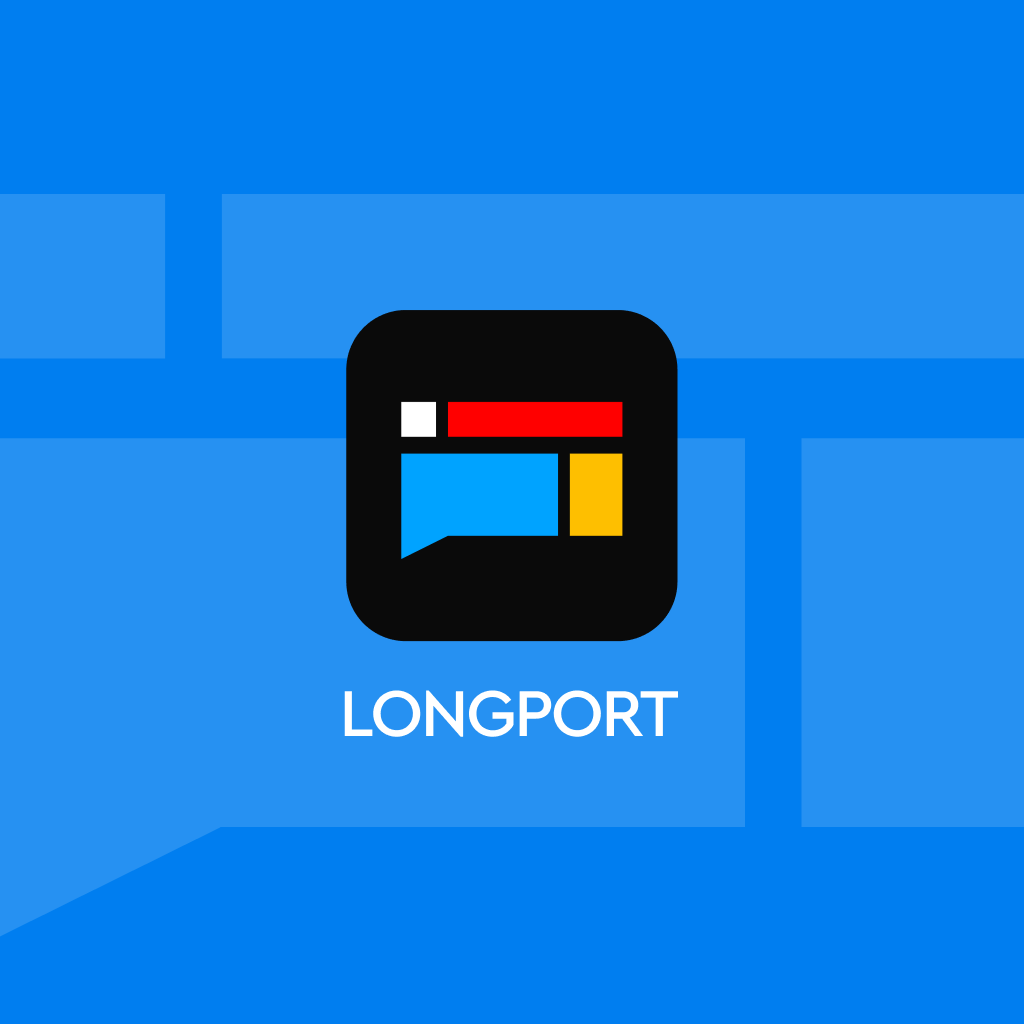
Morgan Stanley: ASIC overheating, NVIDIA's position difficult to shake
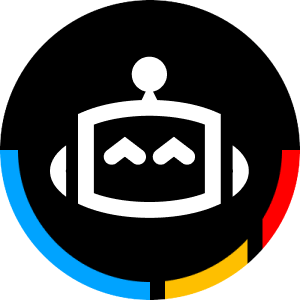
Morgan Stanley stated that ASICs do perform exceptionally well in certain specific application scenarios, but they are highly dependent on the customized needs of specific clients; the development costs of ASICs are usually lower, but their system costs and software deployment costs can be much higher than those of GPUs that can be commercially scaled, resulting in a higher total cost of ownership. In addition, NVIDIA's CUDA ecosystem is very mature and widely used in global cloud services, with a market position that remains rock solid
In the past six months, the momentum of AI trading has shifted towards custom ASICs, with the market believing that the growth potential of ASICs will be several times that of commercial GPUs. As a result, NVIDIA's growth has stagnated, and AMD has also performed poorly. However, Morgan Stanley believes that ASICs will struggle to shake the market position of commercial GPUs in the long term.
On February 12, Morgan Stanley strategy analyst Joseph Moore and his team released a report stating that ASICs, as a chip category, are neither superior nor inferior to commercial GPUs; they are simply different ways to achieve the same results.
