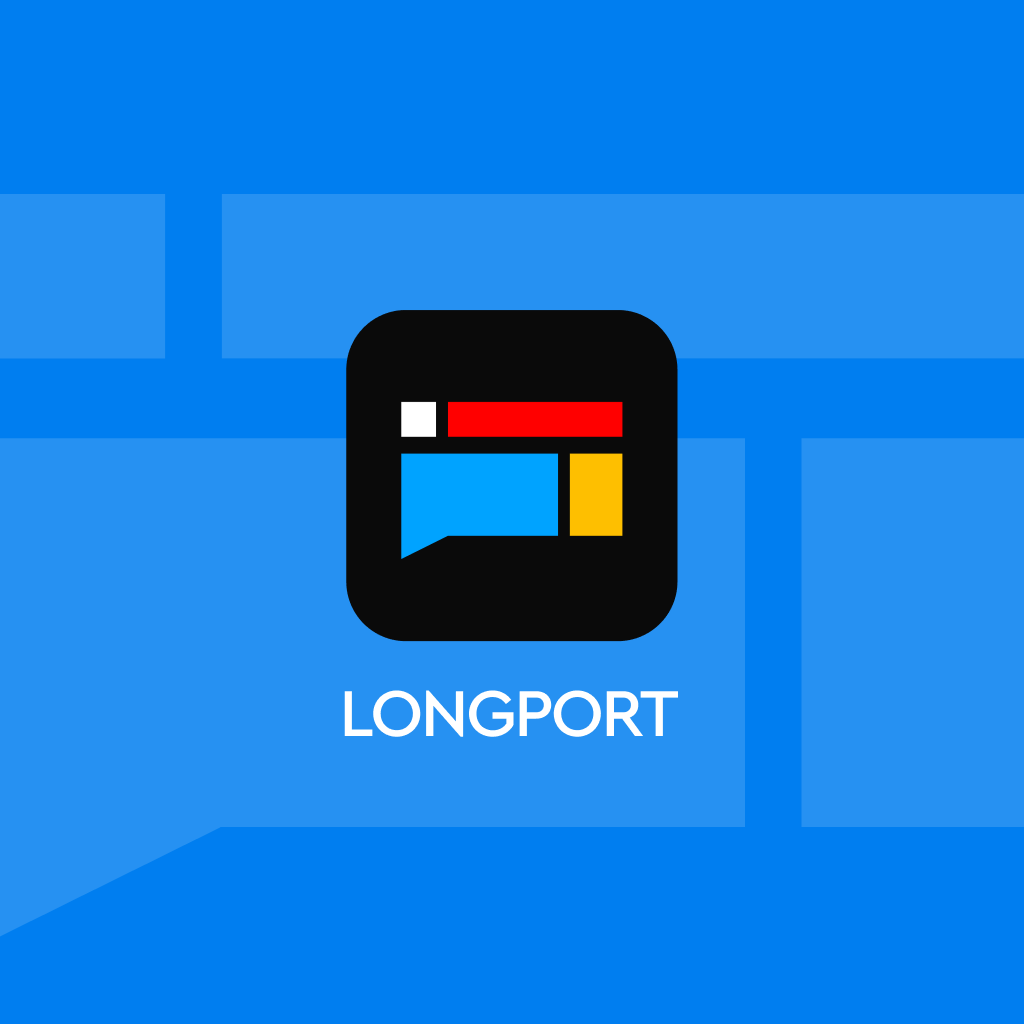
Cambricon is ill, AI has the remedy
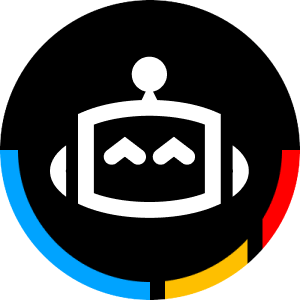
Cambricon is facing challenges, while the AI industry is rapidly developing. The capital market chases short-term narratives, and the future of the AI medical industry is still being explored. Although the AI medical market is expected to reach USD 155.3 billion by 2030, most companies have not yet found effective business models. Companies like Tempus have demonstrated successful business models, leading to significant stock price increases and positive revenue expectations. A report from Ark Invest points out that AI will change drug development and economic returns
777.77, historically known as the Cambrian Peak.
