy are expressions of this investment philosophy. After the 2008 financial crisis, the United States initiated quantitative easing, and the overseas macro environment entered an era of "low interest rates, low inflation, and low growth," known as the "three lows," providing a comfortable growth environment for the "risk parity" strategy. With the post-pandemic inflation center rising, the Federal Reserve raising interest rates, and a significant upward trend in U.S. Treasury yields, the All Weather strategy has also undergone an adjustment period. Coupled with the situation during the Great Depression overseas and the historical testing of basic risk parity in China, we understand that the premise of the "All Weather strategy" is that assets exhibit rotation performance, with long-term returns being positive. In short, this strategy implies the assumptions of "everything has its time" and "when the East is not bright, the West shines." If stocks, bonds, and commodities all perform poorly, the effectiveness of the strategy will significantly decline. From this understanding, in a period where domestic total growth fluctuations gradually stabilize, inflation elasticity decreases, and interest rate centers are not high, the applicability and practicality of the risk parity strategy may increase.
Brief Text
First
The "All Weather strategy" is a well-known asset allocation strategy first proposed by Bridgewater Associates in 1996. Its main idea is not to rely on forward-looking predictions and timing but to attempt to achieve a diversified asset allocation and dynamic adjustment, allowing the investment portfolio to traverse cycles and maintain applicability under different economic conditions, thereby achieving relatively stable returns.
The concept of the "All Weather strategy" originates from the investment return decomposition equation of the Dalio team, where fund returns = risk-free rate + market risk return + active management return, i.e., R = Rf + β + α. By separating β and α, the strategy aims to desensitize the portfolio to the Beta of a single asset through an All Weather approach, obtaining a more robust, long-term stable market β return that traverses cycles, and then enhancing it subjectively to capture α. Therefore, the All Weather strategy is the underlying strategy of Bridgewater Associates, relatively "weakening timing," focusing on diversified allocation, dynamic adjustment, and risk balance, allowing the portfolio to offset risks under different economic conditions, leaving the overall risk premium that grows over time.
Second
Before understanding the "All Weather strategy," we first need to explain a concept: "risk parity." The logical starting point of asset allocation revolves around "risk." Harry Markowitz proposed Modern Portfolio Theory (MPT) in 1952, with its core being the mean-variance model (MVO), which describes asset characteristics using two dimensions: return (mean) and risk (variance). From this framework, it is straightforward; asset allocation seeks to maximize returns per unit of risk, i.e., the optimal Sharpe ratio. However, the Mean-Variance Optimization (MVO) model does not perform well in practice, with three major flaws: it requires subjective input for expected returns, relies too heavily on historical data, and is highly sensitive to parameters. Based on these shortcomings, two improvement paths have evolved: one is the Black-Litterman model, which better combines subjective views and quantitative models through Bayesian theory to optimize estimates of asset expected returns; the other includes models like "Risk Parity," Minimum Variance, and Maximum Diversification, which abandon the estimation of asset expected returns and shift towards allocation based on "risk," which is less sensitive to parameters.
Before Harry Markowitz, the systematic exploration of stock and bond valuation theories primarily relied on discounted cash flow models to understand investment returns, believing that the core of the investment process is to decompose the sources of returns, without giving sufficient attention to risk (or volatility).
The main purpose of asset allocation is to reduce correlation and investment risk through asset diversification, achieving maximum returns per unit of risk. Therefore, regardless of the method used, we hope to maximize the Sharpe ratio of the portfolio through the allocation model.
The core of the Mean-Variance Optimization (MVO) model is "two lines, all points." One is the indifference curve of investor preferences; the other is the efficient frontier representing the relationship between return and risk of the portfolio. Risk-averse investors seek to maximize returns at a given risk level and minimize risk at a given return level. The asset allocation process was first quantified under this model as an "optimization problem" subject to certain constraints.
The drawback of this model is that it is based on historical data and can only represent the past. If future returns and volatility change, or if correlations with other markets and assets change, the aforementioned rules will also change. In fact, this is the core reason why the Mean-Variance Optimization (MVO) model does not perform well in practice: it still requires predicting the future, relies too heavily on the past, and is highly sensitive to parameters (V. Chopra and W. Ziemba, 1993).
Third
Risk Parity is a framework based on "risk." Its core idea is to abandon the difficult-to-predict return benchmarks and only consider "equalizing" risk, meaning that risk is evenly distributed across various asset classes, ensuring that each asset class contributes equally to the portfolio's volatility. Therefore, it is a "Risk Balancing" strategy. Theoretically, Risk Parity reduces volatility by diversifying risk while increasing returns through leverage, thereby improving the Sharpe ratio. This strategy has also been proven effective in real-world operations. For example, using the simplest asset allocation model of a 60/40 stock-bond mix, applying Risk Parity and leverage can yield a portfolio with similar returns but lower volatility and a better risk-return ratio. In Qian's (2005) study using data from 1983-2004, the Sharpe ratio of a stock-bond 77/23 portfolio, which contributes equally to total volatility, was significantly better than that of the 60/40 stock-bond portfolio (0.87 > 0.67), and also higher than any single asset After leveraging, the risk parity portfolio can achieve an annualized return of 8.4% while maintaining the same Sharpe ratio of 0.87, which is higher than the 8.3% return rate of a pure stock index.
We can simply divide the theoretical framework of asset allocation into two strands. One is the "Return Based Allocation Models," such as the constant mix model, mean-variance model (MVO), and the B-L model that incorporates subjective views. The other is the "Risk Based Allocation Models," such as the risk parity model and the upgraded risk budgeting model. After the 2008 financial crisis, many investors realized that traditional allocation models insufficiently depict and focus on "risk."
In contrast, Black-Litterman type models still follow the mean-variance theory, merely inputting more optimized parameters, while risk parity abandons the difficult-to-predict return benchmark and only considers "equalizing" risk to ensure that each asset contributes equally to the overall portfolio's volatility. Theoretically, risk parity reduces volatility through risk diversification while increasing returns through leverage, thereby improving the Sharpe ratio.
In the return-risk coordinate system, the constant 60/40 stock-bond portfolio is located at the upper right end of the boundary, close to an all-equity portfolio. The unleveraged version of the risk parity portfolio significantly reduces returns but achieves a higher Sharpe ratio due to reduced volatility (the slope of the risk parity CML is steeper). The leveraged version of the risk parity portfolio moves along the risk parity CML straight line to the right of the unleveraged version, indicating that after leveraging with a risk-free rate, although volatility increases again, the increase in returns is more pronounced. We can find a combination that, after leveraging, has the same return as the original 60/40 stock-bond portfolio but with lower volatility and a better risk-return ratio.
Dr. Edward Qian discovered in 2005 that the risk of a constant stock-bond portfolio is not balanced. From 1983 to 2004, the Russell 1000 contributed 93% of the portfolio's volatility (variance), while bonds contributed only the remaining 7%. In other words, the portfolio was actually exposed to more volatile equity assets. He allocated 23% of the position to the Russell 1000 index and the remaining 77% equally to the Lehman Aggregate Bond Index to ensure that both contributed equally to the portfolio's volatility. During the period from 1983 to 2004, without considering leverage, the Sharpe ratio of the risk parity portfolio was 0.87, higher than any single asset portfolio, truly achieving higher unit risk returns through diversification. In contrast, the Sharpe ratio of the 60/40 stock-bond portfolio was 0.67, lower than the Sharpe ratio of the pure bond aggregate index at 0.80, and the portfolio's Sharpe ratio was lower than that of some internal assets. Furthermore, under the assumption of a leverage ratio of 1.8:1, the risk parity portfolio achieves an annualized return of 8.4% while maintaining the same Sharpe ratio of 0.87, which is higher than the 8.3% return rate of a pure stock index Fourth
The "All-Weather Strategy" is essentially a model that utilizes the concept of risk parity, but the two are not entirely equivalent. Risk parity is a mathematical model that balances risk without considering macroeconomic factors; it merely addresses issues such as parameter sensitivity and extreme positions compared to the Mean-Variance Optimization (MVO) model. The "All-Weather" strategy resembles a macro strategy model that still starts from the macroeconomic cycle, but it does not attempt to identify and predict cycles. Instead, it uses the concept of risk parity to avoid losses caused by misjudging the cycle. The steps of the All-Weather Strategy can be summarized as "two cores, four steps." The two cores are "risk parity + dynamic adjustment," and the four steps are "defining states, selecting assets, introducing leverage for equal risk weighting, and dynamic rebalancing." "Defining states" refers to the model's assumption that asset volatility arises from unexpected growth and unexpected inflation, with four states being above-expectation growth, below-expectation growth, above-expectation inflation, and below-expectation inflation. "Selecting assets" means identifying superior assets in the four states, with each quadrant allocated 25% of the risk weight. With the introduction of leverage and equal risk weighting, different assets' risk-return characteristics are aligned through leverage. Finally, "dynamic risk rebalancing" refers to recalculating the volatility and correlation of each asset in the next period to adjust asset weights to maintain risk parity.
The similarity between the All-Weather Strategy and risk parity is that neither starts from return predictions; instead, they maintain "the contribution of each asset to the portfolio risk at the same level" through leverage, directly constructing a portfolio that can cope with different risks. The difference is that risk parity is a mathematical model that balances risk without considering macroeconomic factors, merely solving issues of parameter sensitivity and extreme positions compared to MVO.
The All-Weather Strategy, on the other hand, resembles a macro strategy model that still belongs to the macro strategy school based on economic conditions, but it relatively "weakens timing" and advocates for "separating β from α." Unlike the Merrill Clock, the four configuration states of the All-Weather Strategy do not exist in a cyclical rotation over time; they merely objectively divide into four independent configuration scenarios. We summarize the All-Weather Strategy as "two cores, four steps." The two cores are "risk parity + dynamic adjustment," and the four steps are "defining states, selecting assets, introducing leverage for equal risk weighting, and dynamic rebalancing."
"Defining states" refers to the model's assumption that asset volatility arises from unexpected growth and unexpected inflation.
"Selecting assets" means the strategy will identify superior assets in the four states, with each quadrant allocated 25% of the risk weight. When growth is above expectations, equities, corporate bonds, and emerging market credit bonds will perform well; when growth is below expectations, nominal bonds and inflation-protected bonds will dominate; when inflation is above expectations, inflation-protected bonds, commodities, and emerging market credit bonds will excel; when inflation is below expectations, equities and nominal bonds will perform better.
"Introducing leverage for equal risk weighting" means the strategy needs to use leverage to align the risk-return characteristics of different assets, ultimately achieving 25% risk weight for each sub-portfolio. After completing the above two steps, if it is a relatively active allocation method, it will provide a judgment about the future, indicating what kind of environment the next phase will be in and which type of assets should be rotated into The "all-weather" strategy differs in that it subsequently adjusts through leverage while allocating multiple assets, with weights determined by equal risk contributions from each asset.
A risk parity portfolio without leverage will struggle to meet the dual constraints of higher expected returns and a higher Sharpe ratio. The contradiction lies in the fact that to achieve an expected return of 8%, asset selection will aim to be as close as possible to the "upper right corner," meaning that assets such as "non-US market indices," "private equity," and "emerging market bonds" will be assigned higher weights; however, these assets also have higher "volatility," which means that in the "risk parity" process, they will be assigned lower weights. Therefore, a risk parity portfolio without leverage finds it difficult to balance "expected returns" and "risk diversification," ultimately leading it to align more closely with bond indices, resulting in a significant gap from expected returns and only a slight advantage over bond indices. For this reason, although returns are a function of risk, different assets have different risk-return characteristics. Leverage has a "scaling" function; by applying leverage to low-volatility assets and adjusting the expected returns of various assets to an 8% level, we can obtain a new set of "assets"—with different volatilities but all yielding 8%. Finally, through the "parity" process of overweighting low-volatility assets and underweighting high-volatility assets, we achieve a final return of 8%, a volatility of 10%, and a Sharpe ratio of 0.8 for the portfolio. The portfolio achieves higher returns under the same Sharpe ratio, with actual positions being more diversified and risk contributions being equally weighted.