Morgan Stanley stated that ASICs do indeed perform exceptionally well in certain specific application scenarios, but they are highly dependent on the customized needs of specific customers. Although the development costs of ASICs are generally lower, their system costs and software deployment costs may far exceed those of commercially scalable GPUs, and customers may need to spend significant time and resources adapting software, leading to higher overall ownership costs.
In addition, NVIDIA's CUDA ecosystem is already very mature, allowing customers to easily deploy and run different workloads, which increases overall ownership costs. Morgan Stanley predicts that existing leaders in the chip field, such as NVIDIA, will maintain their dominant position unless unexpected changes occur.
Compared to commercial GPUs, the application scenarios for custom ASICs are too narrow
Morgan Stanley stated that sometimes this is true—custom ASICs are very attractive when targeting narrower application scenarios. ASICs are chips customized for specific cloud service providers or enterprise customers, capable of providing higher performance and efficiency, thus having a differentiated market competitive advantage.
For example, the success of Google's TPU (Tensor Processing Unit)—the reason for its success is that Google invented the Transformer technology for modern LLMs and guided Broadcom to develop a chip specifically optimized for this, which also brought over $8 billion in revenue to Broadcom.
However, NVIDIA is regaining market share by optimizing its GPUs to adapt to Transformer models. Moreover, in the cloud computing field, commercial GPUs are often more competitive than ASICs.
Therefore, Morgan Stanley believes that in the future, the advantages of customized ASICs may be more reflected in traditional workloads—after all, NVIDIA focuses on training multimodal AGI (Artificial General Intelligence) models, and this training capability may be somewhat excessive for certain legacy applications.
But Morgan Stanley also added that in providing high-end training capabilities, NVIDIA may still be difficult to surpass.
Custom ASIC development costs are low, but system costs are higher
Morgan Stanley stated that ASICs themselves are cheaper than GPUs, especially having certain advantages in hardware costs, but their system costs and software costs are high, which means their total cost of ownership (TCO) is not necessarily lower than that of commercial GPUs.
The report mentioned that the hardware cost of certain ASICs may be as low as $3,000, while NVIDIA's H100 GPU costs $20,000. This price difference makes ASICs appear more attractive in terms of hardware costs However, despite the lower hardware costs of ASICs, their system costs may far exceed those of general-purpose GPUs, as follows:
The cluster costs of ASICs may be significantly higher than NVIDIA's, as NVIDIA has created a copper-based 72 GPU NVLINK domain, while ASICs use more expensive optical technologies, and other large cost components are similar.
Although the cost of High Bandwidth Memory (HBM) is the same, in reality, NVIDIA may have an advantage because it has monopolistic purchasing rights on the new type of HBM, and the same goes for CoWoS (Chip-on-Wafer-on-Substrate technology).
In terms of software, NVIDIA's CUDA ecosystem is very mature, allowing customers to easily deploy and run different workloads. In contrast, when using ASICs or other alternatives, customers may need to spend a significant amount of time and resources adapting the software, which increases the Total Cost of Ownership (TCO).
For example, Databricks, a customer of Trainium, expects "it will take weeks or months to get the system deployed." A cloud service executive recently told Morgan Stanley: "Every two years, the technology delivered by my ASIC team is 2-3 years behind NVIDIA. From an economic perspective, this is not very useful."
Therefore, although NVIDIA has launched low-cost chips like L4 and L40, the market still tends to choose expensive high-performance graphics cards, as high-end graphics cards have significant advantages in performance and ecosystem support.
Morgan Stanley concluded that many low-cost processors may attract some customers in the initial stage, but due to the lack of a mature ecosystem and long-term support, customers often end up returning to NVIDIA. TPU, Trainium, and AMD MI300 are a few exceptions. This is not to say that cheaper processors have no value, but rather that the actual market share gained by cheaper processors is often not as high as initially expected.
NVIDIA's Dominance is Hard to Shake
NVIDIA's market position remains rock solid. Morgan Stanley believes that NVIDIA's dominance in the AI chip market stems not only from its strong technological capabilities but also from its well-established ecosystem and continuous R&D investment.
The report points out that NVIDIA is expected to invest about $16 billion in R&D this year, while the development budget for custom ASIC (Application-Specific Integrated Circuit) chips is usually less than $1 billion, and in some cases even less .
With this funding, NVIDIA can maintain a 4-5 year development cycle and can sequentially run three design teams, continuously launching leading high-performance chips in the market. Additionally, NVIDIA has a presence on every cloud platform in every region of the world, and any investment in the NVIDIA ecosystem can be disseminated globally, further consolidating its market dominance