The short to medium-term pursuit in the capital market is a narrative—one that can create pleasure and a closed loop, believing first in harvesting and then in faith.
However, in the real world, AI is indeed in the early stages of a major explosion, and its fission speed may exceed the wildest imagination. Today, let’s think outside the box and look ahead to this time this year and this time next year; the people hyping AI may not be the same group, who knows if it will be financial freedom or extinction? The booming AI startups may also not be the same batch, and even DeepSeek could become yesterday's news.
Finding certainty in change. The gold standard for measuring a high prosperity industry or company is still whether revenue can soar. There are many poor-performing companies in the stationery sector, and almost every time a domestic company rises with the wind, it is a uniform group of underperformers with long-term stagnant revenue. The "leader" in the AI medical industry, YIDU TECH, even has to happily announce a contract worth over 2 million from its affiliated companies; should we set up a monument if Biotech receives a hundred million dollar BD upfront payment?
Watching quietly for changes, if this batch of AI companies shows explosive performance by 2025, then they may have really made it.
The healthcare sector is theoretically the largest application field for AI, with the global market size expected to reach 155.3 billion USD by 2030, but the current monetization of AI in healthcare is still in the early exploration stage, and most companies have not found a business model.
The standard answer is faintly visible. The stock price of the American AI diagnostic company Tempus has doubled in 20 days, rising nearly 150% this year, with expected revenues of about 693 million USD in 2024 and about 1.23 billion USD in 2025, having both scale and speed, with a preliminary successful business model and possessing medical insurance payment codes.
Today, we will look at the reliability and unreliability of AI in healthcare from the perspective of overseas mapping.
Ark Invest released the "Big Ideas 2025" report, predicting that AI will completely change multi-omics tools, drug development, and molecular diagnostics, significantly improving the economic returns of drugs.
01 Insights from the Tempus Model
Tempus founder Eric Lefkofsky is, in a sense, the ancestor of Meituan.
Eric Lefkofsky co-founded Groupon in 2008, which is hailed as the pioneer of group buying websites. On the other side of the Pacific, Wang Xing, who had been struggling with no hope in sight for the social media platform Fanfou, quickly followed Groupon and created Meituan.
Tempus is now illuminating the path for medical AI.
What brought Tempus into the spotlight was Congress's stock god Pelosi, who netted about 38.6 million USD from stocks in 2024. On January 14, 2025, Pelosi purchased 50 call options for Tempus AI expiring in January 2026, immediately sending the stock soaring Sister Pei has a criminal record; she bought call options for Microsoft in March 2021, and less than two weeks later, the U.S. Army announced a $22 billion contract with the software company for AR headset devices.
"Power is like real estate; location is everything. The closer you are to the center, the more valuable your property." The former president in "House of Cards" was absolutely right.
According to Shenwan Hongyuan Research, Tempus has two distinctions compared to traditional testing companies. In terms of data inflow, it collects data from various diagnostic modalities rather than focusing on maximizing profits from single tests, resulting in larger data volumes, richer data dimensions, high quality, and standardization, including genomics, proteomics, clinical data, imaging, etc. Multimodal data is crucial; single data types can no longer meet the development of AI in healthcare. In terms of data exchange, it has established a data pipeline that connects providers, with Tempus collaborating with over 65% of academic medical centers in the U.S. and more than 50% of oncologists.
Based on the above, Tempus possesses the world's largest clinical and molecular database, combining data-driven approaches with AI, and currently has three product lines.
Genomics: Its own laboratory provides NGS (next-generation sequencing) diagnostics, molecular genotyping, and other anatomical and molecular pathology tests, generating various types of molecular data, including hereditary cancer risk data, solid tumor analysis, liquid biopsy, and minimal residual disease (MRD) testing. It acquired the genetic testing company Ambry Genetics to expand its hereditary cancer testing business. It partnered with Personalis to co-commercialize Next Personal, an ultra-sensitive MRD detection and monitoring test based on whole genome sequencing with tumor prior information, which can be used for the detection of ctDNA in the blood of early-stage non-small cell lung cancer and breast cancer patients after curative treatment, as well as for immunotherapy monitoring in all solid tumor late-stage cancers.