Finally, "dynamic risk rebalancing" refers to the next period's adjustment of asset weights to maintain risk parity by recalculating the volatility and correlation of each asset.
Fifth
Reviewing the historical performance patterns of the "all-weather strategy." Dalio, R (2015) [3] shows that during the declining interest rate period from 1981 to 2015, the all-weather strategy had an annualized return of 12.1%. Since 2015, there have been few public details on this strategy, but we can obtain performance results from overseas ETF websites (lazyportfolioetf.com) that trace back further (approximately 154 years since 1871). The lazyportfolioetf website shows that its compound returns over the past thirty years and ten years are 7.68% and 4.46%, respectively; volatility has increased from 7.48% to 8.45%, and the Sharpe ratio has decreased from 0.72 to 0.33. The internal composition of the portfolio shows that primarily U.S. Treasury ETFs have been a drag, while U.S. stocks and gold have supported returns in recent years. Simulating based on Dalio's stated allocation ratios (30% U.S. stock exposure, 40% long-term U.S. Treasury, 15% medium-term U.S. Treasury, and 7.5% each for gold and other commodities), the portfolio's annual compound return over the past ten years is also approximately 4.18%, which is roughly consistent with lazyportfolioetf data. Both methods indicate that the largest drawdown in the past thirty years occurred in 2022, with an estimated maximum drawdown of 23.8% according to experience, while lazyportfolioetf's website shows 20.58%, which is also roughly comparable In simple terms, its effectiveness has significantly declined over the past decade, but its effectiveness over a longer dimension is relatively high.
Dalio, R (2015) demonstrated that from 1946 to 2015, the all-weather annualized return was 10.4%, with an excess return of 1.9% per year compared to the 60/40 portfolio. Especially after 1981, as interest rates declined, the all-weather strategy entered a more comfortable zone, achieving an excess return of 2.6% per year compared to the 60/40 portfolio, with an absolute annualized return of 12.1%, and recorded a Sharpe ratio of 0.84 during that period. Although there has been little direct public information released regarding Bridgewater's all-weather strategy performance in recent years, a rough simulation can be made based on the allocation ratios recorded by Tony Robbins in 2014 from Dalio's self-reported experience (30% exposure to U.S. stocks, 40% long-term U.S. Treasuries, 15% intermediate-term U.S. Treasuries, and 7.5% each for gold and other commodities).
The overseas ETF website "lazyportfolioetf.com" shows similar simulation results. This website uses five overseas ETFs to replicate the all-weather portfolio: Vanguard Total Stock Market (VTI), iShares 20+ Year Treasury Bond Index (TLT), iShares 3-7 Year Treasury Bond Index (IEI), SPDR Gold Trust Index (GLD), and iShares Commodity Index (DBC). As of the end of January 2025, its simplified all-weather portfolio had a compound annual growth rate of 4.46% over the past decade, lower than the average annual compound return of 7.68% over the past thirty years. The recent decline in compound returns is mainly due to rising interest rates, which have caused U.S. Treasury ETF yields to drag down the portfolio, with long-term U.S. Treasuries showing compound returns of -2.03%, -7.38%, and -5.48% over the past ten years, five years, and one year, respectively. In contrast, gold and U.S. stocks are sources of return enhancement, with compound returns of 37.2% and 26.2% over the past year, respectively. Correspondingly, the portfolio's annualized volatility increased from 7.48% over the past thirty years to 8.45% over the past decade, and the Sharpe ratio decreased from 0.72 over the past thirty years to 0.33 over the past decade.
We also attempted to construct an overseas version of the all-weather portfolio using the experience values provided by Dalio, and changed the stock representative and intermediate-term U.S. Treasury representative to examine the robustness of the results, specifically replacing Vanguard Total Stock Market (VTI) with SPDR S&P 500 ETF Trust (SPY) and iShares 3-7 Year Treasury Bond Index ETF (IEI) with iShares 7-10 Year Treasury Bond Index ETF (IEF). As of the end of January 2025, the self-constructed (with changes to U.S. stock and intermediate-term U.S. Treasury representatives) all-weather portfolio had a compound annual growth rate of 4.18% over the past decade, which is roughly comparable to the lazyportfolioetf.com website. The recent decline in returns is also due to the drag from U.S. Treasury ETFs, with a compound return of 1.9% over the past five years, -2.9% over the past three years, and recovering to 12.5% over the past year Observing the sub-items, it is also noted that the recovery in earnings over the past year is mainly due to the reduced drag from U.S. Treasury ETFs, with U.S. stocks contributing a 23.4% increase and gold contributing 46.4%.
Combining the drawdown, the self-built portfolio shows a maximum drawdown of 23.8% over the past decade, occurring during the Federal Reserve's interest rate hike cycle in 2022. "lazyportfolioetf.com" provides a longer time dimension for examination, with portfolio performance tracing back to the 1930s during the Great Depression in the U.S., showing that the largest drawdown in nearly thirty years also occurred after the Federal Reserve began its current interest rate hike cycle in 2022, reaching 20.58%. From the 2008 financial crisis to the current drawdown in 2022, the portfolio's annual maximum drawdown was generally controlled within 8%. In a longer historical context (approximately 154 years since 1871), the current drawdown magnitude ranks second historically, with the first being during the Great Depression in the U.S. (September 1929 - May 1932), with a maximum drawdown of 37.02%.
This indicates that an all-weather strategy is not always effective. The volatility and drawdown around 2022 have significantly increased, which may be related to the renewed rise in U.S. interest rates. As Bridgewater mentioned in its January 2024 report "The Big Picture: Back to the Future," the current environment is significantly different from the past twenty years, resembling much earlier historical periods—returning to rates and inflation. The significant boost to the overall BETA returns of equity risk assets from the previous overseas low-interest rate environment (Exceptional) is likely to return to normal (Likely More Normal).
Sixth
**Further review of the historical performance patterns of broadly defined "risk parity funds." Observing PanAgora Risk Parity, the S&P Risk Parity Index, etc., it is similarly found that their "golden age" began after the 2008 financial crisis, faced skepticism from 2013 to 2015 (underperforming the US 60/40 portfolio), rebounded in 2016-2017, and returned to mediocrity after 2018. The annual excess returns of the S&P 500 Risk Parity Index from 2009 to 2012 (compared to the US 60/40 portfolio) were 3.77%, 15.27%, 8.91%, and 5.86%, totaling 33.81%. In 2013, under expectations of tightening overseas monetary policy, the risk parity index underperformed the US 60/40 portfolio by nearly 12 percentage points. The moderate decline in interest rates in 2016-2017 corresponded to a rebound in the S&P 500 Risk Parity Index, recording annualized returns of 13.81% and 10.81%. After 2018, the impact of the pandemic and the Federal Reserve restarting a new round of interest rate hikes did not favor risk parity. In summary, since risk parity relies on the premise of positive long-term returns for assets, when all assets experience a significant increase in volatility due to exogenous shocks or when interest rates rise unexpectedly, simple risk parity strategies are relatively fragile. Therefore, risk parity strategies are not universally applicable or a one-size-fits-all solution; they still require suitable macro and market conditions **
Not only Bridgewater's "All Weather Fund," but AQR, PanAgora Risk Parity, and even the S&P 500 Risk Parity Index exhibit similar patterns. We compared the S&P 500 Risk Parity Index with the classic US 60/40 portfolio (60% S&P 500 Index, 40% US Treasury Total Return Index) since 2004 and found that it performed remarkably well after the financial crisis, consistent with Bridgewater's All Weather strategy.
In May 2013, when the Federal Reserve indicated a potential reduction in bond purchases, market panic selling of US Treasuries led to rising interest rates, causing the Risk Parity Index to significantly underperform the US 60/40 portfolio by nearly 12 percentage points that year, raising doubts about its "sacred" status.
During the period of 2014-2015, as the Federal Reserve exited QE and entered a tightening cycle, the Risk Parity Index still did not outperform the US 60/40 portfolio. Although the Federal Reserve was still in a rate-hiking cycle in 2016-2017, US Treasury rates had already risen significantly during the prior phase of tapering and rate hike expectations, leading to a moderate decline in rates during that period, which allowed the S&P 500 Risk Parity Index to rebound.
However, since 2018, with the impact of the pandemic and the Federal Reserve restarting rate hikes, the Risk Parity Index has not enjoyed a comfortable environment, consistent with the aforementioned All Weather strategy. The pandemic temporarily increased the volatility of multi-assets such as stocks and bonds, and the simultaneous asset pullback allowed Risk Parity to achieve a high Sharpe ratio through risk diversification; however, the Federal Reserve's new round of rate hikes put pressure on the Risk Parity strategy, which is sensitive to interest rate risks.
Combining the aforementioned Risk Parity concept, we can see that the operation of the Risk Parity strategy, which involves "overweighting low-volatility assets and underweighting high-volatility assets," inherently has a "volatility reduction" function. However, a simple Risk Parity strategy relies on the premise that assets have positive long-term returns. When all assets experience a significant increase in volatility due to exogenous shocks or when interest rates rise significantly (beyond expectations), a simple Risk Parity strategy is relatively fragile and carries a risk of pullback. As a practical strategy, Risk Parity is not a "one-size-fits-all" or "set it and forget it" strategy, nor is it completely "outdated" or should it exit the historical stage; it still requires a suitable market and macro environment.
Seventh
**Looking further at the domestic macroeconomic environment and financial market conditions, the applicability of the Risk Parity strategy seems to be on the rise. First, as China's economy enters a new stage of high-quality development, the elasticity of economic growth, inflation, and monetary cycles has become smoother, and the properties of interest rate "cyclical goods" have diminished, leading to a convergence in the volatility of risk and risk-free assets; second, with the continuous development of domestic financial markets, there has been significant progress in asset selection diversification and financial instrument variety, all of which are conducive to the application of Risk Parity and All Weather strategies; third, with the rise in global macroeconomic uncertainty and changes in the characteristics of the wealth management market, there is a greater demand for risk diversification in investments (placing more emphasis on the "Sharpe ratio"), making the "volatility reduction" function of Risk Parity attractive **
We used two sets of empirical cases to find that over the past decade, timing and stock selection in the domestic market have been more significant than allocation; however, this may change in the future, with the core variables being macroeconomic growth, inflation, and the elasticity of the monetary cycle smoothing, which may lead to convergence in asset price fluctuations.
First, we constructed an efficient frontier for global major asset classes. The tangent portfolio shows that from 2015 to 2024, in an assumed environment of free allocation, the optimal Sharpe ratio for a portfolio spanning global stocks, bonds, commodities, and currencies is 3.63. The top five weighted assets are Chinese government bonds, gold, the US dollar, emerging market valuations, and US stocks; while the weights of Chinese equity assets such as the CSI 300, CSI 2000, and Hang Seng Index are relatively lower, indicating that in a short-selling allowed scenario, the portfolio with the highest Sharpe ratio over the past decade mainly invested in Chinese government bonds, gold, the US dollar, emerging market countries, and US stocks; whereas the risk-return ratio of domestic A-shares and Hong Kong stocks is relatively lower, which may require more proactive investment (stock selection and timing).
Second, we compared the return differences between perfectly timed and completely mistimed investments in the Chinese and US markets. We used the Wind All A and S&P 500 as representatives for A-shares and US stocks, respectively, designing two testing dimensions with annual and monthly timing strategies. Scheme one involves buying at the highest point of the year, equivalent to completely mistimed timing; scheme two involves buying at the lowest point of the year, equivalent to perfectly timed timing. Between 2011 and 2024, the annualized return for perfect timing in the A-share market was 3.9%, while the annualized return for completely mistimed timing was 1.3%, with a difference of 2.6% and a ratio of 2.94 times. In other words, over the past fifteen years, the return difference in A-shares based on timing accuracy has been significant, with the return from correct timing being 2.94 times that of incorrect timing. During the same period from 2011 to 2024, the difference in timing for the S&P 500 was not significant. If we look at a longer time frame, from 2000 to 2024, even though it could not avoid the internet bubble of 2000, the difference between correct and incorrect timing for the S&P 500 was only 1.4%, with the annualized return from correct timing being only 1.3 times higher than that from incorrect timing. If the timing frequency is raised to monthly, the return difference based on timing accuracy is only 0.3%, with the annualized return from correct timing at 5.6%, which is close to the 5.3% from incorrect timing.
