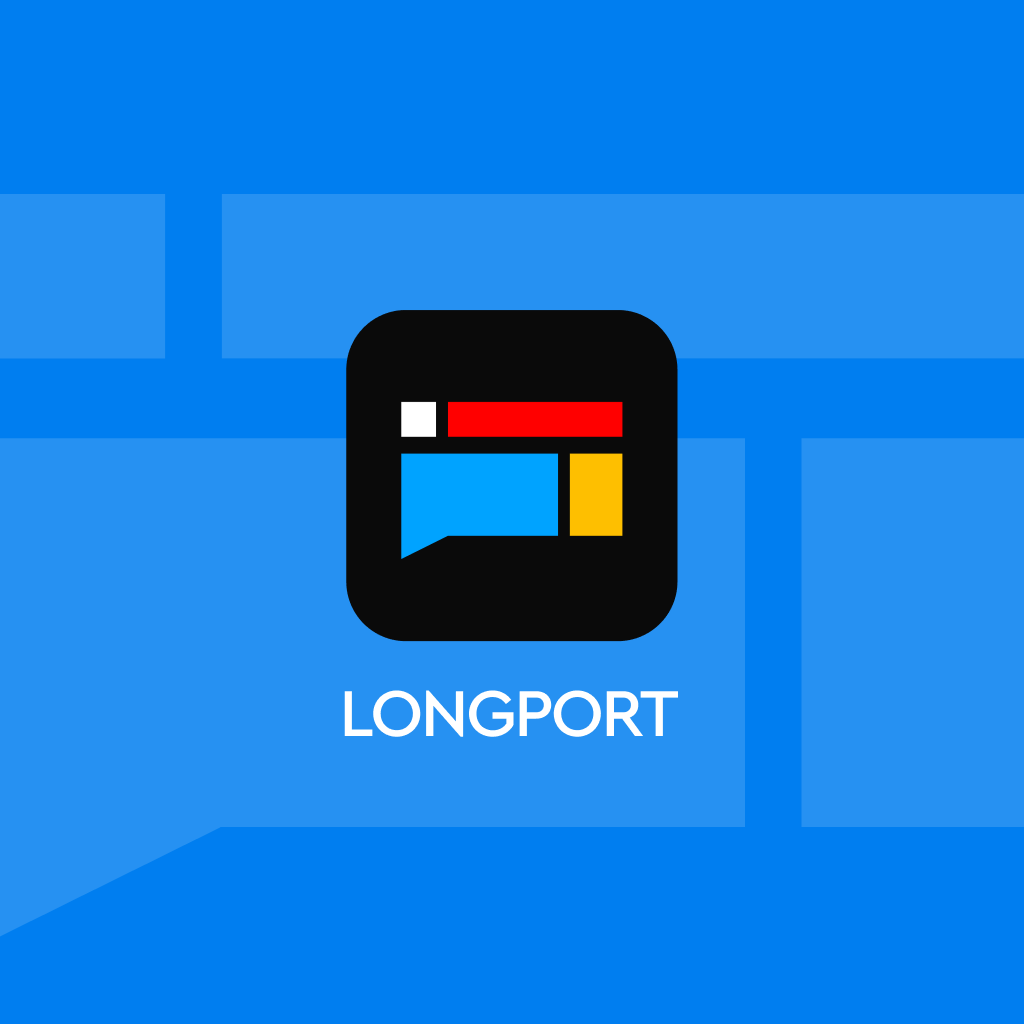
Silicon Valley AI Inspection Report
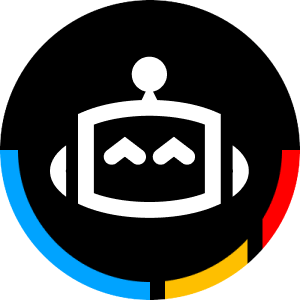
This Silicon Valley AI inspection report summarizes the learning and exchanges with companies such as Microsoft, Google, NVIDIA, and Meta, emphasizing that AI technology has deeply integrated into various aspects of society and that technological progress is rapid. The report points out that the AI revolution is regarded as a significant transformation in human history and may represent the pinnacle of technological development. The inspection focused on discussing the underlying technologies of large models, computing power requirements, and industry applications, particularly the technical architecture of the GPT large model and its potential in practical applications
In order to gain a close understanding of the latest developments and firsthand information on the cutting-edge AI in Silicon Valley, we recently made a special trip to Silicon Valley for study and inspection. We visited the headquarters of companies such as Microsoft, Google, NVIDIA, and Meta, and had in-depth exchanges with many industry insiders, gaining a lot of insights and deep feelings. Through the exchanges, we learned that many of the cutting-edge understandings about computing power and large models differ significantly from the current understanding in the domestic capital market, even to the point of being worlds apart. These differences may contain important investment opportunities. Therefore, we would like to share the insights gained from this study and inspection with everyone at the first opportunity.