Data and Services: The two main products, Insights and Trials, are used to optimize drug development and clinical trials. It has established collaborations with 19 of the top 20 publicly traded pharmaceutical companies, with a total contract value of approximately $940 million, most of which is expected to be delivered in the coming years. The acquired Ambry Genetics generates a large amount of data annually, involving about 400,000 patients, which Tempus can leverage to further enhance its existing data analysis capabilities.
AI Applications: The product NEXT is an AI platform primarily applied in oncology and cardiology, capable of identifying disease progression in patients earlier, especially in the early stages when treatment effects are most significant. It is currently running related applications or algorithms in hundreds of medical institutions, covering tens of thousands of patients. In its electrocardiogram (ECG) product line, the atrial fibrillation prediction algorithm has received FDA approval and has a reimbursement code, allowing hospitals to receive approximately $128 in reimbursement, marking a preliminary breakthrough in the payment model for AI in healthcare According to Soochow Securities, Tempus has a clear strategy, accumulating data through sequencing and testing services to lay the groundwork for future integration with artificial intelligence. From this model, all gene sequencing and testing companies can be seen as a large medical data center, and this business model provides a transformation idea for traditional sequencing and testing companies.
Based on the three major trends in AI healthcare, an overview of AI healthcare companies
02 Judgment of the Three Sisters
AI healthcare in mainland China is still drifting and colliding, and the classification and naming of this world are in chaos. Today, temporarily following the statement of NVIDIA executive Eva-Maria Hempe, AI healthcare is divided into three areas: intelligent agents that improve patient medical processes, robots that perform complex surgical tasks, and generative AI tools that accelerate drug development.
AI detection and diagnosis are the most mature, with domestic comparable companies being KingMed Diagnostics and Dian Diagnostics, which are also expected to follow Tempus's explosion after the holiday.
Cathie Wood's "Big Ideas 2025" report predicts that AI will reduce the costs of reading and writing biological information such as DNA by 100 times and 1,000 times, respectively, and will increase the efficiency of cancer screening by 20 times, expanding the market size by 10 times. Multi-cancer screening technology can identify early signs of multiple cancers through a single blood test, significantly improving screening efficiency. Shield blood testing has become the first major screening option for colorectal cancer approved by the FDA. It is expected that with the improvement of regulations and reimbursement pathways, multi-cancer screening will double the size of the U.S. cancer screening market, growing from the current approximately $80 billion to nearly $100 billion. In addition, minimal residual disease (MRD) testing technology can detect cancer recurrence 20 months earlier than traditional imaging examinations, and it is expected to become a standard care plan for cancer patients in the future, generating data volumes 700 times that of the UK Biobank.
Excluding the impact of the pandemic, KingMed Diagnostics and Dian Diagnostics are both high-quality ICL companies, with substantial accounts receivable bearing significant social responsibility. Who says domestic medical companies are solely profit-driven?
KingMed Diagnostics utilizes large models to deeply explore the potential of medical testing big data, with five data products set to launch on the Guangzhou Data Exchange in 2024, among which the breast cancer data report has completed on-site trading first. This is also the first on-site trading of a medical testing data product in the third-party medical testing industry, officially releasing the first large model in the third-party medical testing industry—Yujian Yiyan large model, which will be open to the public in 2024. Dian Diagnostics has a large amount of self-testing data accumulated across its ten product lines (168 million annual tests + 5.4PB of existing data + 100+TB of monthly data increment), and the uniqueness of its large model lies in using a large amount of closed-source data based on the company's own business. The launched X-MedExplorer clinical research big data platform has collaborated with more than 20 leading tertiary hospitals in China, including Huazhong University of Science and Technology Tongji Medical College Affiliated Union Hospital, to build the largest chronic leukemia drug withdrawal database and prediction model in the country and the second largest in the world Patients can predict whether they can stop or reduce medication based on test results through an AI model after a period of medication.