From the annual amplitude (annual maximum index point - annual minimum index point / annual minimum index point) reflecting volatility, the annual volatility of the domestic equity market is more pronounced than that of the US S&P 500. Since 2014, the domestic ChiNext has had higher volatility than US stocks in all years except 2020. Therefore, although overseas, Gary P. Brinson, who created the Brinson performance attribution model, pointed out in his 1986 paper that asset allocation explains an average of 91.5% of the total quarterly returns of portfolios; for A-share investors, whether through active stock selection or active timing, remains a core issue and an important source of excess returns If we follow the idea of risk parity, the high volatility of domestic equity assets will naturally lead to a lower allocation weight for stocks and a higher allocation weight for relatively low-volatility bond-like assets (including low-volatility dividend assets). If future total elasticity smooths out and financing costs decrease, combined with a convergence of stock and bond volatility, risk parity may find a more comfortable development environment. In our previous report "The Macro Logic and Timing System of High Dividend Strategies," we pointed out that from a medium to long-term perspective, as domestic economic growth no longer solely pursues speed, and with the strong manufacturing supply capacity under the background of a manufacturing powerhouse, the future price elasticity of industrial products and the total elasticity of the monetary cycle may both be moderate.
After the 2008 financial crisis, the United States experienced a downward trend in the interest rate center, low growth, and low inflation. During this period, on one hand, the valuation center of U.S. stocks gradually trended upward, and volatility converged; on the other hand, risk parity and other leverage-dependent allocation models gained recognition and significant development. If a similar macro portfolio emerges domestically in the future, and the properties of past interest rate "cyclical products" fade, the volatility of risk assets and risk-free assets in China may converge, potentially leading to a better risk-return ratio for domestic asset portfolios.
Eighth
We have initially attempted to build a parity model. We conducted three sets of tests: basic global asset indices (six categories), basic ETFs (seven categories), and extended ETFs (eleven categories). Consistent with the MVO test results, since 2015, the best risk-return ratio has been in China bonds (especially credit bonds), followed by gold, U.S. stocks, then dividend assets, the CSI 300, and the Hang Seng Index. If we further shorten the period to since 2021, gold ranks best, with dividends following closely. The variance-covariance matrix of returns between assets is a core input parameter for the risk parity model. Whether looking at long-term or rolling correlations, Chinese assets not only show a negative correlation between stocks and bonds, but also a negative correlation between bonds, Hong Kong stocks, and China bonds; dividend assets, which have some "bond-like" characteristics, generally maintain a positive correlation with the market, although this positive correlation has significantly weakened since 2020. The Chinese concept internet and the domestic CSI 300 ETF have a strong and stable positive linkage; the CSI REITs total return index has shown low correlation with pure stock indices and pure government bonds since 2024.
In terms of asset selection, we first selected "CSI 300 Index, Hang Seng Index, S&P 500 Index, China Bond Corporate Bond Total Index, COMEX Gold Futures Closing Price, Nanhua Comprehensive Index" as the basic global asset pool, covering six major markets: A-shares, Hong Kong stocks, U.S. stocks, China bonds, gold, and commodities. Additionally, we replaced traditional major assets with "ETF products" to test the risk parity model, and given that the KWEB ETF representing the Chinese concept internet index and REITs began developing after 2020, we defined two sets of ETF asset pools: "basic version" and "extended version." The "basic version" ETF asset pool involves relatively fewer assets but has a backtesting period that can be traced back to January 1, 2015; the "extended version" ETF asset pool adds four categories: Hong Kong stocks, Chinese concept stocks, convertible bonds, and CSI REITs on top of the "basic version" framework The "expanded" ETF asset pool has products that have emerged in recent years, so the backtesting period can only trace back to August 2021.
First, in the basic global asset class index pool, from 2005 to February 5, 2025, the best risk-return ratio is the China Bond Corporate Bond Total Index, with a Sharpe ratio of 1.90. Due to a certain upward trend in gold in recent years, its risk-return ratio ranks second, with a Sharpe ratio of 0.47. The risk-return ratios that are relatively lower are the South China Comprehensive Index, CSI 300, and Hang Seng Index. This result is consistent with the findings in the first part of the MVO.
In the ETF asset pool, from 2015 to February 5, 2025, the best risk-return ratio is domestic credit bonds, with the Sharpe ratio of the City Investment 500 ETF at 0.99 and a maximum drawdown of 5.5%. Next are U.S. stocks and gold. When the review period is shortened to nearly ten years, the Sharpe ratios for U.S. stocks and gold increase to 0.69 and 0.64, respectively, while the Sharpe ratios for CSI 300 ETF and commodity ETFs drop to around 0. Among A-share equity assets, the Sharpe ratio for dividend assets is significantly higher than that of CSI 300, but still lower than government bonds and credit bonds. If the review period is further shortened to since August 2021, the best risk-return ratio is for gold ETF, with a Sharpe ratio of 1.34; the advantage of dividend assets over CSI 300 further expands, increasing to 0.28.
From a long-term correlation perspective, from 2005 to February 5, 2025, Chinese assets not only show a negative correlation between stocks and bonds (the correlation between the CSI 300 Index and the China Bond Corporate Bond Total Index is -0.28), but also negative correlations between bonds and Hong Kong stocks with the China Bond (the correlations of the China Bond Corporate Bond Total Index with the South China Comprehensive Index and the Hang Seng Index are -0.35 and -0.33, respectively). Correspondingly, A-shares have a certain degree of correlation with Hong Kong stocks and domestic commodities, with the interval correlation between CSI 300 and the Hang Seng Index at 0.54, and the interval correlation with the South China Comprehensive Index at 0.28. Hong Kong stocks not only correlate with A-shares but also have an interval correlation of 0.61 with U.S. stocks. From the rolling 6-month window, it can also be seen that the domestic large-cap stocks and bonds have generally shown a negative correlation since 2018, with a significant weakening of the negative correlation during 2020-2021, and a "seesaw" return after 2022. Among them, the negative correlation between government bonds and stock indices is more pronounced, while the correlation between credit bonds and stock indices generally runs between [-0.2, 0.2]. In contrast, dividend assets, which have some "bond characteristics," generally maintain a positive correlation with the large-cap index, although this positive correlation has significantly weakened after 2020, oscillating at a lower correlation center than before 2020.
Ninth
From the backtesting results, the risk parity model has a higher Sharpe ratio, win rate, and payout ratio compared to the simple equal-weight and simple volatility inverse-weighted models, and this advantage is more pronounced after 2022. Since 2009, under the basic asset class risk parity scheme, the Sharpe ratio, win rate, and payout ratio are 1.79, 58.3%, and 1.06 (profit-loss ratio), respectively, which are higher than the 0.62 under the equal-weight scheme 53.0%, 1.03; from 2022 to February 2025, the Sharpe ratio, win rate, and odds under the risk parity scheme for basic asset classes are 3.37, 62.1%, and 1.35, respectively, while under the corresponding equal-weight scheme they are 1.07, 51.4%, and 1.17. Under the volatility-weighted scheme, they are 2.47, 58.9%, and 1.24, respectively. During the same period, the risk parity scheme for the basic ETF asset pool shows 2.04, 60.6%, and 1.05, while the equal-weight scheme shows 1.51, 54.8%, and 1.08; the risk parity scheme for the expanded ETF asset pool shows 0.89, 57.4%, and 0.99, while the equal-weight scheme shows 0.88, 52.6%, and 1.07. The years with relatively poor strategy performance are 2011, 2013, 2018, and 2022. In domestic testing, the historical scenarios unfavorable to risk parity are first, multi-asset linked pullbacks (most typically, both stocks and bonds falling, or both stocks and commodities falling), and second, when a particular asset experiences excessive volatility. In terms of weights, the relatively stable net value of the unleveraged basic risk parity still benefits from the relative overweight in bond assets. Compared to the proportions given by Dalio, the total proportion of bond assets in domestic risk parity reaches 74.4%, making it a more dominant and core underlying asset.
Based on the rolling correlation of asset returns (covariance matrix), we use equal weights for all assets as the initial weights, and then perform nonlinear optimization on the risk parity objective function to solve for the numerical solution weights that equalize risk contributions. Given the changing market environment, we rebalance on a monthly basis to ensure that we generally remain in a risk parity state. To view the risk parity results more objectively, we designed two additional benchmark schemes: one where all assets operate continuously under the initial equal-weight method, and the other where all assets directly use the inverse of the past 6 months' volatility for weighting, without involving optimization based on the correlation between assets.
From 2022 to February 2025, the Sharpe ratio, win rate, and odds for the original basic assets under the equal-weight scheme are 1.07, 51.4%, and 1.17 (profit-loss ratio); under the volatility-weighted scheme, they are 2.47, 58.9%, and 1.24; and under the risk parity scheme, they are 3.37, 62.1%, and 1.35. If we extend the view to since 2009, the Sharpe ratio for the risk parity scheme of original major asset classes is 1.79, higher than the simple equal-weight of 0.62 and also higher than the simple volatility-weighted of 1.62; the win rate also rises from 53% under equal weight to 58.3% under volatility weighting, and further to 59.7% under the risk parity scheme; the odds (profit-loss ratio) also increase.
If we observe the basic ETF asset pool, from 2022 to February 2025, the Sharpe ratio, win rate, and odds under the equal-weight scheme are 1.51, 54.8%, and 1.08 (profit-loss ratio); under the volatility-weighted scheme, they are 1.96, 58.9%, and 1.10; and under the risk parity scheme, they are 2.04, 60.6%, and 1.05. Further, for the expanded ETF asset pool, from 2022 to February 2025, the Sharpe ratio, win rate, and odds under the equal-weight scheme are 0.88, 52.6%, and 1.07 (profit-loss ratio) Under the volatility-weighted scheme, the respective values for the same period are 0.61, 54.6%, and 1.04; while under the risk parity scheme, they are 0.89, 57.4%, and 0.99.
Throughout the entire period, the relatively significant drawdowns for risk parity occurred in the third quarter of 2015 and the fourth quarter of 2022. From the perspective of annual return volatility, the years with relatively large drawdowns and low Sharpe ratios for risk parity were 2011, 2013, 2018, and 2022. Combined with the net value curve, it can be seen that the unfavorable asset scenarios for the basic risk parity model are firstly multi-asset linked corrections (the most typical being simultaneous declines in stocks and bonds, or stocks and commodities), and secondly, excessive volatility in a particular asset. The year 2011 saw a decline in inflation, the European debt crisis, tightening liquidity, and a drop in risk appetite, leading equities, Hong Kong stocks, and commodities into a downward cycle; in 2013, both stocks and bonds adjusted under the "money shortage"; in the second half of 2015, the stock market experienced a significant correction; in 2018, the stock market adjusted significantly under real deleveraging; and in 2022, the stock market was sluggish due to pandemic disruptions and the Federal Reserve's interest rate hikes.
From the perspective of initial weights, the allocation weight of government bond ETFs is higher than that of credit bond ETFs, with an average weight of 43% and 31.4% from 2015 to the present. Next are gold assets, which, compared to Dalio's suggested allocation of "30% U.S. stocks, 40% long-term U.S. Treasuries, 15% medium-term U.S. Treasuries, and 7.5% each for gold and other commodities," the total weight of domestic risk parity bond assets reaches 74.4%, making them a more core underlying asset.
If we observe the weights of various assets in the expanded ETF asset pool since 2022, the key positions remain in bonds, with the average weight of government bond ETFs being 43.6% since 2022, and even at the lowest point, the weight was 25.9%; followed by corporate bond ETFs with an average weight of 28.4%; the average weights for CSI REITs and gold are 5.1% and 7.5%, respectively, while the remaining seven assets account for a total of 14.4%. This indicates that the domestic ETF implementation of the risk parity scheme will assign a lower weight to equity assets due to their high volatility, making bonds the absolute dominant underlying asset. On the other hand, it also means that the current simple risk parity application in the domestic market will be exposed to greater interest rate risks, with a more pronounced vulnerability to rising interest rates.