Our biggest impression from this trip is that in the United States, AI is no longer a new thing that sparks curiosity and controversy; it has integrated into various aspects of social production, corporate operations, and people's lives, just like water and electricity. At the same time, the speed of technological advancement in AI is in a "Cambrian" era, where exciting new technologies or products emerge almost every week or even every day and are quickly applied to practical scenarios. Therefore, American VC and PE can be said to be almost ubiquitous, delving into all aspects of AI to seek investment opportunities.
Global tech giants, represented by Google, generally believe that this AI revolution is an unprecedented upheaval for humanity, marking an extraordinary turning point in human progress. We have previously pointed out that this time, AI will combine the highest traffic entrance of the internet with the largest market of public cloud and a unified operating system for all things. Even so, we may still underestimate its significance: this could be the greatest technological revolution in human history!
We will introduce the insights gained from the Silicon Valley AI study and inspection trip from three aspects: the underlying technology and computing power requirements of large models, industry applications, and the development trends of large models and vertical models.
Underlying Technology and Computing Power Requirements of Large Models
First, the underlying technology of the GPT large model is Google's TransFormer, whose core significance lies in inferring the next most likely character for output based on the preceding context. The difference in the foundational large models of some giants lies in whether they use a unidirectional decoding structure or a bidirectional encoding-decoding structure. ChatGPT can support the analysis of the preceding 32,000 characters to infer the next character, which is already a very large magnitude. Moreover, ChatGPT only allows inference based on the preceding context and does not permit the inclusion of subsequent context for analysis (decoder only). Google's first large model, Bard, could analyze and infer using both preceding and subsequent contexts (bidirectional encoding-decoding structure), but it later changed to the PaLM large model, which is similar to ChatGPT and can only infer based on the preceding context. This model may ultimately be closer to the way we use human language.
Second, large models essentially resemble "alchemy" that relies on AI infrastructure; they are about inference rather than cognition. Currently, video generation is still somewhat limited due to the high demands on infrastructure. While ChatGPT performs astonishingly in QA, summarization, and other fields, it is still about inference rather than cognition. The generation of images is essentially also about generating text, but AI inference in video generation is very challenging Because each frame of the video consists of a large number of images, it requires a very powerful AI infrastructure to support it. Figuratively speaking, training a large model is like alchemy; the better the AI infrastructure, the stronger the firepower. However, at the beginning, it is uncertain whether the large model is useful, and there is a certain element of luck involved.
Third, the difficulty for latecomers to catch up with large models is not as great as everyone imagines, and China's large models will catch up with overseas ones relatively quickly. OpenAI itself is not absolutely technologically ahead of other tech giants; the core lies in focusing on the development of Transformers towards general artificial intelligence, while Microsoft, Google, and Meta have many profitable businesses and do not pay much attention to large models. After OpenAI's success, large companies realized the potential of AI and will definitely accelerate their pursuit with better resources. Silicon Valley experts predict that in 6 months to 1 year, the large model levels of major global companies will basically be on par. China's large models will also catch up with overseas ones relatively quickly, as China itself is a very good market. Now, everyone understands all the technologies related to large models; it is merely a matter of resource concentration. OpenAI's success and its "submission" to Microsoft is precisely because training is too costly.
