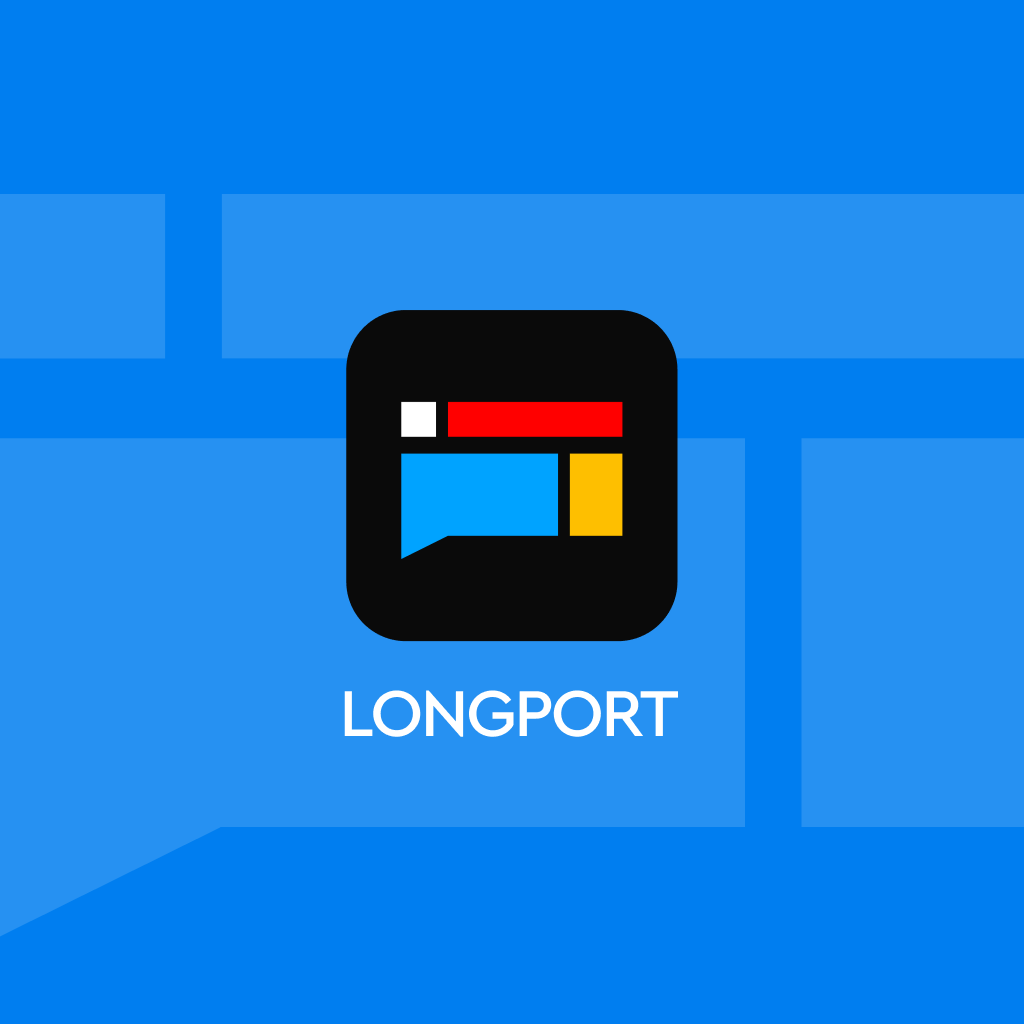
Is it useful? Financial institutions test DeepSeek!
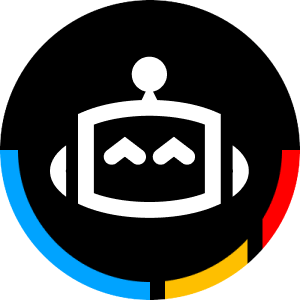
The application of the open-source AI large model DeepSeek in the financial industry is continuously expanding, with many financial institutions such as Bank Of Jiangsu and NCI trying it out. DeepSeek provides low-cost AI capabilities for small and medium-sized financial institutions, helping them improve efficiency in customer service and risk management. Since its launch on January 20, DeepSeek-R1 has received widespread attention for its powerful performance and open-source advantages, prompting financial institutions to accelerate local deployment and explore various application scenarios
The rapidly rising open-source AI large model DeepSeek is continuously expanding its "financial circle."