Chapter Ten
We further introduce leverage. As mentioned earlier, while the unleveraged risk parity portfolio has a high Sharpe ratio, its returns are not attractive. There are two ways to introduce leverage: one is to leverage the assets first and then achieve parity; the other is to achieve parity first and then leverage the portfolio. The former is the approach taken by Bridgewater, while the latter is simpler and more direct, which is to directly leverage the portfolio after achieving parity by obtaining funds from the capital market (pledging bond assets for repurchase) to continue buying into the parity portfolio. The cost of funds is related to the market financing cost and is more closely related to short-term interest rates. This article adopts a method of leveraging while considering domestic actual conditions, limiting leverage to the range of 80% to 140%. We tested the effects when the target volatility is 2%, 3%, 4%, 5%, and 6%. In the three asset pools, the annualized return and annualized volatility of the portfolio both increase with the target volatility, while the Sharpe ratio slightly declines Under the basic ETF risk parity scheme, since 2015, the annualized return of the portfolio has increased from 5.1% to 6.4% as the target volatility rises from no target volatility to a target volatility of 6%, while the annualized volatility has increased from 2.9% to 4.8%, and the Sharpe ratio has decreased from 1.07 to 0.92. If we further shorten the period to since 2022, with the increase in target volatility, the annualized return of the portfolio has risen from 6.0% to 8.3%, the annualized volatility has increased from 2.0% to 3.3%, while the Sharpe ratio has only decreased from 2.04 to 1.93.
In the empirical case of ETFs in China, if leverage is not used, over 70% of the portfolio is allocated to low-volatility bond assets (about 40% in government bonds and about 30% in credit bonds), and the source of the portfolio's Sharpe ratio mainly comes from low volatility (around 2.0%), making it necessary to enhance the attractiveness of returns.
We tested the strategy effects with target volatilities of 2%, 3%, 4%, 5%, and 6%. Due to leverage constraints, the actual annualized volatility of the portfolio cannot fully match the set target volatility, but it shows a monotonically positive correlation.
Under the risk parity scheme of basic major asset indices, since 2009, the annualized return of the portfolio has increased from 6.6% to 7.8% as the target volatility rises from no target volatility to a target volatility of 6%, while the annualized volatility has increased from 2.6% to 3.3%, and the Sharpe ratio has decreased from 1.79 to 1.74.
Under the basic ETF risk parity scheme, since 2015, the annualized return of the portfolio has increased from 5.1% to 6.4% as the target volatility rises from no target volatility to a target volatility of 6%, while the annualized volatility has increased from 2.9% to 4.8%, and the Sharpe ratio has decreased from 1.07 to 0.92. The extended ETF scheme also has similar effects, with annualized returns increasing by 0.4 percentage points, annualized volatility increasing by 1.1 percentage points, and the Sharpe ratio declining by 0.2.
Although the introduction of leverage brings new risks—sensitivity to short-term funding rates—it also sacrifices some risk-return ratios. However, for investors with a certain risk appetite, the leverage mechanism allows them to achieve higher investment returns through risk parity within the range of risks they are willing to bear.
Eleventh
A brief summary of the re-understanding of all-weather strategies. Each financial crisis prompts investors to reflect on the importance of risk awareness, making risk management more crucial. The risk parity model and all-weather strategies are a concrete expression of this investment philosophy. After the 2008 financial crisis, the United States initiated quantitative easing, and the overseas macro environment entered an era of "low interest rates, low inflation, and low growth." The "risk parity" strategy found the most comfortable growth soil. With the rise of inflation post-pandemic, the Federal Reserve's interest rate hikes, and the significant upward trend in U.S. Treasury yields, all-weather strategies have also undergone an adjustment period. Combined with the situation during the Great Depression overseas and the historical verification of basic risk parity in China, we understand that the premise of the "all-weather strategy" is that assets exhibit rotation performance, and long-term returns are positive In short, this strategy implies the premise that "everything has its time" and "if the East does not shine, the West will." If the performance of stocks, bonds, and businesses is poor, the effectiveness of the strategy will also decline significantly. From this understanding, during periods when domestic total growth fluctuations gradually stabilize, inflation elasticity decreases, and interest rate levels are not high, the applicability and practicality of risk parity strategies may increase.
Risk Warning: First, the regression model construction is based on historical data, which may have errors in the event of significant economic shocks or market changes in the future; second, the model still needs to conduct sensitivity analysis on various parameters to improve the robustness of the strategy; third, there may be significant events that change expectations at the fundamental level, such as domestic real estate policy effects falling short of expectations; the impact of the new round of local government debt reduction exceeding expectations; subsequent fiscal policy strength exceeding expectations, etc.
Main Text
PART1 Introduction: Understanding the All Weather Strategy
The "All Weather Strategy" is a well-known asset allocation strategy first proposed by Bridgewater Associates in 1996. Its main idea is not to rely on forward-looking predictions and timing but to attempt to achieve a diversified asset allocation and dynamic adjustment so that the investment portfolio can traverse cycles and have certain applicability under different economic conditions, thereby achieving relatively stable returns.
The concept of the "All Weather Strategy" comes from the investment return decomposition equation of the Dalio team:
Fund Return = Risk-Free Rate + Market Risk Premium + Active Management Return, or R = Rf + β + α.
By separating β and α, the strategy aims to desensitize the portfolio's Beta to a single asset through an all-weather approach, obtaining a more robust, long-term stable market β return that can traverse cycles, and then subjectively enhancing it to capture α. Therefore, the All Weather Strategy is the underlying strategy of Bridgewater Associates, focusing on diversified allocation, dynamic adjustment, and risk balance, allowing the portfolio's risks to offset each other under different economic states, leaving the overall risk premium that grows over time. The entire philosophy of Bridgewater Associates is neither completely active (subjective allocation after identifying macro risks and superior underlying assets) nor completely passive.
Among hedge fund strategy categories, since the "All Weather Strategy" views the essential laws of asset operation as macro risks (growth, inflation), it belongs to a passive allocation within "macro strategies," which weakens timing as a school of thought. Macro hedge strategies essentially utilize macro information for large asset rotation and allocation. Since the 21st century, due to increased global macro uncertainty, funds using this strategy have rapidly risen. According to HedgeList data, by the end of 2024, approximately 13.6% of the top 250 hedge funds globally manage their allocations through macro strategies.
Overseas macro strategies are divided into two major factions due to their different focuses on timing and allocation:
One is more inclined towards active timing, relying on the accuracy of subjective predictions. Represented by Soros Fund Management and Brevan Howard, they actively identify mispricing, engage in long-short trading, and freely allocate assets;
The other is more inclined towards passive allocation, relying on the effectiveness of model construction. Represented by Bridgewater's "All Weather" strategy, it downplays macro timing and does not focus on predicting macro risks in advance, always maintaining a diversified asset position, benefiting from certain sub-portfolios that may rise in different macro scenarios.
PART2 The Logical Starting Point of Asset Allocation
(1) Starting from the Mean-Variance Model (MVO)
Looking at the entire evolution of "asset allocation," its underlying theme and logical starting point revolve around "risk." When discussing asset allocation, many people might recall a saying: "Don't put all your eggs in one basket." This is essentially a philosophical understanding of the need to diversify risk in investments. However, before Harry Markowitz proposed Modern Portfolio Theory (MPT) in 1952, this concept existed only as a simple qualitative understanding.
Prior systematic discussions on stock and bond valuation theories were mainly represented by John Burr Williams' "The Theory of Investment Value," which understood investment returns through intrinsic value and discounted cash flow models, believing that the core of the investment process is to break down the sources of returns, without giving sufficient attention to risk (or volatility).
Before delving into the topic of "risk parity strategy," we first trace back to Harry Markowitz's Mean-Variance Model (MVO) from 1952, which describes asset characteristics using two dimensions: return (mean) and risk (variance), showing that the main purpose of asset allocation is to reduce correlation through asset diversification, lower investment risk, and achieve maximum returns per unit of risk—maximizing the Sharpe ratio.
The core of the Mean-Variance Model (MVO) is "two lines, one tangential point":
One end represents the indifference curve of investor preferences (return, risk);
The other end represents the efficient frontier of the relationship between portfolio returns and risks.
The tangential point of the two is the optimal combination that maximizes returns per unit of risk.
The core assumption of the model is that investors are risk-averse, and people's utility functions are simultaneously influenced by the interaction of returns and risks. What investors most desire is to maximize returns at a given risk level and minimize risk at a given return level The process of asset allocation is quantified for the first time under this model as solving an "optimization problem" with certain constraints.
However, the core reason why the Mean-Variance Optimization (MVO) model does not perform well in practice is that it still requires predicting the future, relying too heavily on the past and being highly sensitive to parameters.
This model is extremely sensitive to input parameters (returns, covariance matrix) (V. Chopra and W. Ziemba, 1993) [3], and even a slight estimation error in parameters can completely change the designed portfolio. One major input of the model is the investor's prediction of future asset returns, which is not an easy task:
If subjective forecasting is used, investors need to have a good grasp of the underlying variables of the future world, which is difficult to predict. It assumes that investors can accurately estimate expected returns (Black and Litterman, 1992) [4].
If time series models (such as ARMA) are used, or historical data is directly utilized as a substitute variable, the implicit assumptions are that "history is repeatable" and "future changes are minimal." In recent years, the macro environment has become complex, undergoing unprecedented changes in a century, and simple modeling methods and using historical returns as future proxy variables are biased. In practice, the theoretical allocation scheme provided by the MVO model may not be a truly "optimal portfolio."
Therefore, the two paths for improvement are also divided into two. One is the Black-Litterman model proposed by Fischer Black and Robert Litterman [5], which better combines subjective views and quantitative models through Bayesian theory to optimize estimates of asset expected returns; the other includes models such as "Risk Parity," minimum variance models, and maximum diversification models, which abandon the estimation of asset expected returns and instead focus on allocation based on "risk."
(2) Risk Parity is a framework based on "risk."
As mentioned above, the two paths to improve the classic Mean-Variance model are more accurate estimates of the future (Black-Litterman model) and "breaking out" of predictions to pursue risk balance (Risk Balancing).
Path One (Black-Litterman model) actually still unfolds along the theory of Mean-Variance, only with more optimized input parameters, while Path Two represents a leap and innovation from "allocating assets" to "allocating risk," which is more akin to a parallel branch of the Mean-Variance model Path Two ("Risk Parity" Model, etc.) abandons the difficult-to-predict return benchmark and only considers "parity" of risk, ensuring that each type of asset contributes equally to the overall portfolio's volatility. To give a simple example, in a portfolio consisting only of stocks and bonds, if the overall portfolio's volatility target is set at 5%, both stocks and bonds should contribute half of that, or 2.5% of volatility. Given that the volatility of stocks as risk assets is higher than that of bonds, the weight of stocks in a risk parity portfolio will be significantly lower than that of bonds. From the underlying logic of the strategy, the concept of risk parity represents a transition and innovation from "allocating assets" to "allocating risk," and is parallel to the mean-variance model.
Thus, we can simply divide the theoretical framework of major asset allocation into two strands.
One is the "Return Based Allocation Models," such as constant mix models, mean-variance models (MVO), and the B-L model that incorporates subjective views.
The other is the "Risk Based Allocation Models," such as the risk parity model and the upgraded risk budgeting model.
Although the essence of "risk parity" is a re-examination of "risk," presenting a transition from "allocating assets" to "allocating risk," if we broaden our perspective, "risk parity" resembles an evolutionary process in investment philosophy (especially after experiencing significant systemic risks like the 2008 financial crisis), moving from a pure pursuit of returns to an awakening of "risk" awareness, and then to a better balance of "risk."
The concept of Risk Parity was first proposed in 2005. At that time, Dr. Edward Qian, Chief Investment Officer of PanAgora Asset Management, attributed the historical performance of a constant stock-bond portfolio (with the Russell 1000 Index and the Lehman Aggregate Bond Index accounting for 60% and 40%, respectively) [6]. He found that although the portfolio was easy to construct and diversified, it did not balance risk. Between 1983 and 2004, the Russell 1000 contributed 93% of the volatility (variance) of the portfolio, while bonds contributed only the remaining 7%. In other words, the portfolio was actually exposed to more volatile equity assets.