AI imaging diagnostic technology has matured. As of June 2024, China has approved 92 types of artificial intelligence medical imaging auxiliary diagnostic software as Class III medical devices by the NMPA. Mindray Medical, centered on the "SanRui" digital ecosystem, has partnered with Tencent to launch the world's first large model for critical care medicine—Qiyuan Critical Care Large Model, which can integrate and predict patient conditions and provide suggestions within 5 seconds, generate medical documentation within 1 minute, and offer critical knowledge query results with an accuracy rate of up to 95%. United Imaging Healthcare has launched the uAI intelligent series, including the Imaging Intelligence Large Model and Intelligent Reporting System, increasing investment in United Imaging Intelligence (which has over 100 medical AI products) to enhance its layout in medical AI. The US-listed AI ultrasound company Butterfly Network saw its stock price rise by 183% last year and nearly 50% this year. Its core product, Butterfly iQ+, is a handheld single-probe whole-body ultrasound system that uses semiconductor technology, combined with cloud-connected software and hardware technology, allowing healthcare professionals to obtain imaging information at an affordable price and easily access it via mobile devices.
AI health management is also changing the industry ecosystem. Runda Medical has partnered with Huawei Cloud to extend digital healthcare and AI technology to pharmacies and health check applications. The AI intelligent health assistant Xiao Hui is expected to become an agent for both B-end and C-end applications.
AI drug development is considered the primary AI medical application market, but it is actually the hardest nut to crack, and it is currently difficult to achieve breakthrough progress.
NVIDIA's Eva believes that models for generating, predicting, and optimizing molecules can explore nearly infinite possibilities for drug combinations. Cathie Wood believes that AI will reduce drug development costs by four times and increase the return on investment in R&D by five times, with the commercial value of AI drugs being 20 times higher than standard drugs and 2.4 times higher than the best-in-class precision drugs.
These statements from the two ladies sound impressive, but do they underestimate the complexity of carbon-based life forms?
In the wave of AI, the stock performance of two leading AI pharmaceutical companies, Recursion and Schrodinger, has lagged, indicating that mainstream funds are still observing.
Once again, I admire Pei Jie as a true stock god.
AI can currently only play a supportive role in drug development, and even AI's own responses are filled with pessimism:
About 90% of candidate drugs fail in clinical trials (especially after Phase II), regardless of whether AI is used; issues such as disease mechanisms, human metabolism, and off-target effects cannot be fully simulated by algorithms; success in in vitro experiments or animal models does not necessarily predict human effects; existing drug databases (such as ChEMBL) tend to favor researched molecular structures, leading AI to generate candidate molecules "similar to known drugs" rather than truly innovative ones; individual patient differences and dynamic physiological environments are difficult to structure for AI training; AI models (such as generative models and molecular dynamics simulations) rely on simplified physical or chemical rules, which may overlook key biological details (such as protein conformational changes) The differences between preclinical models (such as cells and mice) and human responses make it difficult for AI to accurately predict side effects; AI can quickly generate candidate molecules, but the processes of synthesis, in vitro testing, and animal experiments still take months or even years, potentially uncovering design flaws.
With algorithm iterations and data accumulation, AI may increase the clinical success rate from 10% to 20%-30%, but it still needs to be deeply integrated with traditional methods.
AI is a tool, not a "disruptor"; the core of drug development remains biological insights and clinical validation.
Give civilization to time, believe that time will remove technological barriers, and believe that everything will be new next year; not only will the answers change, but even the questions will change.
Source: Archimedes Biotech, original title: "The Cambrian is Sick, AI has the Medicine"
Risk Warning and Disclaimer
The market has risks, and investment requires caution. This article does not constitute personal investment advice and does not take into account the specific investment goals, financial conditions, or needs of individual users. Users should consider whether any opinions, views, or conclusions in this article align with their specific circumstances. Investing based on this is at your own risk