According to incomplete statistics, financial institutions including Bank of Jiangsu, SuShang Bank, Chongqing Rural Commercial Bank, insurance institutions like NCI, securities firms such as CITIC Securities and Guotai Junan, as well as public fund companies like Huatai-PineBridge and Franklin Templeton are all "trying out" DeepSeek, exploring relevant application scenarios.
In this wave of integrating DeepSeek, the presence of small and medium-sized financial institutions is everywhere. Some practitioners believe that DeepSeek may provide an opportunity for small and medium-sized financial institutions to "overtake on a curve." "Small and medium-sized financial institutions often face limited resources and insufficient technological accumulation. The open-source nature of DeepSeek offers them the possibility to catch up quickly. By adopting DeepSeek, small and medium-sized financial institutions can obtain advanced AI capabilities at a lower cost, thereby achieving significant improvements in customer service, risk management, and other areas," said Zhu Keli, executive director of the China Information Association and founding director of the National Research Institute for New Economy, in an interview with the Financial Times.
Institutions Compete to Layout, Applications Bloom in Multiple Points
On January 20, DeepSeek launched and open-sourced DeepSeek-R1. Based on its strong performance in tasks such as mathematics, coding, and natural language reasoning, as well as its low-cost and open-source advantages, DeepSeek-R1 attracted widespread attention both domestically and internationally upon release. Subsequently, various industries "rushed to board," and financial institutions accelerated the localized deployment of the DeepSeek series of models, with application scenarios blooming in multiple points.
For example, Postal Savings Bank will further explore more specialized service applications in financial scenarios based on the "Youzhi" large model, leveraging DeepSeek's technological capabilities. In the field of risk prevention and control, it will efficiently analyze and automatically generate case analysis reports, improving the efficiency and accuracy of suspicious point identification and enhancing proactive defense capabilities against fraud.
Guotai Junan stated that it has completed the localized deployment of the DeepSeek-R1 model and applied it to the "Junhong Lingxi" large model, empowering multiple core business scenarios such as intelligent Q&A, smart investment services, investment education, industry research, market analysis, and compliance risk control.
Beijing University Founder Life officially launched the intelligent business assistant "Fangling" based on the DeepSeek large model. Initially, the "Fangling" assistant will unlock the basic function of "knowledge intelligent retrieval," serving as an E-marketing intelligent assistant, providing agents with comprehensive answers covering basic insurance concepts, laws and regulations, regulatory policies, insurance product sales concepts, and trends in the insurance industry.
"The reason DeepSeek can quickly attract the attention of financial institutions is primarily due to its open-source characteristics," said Zeng Gang, chief expert and director of the Shanghai Financial and Development Laboratory, in an interview with the Financial Times. Unlike traditional closed AI models, DeepSeek provides users with greater freedom and customizability through open code and model architecture. This openness allows financial institutions to flexibly adjust the model's functions and performance according to their own needs, thereby developing solutions that are more aligned with business scenarios For example, banks can use DeepSeek to optimize risk assessment models, securities companies can enhance market prediction accuracy through it, and insurance companies can improve the automation of claims processes with its help.
Feedback from the industry indicates that DeepSeek demonstrates tremendous potential in enhancing service efficiency, upgrading risk control capabilities, improving operational efficiency, and innovating business models.
Suzhou Bank stated that by innovatively applying the DeepSeek-VL2 multimodal model and constructing an innovative system of "multimodal technology + mixed expert framework," it has achieved precise analysis of complex scenario materials such as nested tables and image data, raising the comprehensive identification accuracy of credit materials to over 97%. This technology has improved the efficiency of the entire credit review process by 20%.
Zhu Keli stated that as an open-source AI large model, DeepSeek brings unprecedented flexibility to the financial industry, allowing financial institutions to customize and optimize it according to their needs, thereby reducing reliance on external vendors. At the same time, DeepSeek is expected to address challenges faced by the development of large financial models, such as computing power and costs, by enhancing computational efficiency and lowering deployment costs through efficient algorithms and architectural design, enabling financial institutions to utilize AI technology more economically to improve service quality and risk management capabilities, and to create new business models.