He constructed a simple risk parity portfolio by allocating 23% of the position to the Russell 1000 Index and evenly distributing the remaining 77% to the Lehman Aggregate Bond Index, **to ensure that both contribute equally to the portfolio's volatility **
Theoretically, risk parity reduces volatility through diversification while increasing returns through leverage, thereby improving the Sharpe ratio.
If we plot Qian (2005)'s risk parity portfolio and a constant proportion portfolio (60% stocks and 40% bonds) on a risk-return coordinate system, we can see that the constant proportion portfolio of 60/40 stocks and bonds is located at the upper right end of the boundary, close to an all-equity portfolio.
The return of the unleveraged version of the risk parity portfolio is significantly reduced, but it achieves a higher Sharpe ratio with a decrease in volatility (the slope of the risk parity CML is steeper). The leveraged version of the risk parity portfolio moves along the risk parity CML line to the right of the unleveraged version, indicating that after leveraging with risk-free rate financing, although volatility increases again, the increase in returns is more pronounced. We can find a combination that, after leveraging, has the same return as the original 60/40 stock-bond portfolio but with lower volatility, resulting in a better risk-return ratio.
In simple terms, returns are compensation for risk (volatility), and the process of "equalizing" "risk" in the risk parity strategy is inherently a process of overweighting low-volatility assets and underweighting high-volatility assets.
Further comparison of this portfolio with the benchmark index and the 60/40 stock-bond investment portfolio reveals that during the period from 1983 to 2004, without considering leverage, the Sharpe ratio of the risk parity portfolio was 0.87, higher than any single asset portfolio, truly achieving a higher unit risk return through diversification.
In contrast, the Sharpe ratio of the 60/40 stock-bond investment portfolio was 0.67, showing limited improvement compared to the pure stock index of 0.55, and still significantly lower than the Sharpe ratio of the pure bond composite index of 0.80. This indicates that while the 60/40 stock-bond portfolio has some diversification, the degree of diversification is not high, and the overall Sharpe ratio of the portfolio is lower than that of some of its internal assets.
At the same time, Qian (2005) also pointed out that a leveraged risk parity investment portfolio can achieve higher returns while maintaining a high Sharpe ratio. In Qian (2005)'s case, although the unleveraged version of the risk parity portfolio had a high Sharpe ratio, the returns were not high, with an annualized return of only 4.7%, slightly above that of the bond index.
One solution is to use leverage to achieve a higher level of returns. Under the assumption of a leverage ratio of 1.8:1, the risk parity portfolio achieves an annualized return of 8.4% while maintaining the same Sharpe ratio of 0.87, surpassing the 8.3% return rate of the pure stock index.
PART3 Deconstructing the Core of the All-Weather Strategy
(1) All-Weather ≠ Risk Parity
The "All-Weather Strategy" is essentially a model that utilizes the concept of risk parity, but the two are not entirely equivalent. Risk parity is a mathematical model that balances risk without considering macroeconomic factors; it simply designs asset allocation weights that are more risk-diversified based on the historical volatility of assets over a certain period. As a concept, its underlying structure still has a lagging nature and relies on historical data. Compared to the Mean-Variance Optimization (MVO) model, the historical representativeness of volatility is more meaningful for simple future predictions than that of returns, addressing issues such as parameter sensitivity and extreme positioning.
The "All-Weather" approach is more like a macro strategy model, fundamentally starting from the macroeconomic cycle, but it does not attempt to identify and predict cycles. Instead, it uses the concept of risk parity to avoid losses caused by misjudging the cycle. Therefore, the "All-Weather" strategy complements the alpha strategy in Bridgewater Associates.
(2) Strategy Concept: "Two Core Principles, Four Steps"
We summarize the All-Weather Strategy as "Two Core Principles, Four Steps." The two core principles are "Risk Parity + Dynamic Adjustment," and the four steps are "Define States, Select Assets, Introduce Leverage for Equal Risk Weighting, Dynamic Rebalancing."
Step One: "Define States" refers to the model's assumption that asset volatility arises from unexpected growth or inflation.
It is worth noting that, superficially, the Merrill Lynch Clock is somewhat similar to the All-Weather Strategy, as both categorize the macro environment into four quadrants. However, the Merrill Lynch Clock is an active depiction of the economic cycle, with its four quadrants corresponding to growth-inflation pairings: recovery, overheating, stagflation, and recession. It attempts to determine and predict the macro quadrant in which the future will be, thereby finding the corresponding dominant assets.
In contrast, the All-Weather quadrants categorize growth and inflation based on whether they exceed expectations, dividing them into "Growth Exceeds Expectations, Growth Below Expectations, Inflation Exceeds Expectations, Inflation Below Expectations."
In other words, if the macro world can be understood through growth and inflation, then at any given moment, it will always be in one of the four states, such as unexpected growth and inflation exceeding expectations. These four configuration states do not exist in a cyclical rotation over time; they simply objectively delineate four independent configuration scenarios.
Step Two: "Select Assets" means the strategy will identify the dominant assets under the four states, with each quadrant allocated 25% of the risk weight. For example:
When growth exceeds expectations, equities, corporate bonds, and emerging market credit bonds will perform well;
When growth is below expectations, nominal bonds and inflation-protected bonds will dominate;
When inflation exceeds expectations, inflation-protected bonds, commodities, and emerging market credit bonds will excel;
When inflation is below expectations, stocks and nominal bonds will perform better Step Three: "Introducing Leverage for Risk Parity" refers to the strategy that requires leveraging to align the risk-return characteristics of different assets, ultimately achieving a risk weight of 25% for each sub-portfolio.
After completing the above two steps, if the allocation method is more active, it will provide judgments about the future, indicating what kind of environment the next phase will be in and which type of assets should be rotated into.
In contrast, the "All Weather" strategy adjusts through leverage and allocates multiple assets, with weights determined by equal risk contributions from each asset. In other words, the underlying idea is that, in the long run, since the portfolio has achieved risk balance across the four configuration scenarios facing the objective world, the risks of the four economic environments will offset each other, leaving behind the returns that will grow over time, which is the overall risk premium.
During this process, Bridgewater's All Weather adjusts the risk-return characteristics of various assets to be "fundamentally similar" through leverage. If leverage is not considered, even if the portfolio's Sharpe ratio is attractive, the returns will fall far short of expectations due to excessively low volatility. As shown in the figure below, if the expected return is 8%, then a Sharpe ratio of 0.8 corresponds to an acceptable volatility of 10%. However, an unleveraged risk parity portfolio will be unable to meet the dual constraints of an 8% expected return and a 0.8 Sharpe ratio. The contradiction lies in:
To achieve an expected return of 8%, asset selection will try to get as close to the "upper right corner" as possible, meaning that assets like "non-U.S. market indices," "private equity," and "emerging market bonds" in the upper right corner will be assigned higher weights;
However, these assets also have higher "volatility," which means that in the "risk parity" process, they will be assigned lower weights.
Therefore, an unleveraged risk parity portfolio finds it difficult to balance "expected returns" and "risk diversification," ultimately leading to a situation where it can only converge towards bond indices to achieve "risk parity," resulting in a significant gap from the expected return and only a slight advantage over bond indices. For this reason, Dr. Edward Qian pointed out in his 2011 paper "Risk Parity and Diversification" that having similar Sharpe ratios among assets is a prerequisite for risk parity.
Introducing leverage can resolve this contradiction. Although returns are a function of risk, different assets have different risk-return characteristics. Leverage has a "scaling" function, applying leverage to low-volatility assets adjusts the expected returns of various assets to an 8% level, resulting in a new set of "assets"—with different volatilities but all yielding 8%.
Furthermore, by allocating weights through the "parity" process of overweighting low-volatility assets and underweighting high-volatility assets, we achieve a final return of 8%, a volatility of 10%, and a Sharpe ratio of 0.8 for the portfolio. The portfolio achieves higher returns under the same Sharpe ratio, with actual positions of each asset being more diversified, and risk contributions being equal (risk contribution = asset weight × volatility) Step Four: "Dynamic Risk Rebalancing" refers to adjusting asset weights in the next period by recalculating the volatility and correlation of each asset to maintain risk parity.
PART4 Review of All-Weather Historical Performance
First, let's review the historical performance of Bridgewater's All-Weather strategy. Over a long historical dimension, Dalio, R (2015) [8] demonstrated the historical backtesting results of the All-Weather strategy from 1946 to 2015. From a full-time perspective, the All-Weather annualized return is 10.4%, with an excess return of 1.9% per year compared to the 60/40 portfolio. During the long-term rising interest rate period from 1946 to 1981, the excess return was slightly inferior, with an excess return of 1.1% per year compared to the 60/40 portfolio; after 1981, as interest rates declined, the All-Weather strategy entered a more comfortable zone, with an excess return of 2.6% per year compared to the 60/40 portfolio, and its absolute annualized return was 12.1%, also recording a Sharpe ratio of 0.84 during that period.
Among them, the period from July 2007 to August 2011 is important evidence of the All-Weather strategy withstanding the crisis test, and it is precisely this excellent performance after the crisis that has increased the attention on risk parity. According to disclosures on Bridgewater's official website [9], during this period, the cumulative total return of the All-Weather asset portfolio was 43.2%, while global stocks were still in negative returns (-17.2%), and traditional asset portfolios only achieved a slight positive return of 1.4%.
Although there has been little direct public information released regarding the performance of Bridgewater's All-Weather strategy in recent years, based on the allocation ratio experience recorded by Tony Robbins in 2014 [10] (30% exposure to U.S. stocks, 40% in long-term U.S. Treasuries, 15% in intermediate-term U.S. Treasuries, and 7.5% each in gold and other commodities), we can roughly simulate the performance of the All-Weather portfolio. The overseas ETF website "lazyportfolioetf.com" also directly provides similar results.
This website uses five overseas ETFs to replicate the All-Weather portfolio. The representative ETFs for U.S. stocks, long-term U.S. Treasuries, intermediate-term U.S. Treasuries, gold, and commodities are Vanguard Total Stock Market (VTI), iShares 20+ Year Treasury Bond ETF (TLT), iShares 3-7 Year Treasury Bond ETF (IEI), SPDR Gold Trust (GLD), and iShares Commodity Index (DBC)
First, let's look at the results from the lazyportfolioetf website. As of the end of January 2025, the simplified all-weather portfolio's compound annual growth rate over the past ten years is 4.46%, which is lower than the average annual compound return of 7.68% over the past thirty years. The main reason for the recent decline in compound returns is the rise in interest rates, which has caused U.S. Treasury ETF yields to drag down the portfolio. The long-term U.S. Treasury has a compound return of only -2.03% over the past ten years, -7.38% over the past five years, and -5.48% over the past year.
Meanwhile, gold and U.S. stocks contributed more to the return enhancement, with compound returns of 37.2% and 26.2% respectively over the past year. Correspondingly, the portfolio's annualized volatility has increased from 7.48% over the past thirty years to 8.45% over the past ten years, and the Sharpe ratio has decreased from 0.72 over the past thirty years to 0.33 over the past ten years.
Next, we also attempt to construct an overseas version of the all-weather portfolio using the empirical values provided by Dalio, and change the stock and intermediate U.S. Treasury representatives to examine the robustness of the results. Specifically, we replace Vanguard Total Stock Market (VTI) with SPDR S&P 500 ETF Trust (SPY) and iShares 3-7 Year Treasury Bond ETF (IEI) with iShares 7-10 Year Treasury Bond ETF (IEF). As of the end of January 2025, the self-constructed all-weather portfolio (with changes to the U.S. stock and intermediate U.S. Treasury representatives) has a compound annual growth rate of 4.18% over the past ten years, which is close to that of lazyportfolioetf.com. Recent returns have also significantly declined due to the drag from U.S. Treasury ETFs, with a compound return of only 1.9% over the past five years, -2.9% over the past three years, and a recovery to 12.5% over the past year. Observing the detailed items, we can also see that the recovery in returns over the past year is mainly due to the reduced drag from U.S. Treasury ETFs, with U.S. stocks contributing a 23.4% increase and gold contributing 46.4%.
In terms of drawdown, the self-constructed portfolio shows a maximum drawdown of 23.8% over the past decade, occurring in October 2022 during the Federal Reserve's interest rate hike cycle, which is also similar to the results from lazyportfolioetf.com. In contrast, "lazyportfolioetf.com" can provide a longer time dimension for examination, with portfolio performance tracing back to the 1930s during the "Great Depression" in the United States
According to lazyportfolioetf.com, the largest drawdown in nearly thirty years also occurred after the Federal Reserve began its current interest rate hike cycle in 2022, with a drawdown reaching as high as 20.58%; followed by a drawdown of around 11% during the financial crisis. Since the end of 2022, the drawdown of the all-weather portfolio has significantly eased, continuously rebounding for about 28 months. From the 2008 financial crisis to the current drawdown in 2022, the annual maximum drawdown of the portfolio has generally been controlled within 8%.
Looking back over a longer history (approximately 154 years since 1871), the current drawdown is the second largest in history, with the first being during the Great Depression in the United States (September 1929 - May 1932), which had a maximum drawdown of 37.02%. This shows that the all-weather strategy is not always effective. The fluctuations and drawdowns around 2022 have significantly increased, which may be related to the rise in U.S. interest rates again.
After reviewing 154 years of history, we should more dialectically assess the all-weather strategy. Its acclaim as the "Holy Grail" and "the pearl on the crown" after the 2008 financial crisis has strong "temporal characteristics": on one hand, the 2008 financial crisis prompted investors to further reflect on the lack of emphasis on risk, with risk management being more important than performance management in the entire portfolio optimization process, and risk parity strategies becoming a concrete expression of this investment philosophy; on the other hand, after the financial crisis, the U.S. initiated quantitative easing, entering a macro environment of "low interest rates, low inflation (relatively mild), and low growth," which concealed the risks of "risk parity" while providing the most comfortable growth soil.
However, with the tightening of the Federal Reserve's monetary policy, the outbreak of the pandemic, and the upward trend of U.S. Treasury yields post-pandemic, the all-weather strategy has also undergone an adjustment period.
Combining this with the performance during the Great Depression in the 1930s, we can more profoundly understand that the premise of the "all-weather strategy" is that assets exhibit rotational performance, implying the assumption of "when the East is not bright, the West shines." If stocks, bonds, and commodities all perform poorly, then the overall portfolio will also face significant drawdowns As mentioned in Bridgewater's January 2024 report "The Big Picture: Back to the Future," the current environment is significantly different from the past two decades, resembling more distant historical periods—specifically, the return of interest rates and inflation. The significant boost to the overall BETA returns of equity risk assets from the previous overseas low-interest-rate environment will likely return to normal.
PART5 Review of the Historical Performance of "Risk Parity Funds"
If we broaden our perspective to the historical performance of other "risk parity funds," we find that it is not only Bridgewater's "All Weather Fund" that follows similar patterns; AQR, PanAgora Risk Parity, and even the S&P 500 Risk Parity Index [14] exhibit similar trends:
A "highlight moment" following the 2008 financial crisis;
Underperformance compared to the US 60/40 portfolio (60% S&P 500 Index, 40% US Treasury Total Return Index) from 2013 to 2015;
A rebound in 2016-2017;
A return to mediocrity from 2018 to 2024.
Specifically, we compared the S&P 500 Risk Parity Index with the classic US 60/40 portfolio (60% S&P 500 Index, 40% US Treasury Total Return Index) since 2004, and found that consistent with Bridgewater's All Weather strategy's strong performance post-financial crisis, the S&P 500 Risk Parity Index also significantly outperformed the US 60/40 and pure equity portfolios from 2009 to 2012, with annual excess returns (compared to the US 60/40 portfolio) of 3.77%, 15.27%, 8.91%, and 5.86%, totaling 33.81%.
With the Federal Reserve indicating in May 2013 that it might reduce its bond-buying program, market panic selling of US Treasuries led to rising interest rates, causing the risk parity index to significantly underperform the US 60/40 portfolio by nearly 12 percentage points that year, raising doubts about its "sacred" status. In the context of the Federal Reserve exiting QE and entering a tightening cycle in 2014-2015, the risk parity index still did not outperform the US 60/40 portfolio. Although the Federal Reserve was still in a rate-hiking cycle in 2016-2017, US Treasury rates had already risen significantly during the prior phase of tapering and rate hike expectations, leading to a moderate decline in rates during that period, which allowed the S&P 500 Risk Parity Index to rebound, recording annualized returns of 13.81% and 10.81%. However, since 2018, with the impact of the pandemic and the Federal Reserve restarting rate hikes, the risk parity index has not experienced a favorable environment, consistent with the aforementioned All Weather strategy. The pandemic temporarily increased the volatility of multi-assets such as stocks and bonds, and the simultaneous asset pullback allowed risk parity to achieve high Sharpe ratios through diversification; however, the Federal Reserve's new round of rate hikes put pressure on the risk parity strategy, which is sensitive to interest rate risks
Combining the risk parity concept mentioned above, we can see that the process of "overweighting low-volatility assets and underweighting high-volatility assets" in the operation of risk parity strategies inherently has the function of "reducing volatility." However, a simple risk parity strategy relies on the premise that asset long-term returns are positive. When all assets experience a significant increase in volatility due to exogenous shocks or when interest rates rise significantly (beyond expectations), the simple risk parity strategy is relatively fragile and carries a risk of drawdown.
As a practical strategy, the risk parity strategy is not a "one-size-fits-all" or "set it and forget it" approach, nor is it completely "outdated" or should it exit the historical stage; it still requires a suitable market and macro environment. As a concept for portfolio optimization, its foundational framework still holds profound significance for investors in understanding and managing portfolio risks, although the methods of risk measurement and optimization paths can still be further improved.
PART6 The applicability of risk parity strategies in China seems to be increasing
First, as China's economy enters a new stage of high-quality development, the elasticity of economic growth, inflation, and monetary cycles has become smoother. The past characteristics of interest rate "cyclical goods" have diminished, and the volatility of both risky and risk-free assets may converge. In this environment, domestic risk parity strategies do not need to rely heavily on leverage and will allocate more weight to risky assets, thereby increasing the overall expected return potential.
Second, with the continuous development of domestic financial markets, there has been significant progress in asset selection diversification and financial instrument variety, all of which are conducive to the application of risk parity strategies and all-weather strategies;
Third, with the rise in global macroeconomic uncertainty and changes in wealth management market characteristics, there is a greater demand for investment to diversify risks (placing more emphasis on the "Sharpe ratio"), making the "volatility reduction" function of risk parity attractive.
We used two sets of empirical cases to find that over the past decade, timing and stock selection have been more significant than allocation in China; however, this may change in the future.
Using performance data from 18 asset classes from 2015 to 2024, we initially constructed the efficient frontier of major asset classes using historical annualized returns and volatility as model parameters. In this, we assumed that the investor's utility function is quadratic, with a risk aversion coefficient of 2, a target volatility of 2%, the portfolio can allocate risk-free assets, the annualized risk-free return is set at 2%, and short selling is allowed. The point of tangency between the Capital Market Line (CML) and the efficient frontier of major assets represents the market portfolio M with the maximum Sharpe ratio
From the results of the intersection combination, in an assumed environment where allocation can be freely configured, the optimal Sharpe ratio for a portfolio spanning global stocks, bonds, commodities, and currencies is 3.63. The top five weighted assets are China bonds, gold, US dollars, emerging market valuations, and US stocks, corresponding to the weights of the China Government Bond 10-Year Index, London Gold Spot, US Dollar Index, India Sensex30, Vietnam VN30, and S&P 500, which are 100%, 28.4%, 25.9%, 25.9%, 13.6%, and 13.1% respectively; while the weights of Chinese equity assets such as the CSI 300, CSI 2000, and Hang Seng Index are relatively lower, at 10.5%, 0.04%, and -0.02% respectively.
This means that in a scenario where short selling is allowed, the portfolio with the highest Sharpe ratio in the past decade primarily invests in China bonds, gold, US dollars, emerging market countries, and US stocks; while the risk-return ratio of domestic A-shares and Hong Kong stocks is relatively lower, requiring more proactive investment (stock selection and timing).
However, it is worth noting that this conclusion is based on historical data and can only represent the past. If the returns and volatility of Chinese assets change in the future, or if their correlation with other markets and assets changes, the above patterns will also change.
Another comparative empirical data between the Chinese and US markets can illustrate this point.
We use Wind All A and S&P 500 as representatives of A-shares and US stocks, respectively, designing two testing dimensions: annual timing and monthly timing. For each frequency level, two schemes are designed. Taking annual timing as an example, assuming an annual investment of 10,000 yuan, Scheme One is to buy at the highest point of that year, which corresponds to completely incorrect timing; Scheme Two is to buy at the lowest point of that year, which corresponds to completely correct timing. Monthly timing is similar, also constructing schemes for perfect correct timing and incorrect timing.
In the fifteen years from 2011 to 2024, the annualized return of the A-share market with perfect timing is 3.9%, while the annualized return with completely incorrect timing is 1.3%, resulting in a difference of 2.6%, with a ratio of 2.94 times. In other words, over the past fifteen years, the return difference between correct and incorrect timing in A-shares is significant, with the return from correct timing being 2.94 times that of incorrect timing. If the timing frequency is raised to monthly, the annualized return from perfect timing is 2.5%, while the annualized return from incorrect timing is 1.0%, making the return from correct timing 1.29 times that of incorrect timing.
If the peak in 2015 was not exited in time, then during the period from 2015 to 2024, the annualized return from completely incorrect timing would be -0.5%, and the annualized return from perfect timing would also drop to 2.8%, with the difference rising to 3.3% Correct timing can avoid losses. If the timing frequency rises to monthly, the annualized return of perfect timing is 1.3%, while the annualized return of incorrect timing is 0.5%, making the return of correct timing 2.78 times that of incorrect timing.
In contrast, during the period from 2011 to 2024, the difference in timing for the S&P 500 is not significant. First, looking at the two schemes of annual timing, the annualized return of correct timing is 2.0% higher; considering the magnitude of U.S. stock returns, the annualized return of correct timing is 1.3 times that of incorrect timing, which is much smaller than the 2.94 times in the A-share market.
Moreover, if we extend the time frame, during the period from 2000 to 2024, even if one cannot avoid the internet bubble of 2000, the difference between correct and incorrect timing is only 1.4%, with the annualized return of correct timing being only 1.3 times higher than that of incorrect timing. If the timing frequency rises to monthly, the return difference between correct and incorrect timing is only 0.3%, with the annualized return of correct timing at 5.6%, which is close to the 5.3% of incorrect timing.
Similarly, observing since 2015, it can still be seen that the return of perfect timing in the U.S. stock market is only 1.5 times that of completely incorrect timing. Based on past historical experience, the environments of the equity markets in China and the U.S. are quite different, and different strategies and investment philosophies have varying applicability in the two markets.
This may be related to the relatively higher volatility of the A-share market in the past. The market does not exhibit a relatively sustained one-sided trend; correspondingly, the domestic bond market is exactly a mirror, where the significance of allocation outweighs timing due to the persistence of one-sided trends.
From the perspective of annual amplitude (annual maximum index point - annual minimum index point / annual minimum index point) reflecting volatility, the annual volatility of the domestic equity market is more pronounced than that of the S&P 500. Since 2014, the domestic ChiNext has had higher volatility than the U.S. stock market in all years except for 2020.
Therefore, although Gary P. Brinson, who created the Brinson performance attribution model, pointed out in his 1986 paper that asset allocation explains an average of 91.5% of the total quarterly returns of investment portfolios (analyzing the performance of 82 large pension plans from December 1977 to December 1987);
For A-share investors, whether through active stock selection or active timing, remains a core issue and an important source of excess returns.
However, in our previous report "The Macroeconomic Logic and Timing System of High Dividend Strategies," we pointed out that from a medium to long-term perspective, as domestic economic growth no longer solely pursues speed, and with the manufacturing supply capacity remaining strong under the background of a manufacturing powerhouse, the future elasticity of industrial product prices and the overall elasticity of the monetary cycle may both be relatively moderate. This will correspond to a lower apparent interest rate center, lower growth, and lower inflation, entering a macroeconomic environment similar to that faced by the United States before the pandemic in 2020.