Opportunities for Small and Medium-sized Institutions to Address Shortcomings
It is understood that compared to the tens of millions or even hundreds of millions of investments required for traditional large models, the cost of locally deploying DeepSeek by banks can be as low as less than one million yuan. Some financial practitioners believe that DeepSeek may provide a "shortcut" opportunity for small and medium-sized financial institutions, allowing more small and medium-sized banks to benefit.
"The training and deployment of traditional AI models often require enormous computing resources and financial investments, which are difficult for many small and medium-sized financial institutions to bear. The emergence of DeepSeek provides a new solution to this problem," Zeng Gang stated. Traditionally, the high threshold of financial technology has made it difficult for small and medium-sized institutions to compete, while the popularization of tools like DeepSeek may lower the barriers to technology use, enabling small and medium-sized institutions to acquire advanced technological capabilities at a lower cost. This "democratization of technology" helps narrow the gap between small and medium-sized institutions and large institutions. By deploying technologies like DeepSeek, small and medium-sized financial institutions can gain efficient data mining, analysis, and forecasting capabilities, allowing them to quickly obtain market insights and optimize decision-making processes, thus achieving breakthroughs in certain niche areas. Zeng Gang also pointed out that DeepSeek, as an emerging technological tool, may indeed help small and medium-sized financial institutions address shortcomings and gain some development opportunities. However, the "shortcut" may not be realistic, as large institutions using the same tools may have stronger capabilities. Additionally, small and medium-sized financial institutions face some challenges when using such technologies: on one hand, there are issues of data quality and privacy; the data accumulation of small and medium-sized institutions may be insufficient, with varying data quality, while also needing to address data privacy and compliance risks; on the other hand, there is the challenge of technological dependence and competitive pressure. Over-reliance on external technology vendors may lead to insufficient core competitiveness, and large institutions may quickly adopt similar technologies, further intensifying competition AI hallucination remains a common issue
Although generative AI has made significant breakthroughs, AI hallucination remains a common problem, and DeepSeek is no exception. A user shared on social media that when asking DeepSeek to "recommend a few newly opened popular stores," they received "fabricated lies"—DeepSeek invented places that do not exist, and the recommended addresses and selling points were all false.
When a reporter asked, "Why does it seriously spout nonsense?" DeepSeek provided the following response: As a large language model, DeepSeek, despite extensive data training and algorithm optimization, may still produce inaccurate or erroneous content. This phenomenon is mainly caused by several factors: data dependency characteristics, limitations of semantic associations, characteristics of expression mechanisms, and the stage of technological development. At the same time, it suggested users maintain a habit of verifying content in specialized fields, provide clearer contextual constraints, and use the latest knowledge retrieval functions to assist in judgment.
Zeng Gang stated that AI hallucination refers to the possibility of generative AI producing inaccurate or even false information when lacking real data support. In the financial sector, such issues can lead to serious consequences. For example, in risk assessment, AI may generate false risk predictions based on incorrect inferences, leading to decision-making errors; in customer service, AI may provide inaccurate financial advice, harming customer interests. This phenomenon not only affects the operational efficiency of financial institutions but may also trigger legal and reputational risks. Technical paths to address AI hallucination include introducing domain knowledge constraints, multimodal data verification, strengthening human-machine collaboration, and dynamic optimization and feedback mechanisms.
"Despite significant breakthroughs in generative AI, issues of AI hallucination, data security, and privacy protection still need attention," said Zhu Keli. When applying large models in the financial sector, a comprehensive data verification and error correction mechanism should be established to ensure the accuracy of AI-generated information. Additionally, data encryption and access control should be strengthened to ensure the security of data during transmission, storage, and processing. Furthermore, financial institutions should comply with relevant laws and regulations to ensure that customer privacy is fully protected. By combining technological means with institutional construction, the financial sector can effectively address these challenges while enjoying the conveniences brought by AI. Source: Financial Times Client, Original Title: "Is it Useful? Financial Institutions Test DeepSeek!" Reporter: Xu Beibei Editor: Duan Jiaxi
Risk Warning and Disclaimer
The market has risks, and investment requires caution. This article does not constitute personal investment advice and does not consider the specific investment goals, financial situation, or needs of individual users. Users should consider whether any opinions, views, or conclusions in this article align with their specific circumstances. Investing based on this is at one's own risk