This period (post-financial crisis) marks the beginning of a gradual upward trend in the valuation center of U.S. stocks, continuously deviating from historical average positions, as well as a period where risk parity and other leverage-dependent allocation models gained recognition and significant development.
If a macro combination of "three lows" appears domestically in the future, and the attributes of past interest rate "cyclical products" diminish, the volatility of both risk assets and risk-free assets in China may converge, potentially leading to a better risk-return ratio for domestic asset portfolios. On one hand, this reduces the necessity for high-frequency timing similar to U.S. stocks after 2008; on the other hand, it enhances the applicability of multi-asset allocation and risk parity strategies domestically, akin to the development of quantitative allocation models in the U.S.
PART7 Preliminary Construction of the Risk Parity Model
In this section, we will initially attempt to construct a basic risk parity model for traditional major asset classes and extend and implement it using the increasingly rich domestic ETFs.
(1) Asset Selection, Risk-Return Characteristics, and Correlation Analysis
In terms of asset selection, we first consider the "CSI 300 Index, Hang Seng Index, S&P 500 Index, China Bond Corporate Bond Total Index, COMEX Gold Futures Closing Price, and South China Comprehensive Index" as the basic global major asset pool, covering six major markets: A-shares, Hong Kong stocks, U.S. stocks, China bonds, gold, and commodities.
Moreover, ETFs are index investment tools that essentially construct a portfolio of securities that track index changes by replicating the underlying index, characterized by low cost and high transparency, allowing investors to conveniently allocate to these assets by buying and selling a single ETF. The domestic ETF market began in 2004, developing slowly in its early years, but has entered a "fast lane" since 2020. As of February 15, 2025, the number of products in the domestic ETF market has reached 1,079, with a net asset value of 3.83 trillion. Coupled with the new "Nine National Policies" supporting ETFs in April 2024, the variety of domestic ETFs will become increasingly rich, serving as an effective tool for potential major asset allocation.
Therefore, we will also change traditional major assets to "ETF products" to test the risk parity model, and given that the KWEB ETF representing the China Concept Internet Index and REITs began developing after 2020, we have delineated two sets of "basic" and "expanded" versions for the ETF asset pool The "Basic Version" ETF asset pool involves relatively few assets, but the backtesting period can be traced back to January 1, 2015, mainly consisting of 7 categories: CSI 300 ETF, Huatai-PB Dividend ETF, Bosera S&P 500 ETF, Treasury ETF, Urban Investment Bond 500 ETF, Gold ETF, and CITIC Prudential Global Commodity Theme ETF, covering seven categories including A-shares, A-share dividends, US stocks, interest rate bonds, credit bonds, gold, and commodities.
The "Extended Version" ETF asset pool adds four categories on top of the "Basic Version" framework: Hong Kong stocks, US-listed Chinese stocks, convertible bonds, and CSI REITs. The representative ETFs are Huaxia Hang Seng ETF, KWEB China Internet ETF, and Convertible Bond ETF; additionally, given that domestic ETFs based on REITs are still being explored, we temporarily use the "CSI REITs Total Return Index" as a proxy indicator for domestic REITs ETFs in the "Extended Version" ETF. Due to the addition of products that have started in recent years, the backtesting period for the "Extended Version" ETF can only be traced back to August 2021.
Furthermore, we observed the risk-return characteristics of various varieties in different historical stages.
First, in the basic global major asset index pool, from 2005 to February 5, 2025, the best risk-return ratio is the China Bond Corporate Bond Total Index, with a Sharpe ratio of 1.90. Its annualized return is 5.1%, ranking in the middle, but the annualized volatility is only 1.6%, and the maximum drawdown is 8.6%, making it the most controllable asset among various medium-risk assets. Due to a certain upward trend in gold in recent years, its risk-return ratio ranks second best, with a Sharpe ratio of 0.47. The risk-return ratios that rank relatively low are the South China Comprehensive Index, CSI 300, and Hang Seng Index. This result is consistent with the findings in the first part of MVO.
Next, looking at the ETF asset pool. From 2015 to February 5, 2025, the best risk-return ratio is domestic credit bonds, with the Urban Investment 500 ETF having a Sharpe ratio of 0.99 and a maximum drawdown of 5.5%. Next are US stocks and gold. When the review period is shortened to nearly ten years, the Sharpe ratios for US stocks and gold increase to 0.69 and 0.64, respectively, while the Sharpe ratios for CSI 300 ETF and commodity ETFs drop to around 0. Among A-share equity assets, the Sharpe ratio for dividend assets is significantly higher than that of CSI 300, but still lower than that of Treasury bonds and credit bonds.
If the review period is further shortened to since August 2021, the best risk-return ratio is for the Gold ETF, with a Sharpe ratio of 1.34, where the annualized volatility is still 11.7%, far higher than the 1.6% for interest rate bonds, 2.3% for credit bonds, and 9.5% for convertible bonds, but its annualized return is high at 17.7%, significantly higher than other assets, second only to the global commodity theme ETF during the same period The Sharpe ratio of the US stock market continues to rise slightly to 0.71, while the CSI 300 ETF has fallen to -0.4. The Sharpe ratio of dividend assets has further expanded its advantage over the CSI 300, rising to 0.28.
Further examination of the correlation of major asset classes. From the derivation in the appendix, it can be seen that the variance-covariance matrix of asset returns is a core input parameter of the risk parity model. The measurement of correlation can use different statistical periods of returns (daily, weekly, monthly, etc.), as well as different rolling window periods (3 months, 6 months, 9 months, etc.). The short-term dynamic correlation we examine mainly looks at the degree of association of daily returns between assets within a 6-month rolling window; long-term correlation averages the daily return correlations over a rolling 6-month period.
From a long-term correlation perspective, from 2005 to February 5, 2025, Chinese assets not only show a negative correlation between stocks and bonds (the correlation between the CSI 300 index and the China Bond Corporate Bond Total Index is -0.28), but also negative correlations between bonds, Hong Kong stocks, and the China Bond (the correlation between the China Bond Corporate Bond Total Index and the Nanhua Composite Index, and the Hang Seng Index is -0.35 and -0.33, respectively). Correspondingly, A-shares have a certain degree of linkage with Hong Kong stocks and domestic commodities, with the correlation between the CSI 300 and the Hang Seng Index being 0.54, and the correlation with the Nanhua Composite Index being 0.28. Hong Kong stocks not only move in tandem with A-shares but also have a correlation of 0.61 with US stocks.
From 2015 to February 5, 2025, the negative correlation between domestic stocks and bonds continues to be maintained, with the correlation of the CSI 300 ETF with the government bond ETF and the urban investment bond ETF being -0.28 and -0.16, respectively;
Domestic dividend assets have an average correlation of 0.76 with the market over the past decade, stronger than the correlation of the government bond ETF and the urban investment bond ETF (0.56);
The gold ETF has a weak correlation (close to 0) with the domestic market, dividend assets, credit bonds, and US stocks during this period, while having a slightly higher correlation with the commodity index and domestic interest rate bonds, at 0.11 and 0.26, respectively.
Looking back at the period further shortened to since August 2021, the domestic market has shown a stable negative correlation with government bonds, with the correlation between the CSI 300 index and the government bond ETF being -0.26, which is basically similar to the previous two periods;
The correlation between the domestic market and corporate bonds has decreased to around 0, but it is highly positively correlated with convertible bonds (the interval correlation reaches 0.66);
The correlation between Hong Kong stocks (Huaxia Hang Seng ETF), US-listed Chinese stocks (KWEB China Internet ETF), and A-shares (CSI 300) reaches 0.72 and 0.76, respectively; the two US-listed Chinese stocks and Hong Kong stocks are almost highly correlated, with the correlation between KWEB China Internet ETF and Huaxia Hang Seng ETF reaching 0.92; The correlation between domestic dividend assets and the A-share market has dropped to 0.41;
Gold ETFs still have the highest correlation with commodity indices in recent years (0.47), while their correlation with other assets remains relatively low (<0.20);
From the perspective of the entire range, the correlation of CSI REITs with most assets is negative, and there is no correlation with the commodity index.
From the rolling 6-month window, it can also be seen that the domestic market and bonds have generally shown a negative correlation since 2018, with a significant weakening of the negative correlation between 2020-2021, and a "seesaw" return after 2022. Among them, the negative correlation between government bonds and stock indices is more pronounced, while the correlation between credit bonds and stock indices generally runs between [-0.2, 0.2]. In contrast, dividend assets, which have some "bond characteristics," still maintain a positive correlation with the market overall, although the positive correlation has significantly weakened after 2020, and in recent years has oscillated around a lower correlation center than before 2020.
The correlation between the domestic market and the domestic commodity index fluctuates greatly. From 2016-2020, 2022-2023, and since the second half of 2024, there has been a strengthening of positive correlation; however, the correlation with COMEX gold is not high, maintaining between [-0.2, 0.2] for many years. From the ETF perspective, the sequence of changes in rolling correlation is more cyclical, and since 2024, there has been a trend of strengthening positive correlation between commodities and stock indices.
Due to the positive linkage between stock indices and commodities, commodities and domestic bonds are more of a reverse linkage, with a more pronounced negative correlation with interest rate bonds, followed by credit bonds, and then dividend assets (showing weak positive correlation).
The correlation between the domestic market and U.S. stocks also fluctuates greatly, with a significant weakening of positive correlation since 2020. This is more evident from the ETF perspective; since the second half of 2024, the correlation between the CSI 300 ETF and the Bosera S&P 500 ETF has even turned negative.
After including the KWEB ETF, which represents the Chinese concept internet index, and the CSI REITs total return index, it can be seen that the Chinese concept internet has a strong and stable positive linkage with the domestic CSI 300 ETF, while the correlation with government bonds is basically stable at [-0.3, -0.2] in negative correlation; the CSI REITs total return index has not shown high correlation with pure stock indices or pure government bonds since 2024.
(2) Strategy Backtesting Performance and Comparison
Based on the rolling correlation (covariance matrix) of the above three sets of asset returns, we use equal weights for all assets as the initial weights, and then perform nonlinear optimization on the risk parity objective function to solve for the numerical solution weights that equalize risk contributions. The detailed optimization process and principles can be found in Appendix 4.1. Additionally, given the changing market environment, we rebalance on a monthly basis to ensure that we generally remain in a risk parity state.
Furthermore, to view the risk parity results more objectively, we designed two additional benchmark schemes: one is to operate all assets continuously with the initial equal weight, and the other is to directly use the inverse of the volatility of all assets over the past six months for weighting, without optimizing based on the correlations between assets, thus not considering the risk linkage and spillover between assets. This is the most straightforward implementation of "underweighting high volatility and overweighting low volatility."
From the backtesting results, although the model with simple equal weight allocation appears to achieve diversification, it has a higher annualized volatility and a Sharpe ratio significantly lower than that of the simple volatility inverse weighting and risk parity models. The risk parity model, in contrast, has the highest Sharpe ratio, win rate, and payout ratio, with this advantage becoming more pronounced since 2022:
For example, from 2022 to February 2025, the Sharpe ratio, win rate, and payout ratio of the original underlying assets under the equal weight scheme were 1.07, 51.4%, and 1.17 (profit-loss ratio), respectively; under the volatility-weighted scheme, they were 2.47, 58.9%, and 1.24 for the same period; while under the risk parity scheme, they were 3.37, 62.1%, and 1.35, respectively. If we extend the view to since 2009, the Sharpe ratio of the risk parity scheme for the original major asset classes is 1.79, higher than the simple equal weight of 0.62 and also higher than the simple volatility-weighted 1.62; the win rate also increased from 53% under equal weight to 58.3% under volatility weighting, further rising to 59.7% under the risk parity scheme; the payout ratio (profit-loss ratio) also increased.
If we observe the underlying ETF asset pool, from 2022 to February 2025, the Sharpe ratio, win rate, and payout ratio under the equal weight scheme were 1.51, 54.8%, and 1.08 (profit-loss ratio), respectively; under the volatility-weighted scheme, they were 1.96, 58.9%, and 1.10 for the same period; while under the risk parity scheme, they were 2.04, 60.6%, and 1.05, respectively.
Further extending to the expanded ETF asset pool, from 2022 to February 2025, the Sharpe ratio, win rate, and payout ratio under the equal weight scheme were 0.88, 52.6%, and 1.07 (profit-loss ratio), respectively; under the volatility-weighted scheme, they were 0.61, 54.6%, and 1.04 for the same period; while under the risk parity scheme, they were 0.89, 57.4%, and 0.99, respectively.
Therefore, overall, the risk parity model may not achieve returns exceeding those of simple equal weight and volatility inverse weighting, but it can generally maintain a higher Sharpe ratio. Combined with the win rate and payout ratio, risk parity represents a direction that improves both the win rate and payout ratio compared to traditional allocation models
Observing the maximum drawdown periods of each scheme over the entire interval, it can be seen that the periods of significant drawdown for risk parity were in the third quarter of 2015 and the fourth quarter of 2022. From the performance of annual return volatility, the years with relatively large drawdowns and low Sharpe ratios for risk parity were 2011, 2013, 2018, and 2022, where the Sharpe ratios were all below 0.2, and some were even negative.
Combining this with the net value curve, the unfavorable asset scenarios for the basic risk parity model are firstly multi-asset correlated pullbacks (the most typical being simultaneous declines in stocks and bonds, or stocks and commodities), and secondly, excessive volatility in a particular asset. The former is because risk parity is underweighting high volatility and overweighting low volatility; if assets resonate downwards, the overweighted objects may not have cost-effectiveness but merely result in a passive increase in positions; the latter is due to the assumption in risk parity that asset volatility (risk) remains relatively stable during the forecast period and does not significantly deviate from past volatility ranges.
The year 2011 saw a decline in inflation, the European debt crisis, tightening liquidity, and a retreat in risk appetite, leading equities, Hong Kong stocks, and commodities into a downward cycle; in 2013, both stocks and bonds adjusted under the "money shortage"; in the second half of 2015, the stock market underwent a significant pullback; in 2018, the stock market adjusted significantly under real economy deleveraging; and in 2022, the stock market was sluggish due to pandemic disruptions and the Federal Reserve's interest rate hikes.
From the beginning of the month weightings, whether based on traditional major asset classes for risk parity or on the asset pools of basic and extended ETFs, the relatively stable net value of risk parity still benefits from the relative overweighting in bond assets. Among these, the allocation weight of government bond ETFs is higher than that of credit bond ETFs, with an average weight of 43% and 31.4% respectively from 2015 to present. Next are gold assets, with an average weight of 8.3% from 2015 to present; global commodity ETFs average 4.4%; dividend ETFs average 3.9%, while the average proportion of the CSI 300 ETF is 3.6%, and the average weight of the S&P 500 ETF is 5.3%. Thus, compared to the proportions given by Dalio of "30% U.S. stocks, 40% long-term U.S. Treasuries, 15% medium-term U.S. Treasuries, and 7.5% each for gold and other commodities," the total proportion of bond assets in domestic risk parity reaches 74.4%, making it a more core underlying asset.
If we observe the weights of various assets in the extended ETF asset pool since 2022, the key positions remain in bonds, with the average weight of government bond ETFs being 43.6% since 2022, and even at its lowest, the weight was 25.9%; next is corporate bond ETFs, with an average weight of 28.4%; the average weights for CSI REITs and gold are 5.1% and 7.5% respectively, while the remaining seven assets account for a total of 14.4% This indicates that the domestic ETF risk parity scheme will have a lower weight due to the high volatility of equity assets, with corresponding bonds becoming the absolute dominant base.
On the other hand, it also means that simple risk parity applications in the domestic market will be exposed to greater interest rate risks, making them more vulnerable to rising interest rates.
(3) Strategy Comparison After Introducing Leverage and Scaling Target Volatility
As mentioned earlier, the unleveraged version of the risk parity portfolio has a high Sharpe ratio but lacks attractive returns. Utilizing leverage is an essential means to enhance strategy flexibility, balancing both Sharpe and returns. Particularly, as evidenced in the empirical cases of ETFs in China, if leverage is not used, over 70% of the portfolio is allocated to low-volatility bond assets (approximately 40% in government bonds and 30% in credit bonds), with the source of portfolio Sharpe mainly coming from low volatility (around 2.0%), making it necessary to enhance the attractiveness of returns.
There are two ways to introduce leverage: one is to leverage the assets first and then achieve parity; the other is to achieve parity first and then leverage the portfolio.
The former refers to the approach mentioned earlier by Bridgewater. Leverage is applied to low-volatility assets, such as leveraging bonds, to change the risk-return ratio of low-volatility assets and equalize the expected returns of various assets. Then, parity is achieved based on different volatilities. Due to the "scaling" function of leverage, while the returns of low-volatility assets increase, their volatility also increases, thereby reducing the bond allocation. This can be done through parity methods or optimizing the Sharpe ratio under new expected returns.
The latter is simpler and more direct, which is to directly leverage the portfolio after achieving parity, obtaining funds from the money market (by pledging bond assets for repurchase) to continue buying the portfolio after parity. The cost of funds is related to market financing costs, closely linked to short-term interest rates and regulatory policies. Leverage plays a "scaling" function on the portfolio, amplifying the returns and volatility of the overall "low-volatility asset" portfolio.
This article adopts the latter method of introducing leverage and considers the actual situation in the domestic market, limiting leverage to the range of 80% to 140% [15]. We tested the strategy effects when the target volatilities are 2%, 3%, 4%, 5%, and 6%. Due to leverage constraints, the final actual annualized volatility of the portfolio cannot fully match the set target volatility, but there is a monotonically positive correlation. At the same time, for comparison, we also examined the risk parity strategy without setting a target volatility (i.e., without introducing leverage) as a benchmark.
From the results, it can be seen that in the three asset pools, the annualized return and annualized volatility of the portfolio both increase with the rise in target volatility, while the Sharpe ratio slightly declines.
Under the risk parity scheme of basic asset class indices, since 2009, from the basic scheme without a target volatility to a target volatility of 6%, the portfolio's annualized return increased from 6.6% to 7.8%, the annualized volatility rose from 2.6% to 3.3%, and the Sharpe ratio decreased from 1.79 to 1.74.
Under the basic ETF risk parity scheme, since 2015, from the basic scheme without a target volatility to a target volatility of 6%, the portfolio's annualized return increased from 5.1% to 6.4%, the annualized volatility rose from 2.9% to 4.8%, and the Sharpe ratio decreased from 1.07 to 0.92. If further shortened to since 2022, with the increase in target volatility, the portfolio's annualized return rose from 6.0% to 8.3%, the annualized volatility increased from 2.0% to 3.3%, while the Sharpe ratio only decreased from 2.04 to 1.93. The extended ETF scheme also showed similar effects, with annualized returns increasing by 0.4 percentage points, annualized volatility rising by 1.1 percentage points, and the Sharpe ratio declining by 0.2.
Although the introduction of leverage brings new risks—sensitivity to short-term funding rates, it also sacrifices part of the risk-return ratio. However, for investors with a certain risk appetite, the leverage mechanism allows them to achieve higher investment returns through risk parity within the range of risk they are willing to bear.
Finally, a brief summary of the re-understanding of the all-weather strategy.
Each financial crisis prompts investors to reflect on the importance of risk, making risk management more crucial. The risk parity model and all-weather strategy are expressions of this investment philosophy.
After the 2008 financial crisis, the United States began quantitative easing, and the overseas macro environment entered the "three lows" era of "low interest rates, low inflation, low growth," providing the most comfortable growth soil for the "risk parity" strategy. With the post-pandemic inflation center rising, the Federal Reserve raising interest rates, and the significant upward trend in U.S. Treasury yields, the all-weather strategy has also undergone an adjustment period. Combined with the situation during the overseas Great Depression and the historical testing of basic risk parity in China, we understand that the premise of the "all-weather strategy" is that assets exhibit rotation performance, and long-term returns are positive.
In short, this strategy implies the premise assumption that "everything has its time" and "if the East is not bright, the West is bright." If the performance of stocks, bonds, and commodities is poor, the effectiveness of the strategy will also decline significantly. From the above understanding, during periods when domestic total growth fluctuations gradually stabilize, inflation elasticity decreases, and interest rate centers are not high, the applicability and practicality of the risk parity strategy may increase Risk Warning: First, the regression model construction is based on historical data, which may have errors in the event of significant economic shocks or market changes in the future; second, the model still needs to conduct sensitivity analysis on various parameters to improve the robustness of the strategy; third, there may be events at the fundamental level that significantly change expectations, such as the domestic real estate policy effects falling short of expectations; the impact of the new round of local government debt management exceeding expectations; subsequent fiscal policy strength exceeding expectations, etc.
Appendix:
(1) Specific derivation process of risk parity model modeling
The risk measure of ordinary risk parity is the volatility of the portfolio, which is measured by the standard deviation:
Where the marginal risk contribution (MRC) of various assets is:
If there are only 2 assets, then:
Similar derivation, this indicator measures the sensitivity of the i-th asset to the overall portfolio volatility. If the measure of "risk" is not the standard deviation, but VaR or CvaR, then the corresponding changes apply.
Sensitivity * Asset Weight = Total Risk Contribution (TRC):
Similar derivation, total risk is the sum of the risk contributions of various assets:
For N types of assets, it is:
The risk contribution ratio of each asset becomes the relative risk contribution (RRC):
The process of risk parity is to make the risk contributions of various assets equal, thus we have:
That is:
Extending this to N types of assets gives:
Thus, the risk contribution of each asset type accounts for one N-th of the total portfolio risk:
At this point, we can compare it to the simplest equal-weighted portfolio:
The above is the requirement for risk parity, and the optimal portfolio seeks to solve the following optimization problem—minimizing the sum of squared deviations of risk contributions from the target, where a smaller deviation indicates closer to risk parity:
In general, the risk parity model does not have an analytical solution and is usually a nonlinear numerical solution:
The model's inputs are the covariance matrix of N types of assets, the target volatility C of the portfolio, and the weights under optimal allocation are wi. Typically, risk parity strategies set a target volatility, as this can enhance returns through leverage.
If no target volatility is set, it means that the portfolio weights are optimized directly based on the equilibrium of overall risk contributions, allowing each asset to flexibly adjust risk parity during rebalancing according to market changes, rather than frequently trading to meet a specific target volatility.
Overall, this model does not require predicting returns and is relatively less sensitive to parameter changes.
(2) The relationship between risk parity optimization and MVO
Under the conditions where the returns of various assets are independent and have the same Sharpe ratio, ordinary risk parity is equivalent to the goal of maximizing the portfolio Sharpe ratio in MVO, meaning that the covariance matrix of the aforementioned N types of assets is a diagonal matrix. Specifically, under the same target volatility, the process of Markowitz maximizing the portfolio Sharpe ratio is:
The optimal Sharpe ratio means that each type of asset within the portfolio provides equal excess return per unit of risk to the overall portfolio, indicating that investors do not increase the overall portfolio's Sharpe ratio by reallocating funds to assets with a higher excess return-to-risk ratio, that is:
Under risk parity,
If the correlation of returns between assets is 0, then:
That is, when the covariance of assets is 0, risk parity is equivalent to a volatility inverse weighting strategy, which means a complete underweighting of high-volatility assets and an overweighting of low-volatility assets. Furthermore, substituting (2) into (1):
That is:
When asset returns are independent and the Sharpe ratios are the same, the risk parity portfolio is the combination with the optimal Sharpe ratio on the efficient frontier of Markowitz.
Author of this article: Chen Liqing S0260523080003, Source: Guo Lei Macro Tea House, Original title: "【GF Macro Chen Liqing】Interpretation of the 'All-Weather' Strategy"
Risk Warning and Disclaimer
The market has risks, and investment requires caution. This article does not constitute personal investment advice and does not consider the specific investment goals, financial situation, or needs of individual users. Users should consider whether any opinions, views, or conclusions in this article are suitable for their specific circumstances. Investment based on this is at their own risk