Fourth, overseas AI giants have a computing power reserve of A100 chips at a scale of over 500,000 units. NVIDIA is developing its computing power resources towards cloud services while also laying out its own large models. Currently, the average number of A100 chips among overseas giants is estimated to be over 500,000, while H100 chips may be around one or two hundred per company, with large-scale deployment expected around June or July. NVIDIA's actual advantage lies in the combination of hardware and software; its hardware has a framework layer called TensorRT, and NVIDIA has a team of hundreds of engineers designing frameworks. For example, PyTorch must have TensorRT to run, and this intermediate layer of software is generally not something hardware companies can write. NVIDIA not only makes hardware but also develops TensorRT and the underlying infrastructure. In the future, NVIDIA is expected to form a cloud brand while also laying out large models, which may have a significant impact on the entire AI ecosystem.
Fifth, the market for inference chips is much larger than that for training chips, and it is even larger than the total of the training market plus the cloud inference market. China has a very large market space in edge AI computing power. Edge computing applied to small devices in the Internet of Things has low process requirements, and the current market structure is fragmented. The market for inference chips is much larger than that for training chips, and it is even larger than the total of the training market plus the cloud inference market. China can leverage its manufacturing advantages to reduce the process for the Internet of Things sector and push specialized, small-scale, low-computing power AI inference chips to the market, which is a huge opportunity. In fact, the scale of terminal devices is enormous. The number of data centers provided by cloud service providers in the world is small compared to the vast number of terminal devices, with chip demand being roughly in a 2/8 ratio.
Regarding the underlying technology and computing power requirements of large models, we believe:
1. There is no ceiling on computing power demand. Currently, the main computing power demand for large models comes from text training. In the future, as we move from text to images and then to videos, from training to inference, and from cloud to edge, the continuous high growth of computing power demand is highly certain.
2. The market landscape for GPU chips may change. With strong support from giants like Microsoft, AMD's relatively weak software ecosystem is expected to make significant progress, posing a strong challenge to NVIDIA.
3. Chips represent the largest gap in the competition between China and the United States. Achieving a comparable level of computing power reserves between the two countries is both a bottleneck that needs urgent resolution and a certain investment opportunity for the future. Especially in edge-side inference computing power, which is an underestimated market that far exceeds training computing power, it also provides China with an opportunity to showcase its manufacturing advantages.
About AI Industry Applications
First, large models are suitable for industries that require a certain tolerance for errors. ChatGPT's commercial paid usage, known as Plus, is actually not profitable; the core purpose is to filter out some users who misuse the service, thereby increasing costs. Currently, applying large models in industries that require 100% accuracy is quite challenging. More commonly, they are used in customer service consultations, artistic creation, meeting minutes, article writing, data analysis, etc. The commercialization of large models has already shown results in the B-end, such as: Microsoft's Office suite, which reduces production time, enhances completion rates, and increases repurchase rates; customer service: saving front-end customer service costs for real estate and medical companies. Video production: tools like visla.us that can generate demo videos with one click, eliminating the need to find studios and saving labor costs. GPT-4 has only been around for a month and a half, and the market is still discussing how to apply it. In six months, we can expect to see more clear implementations.
Second, Microsoft's M365 products focus on large-scale delivery, privacy, and security. Microsoft's main goal now is how to deliver at scale, especially in solving some personalized AI features, along with preparations for security and privacy. M365 is currently Microsoft's core product. For the entire workflow of enterprises, the entire collaboration platform, all tools, storage, and security are under the M365 umbrella. Copilot significantly enhances the production capabilities of existing product lines. M365 has two different computing systems, relying on Azure's data centers for global expansion, and M365 also has its own data centers internally; M365 embeds OpenAI into its products rather than using public OpenAI. There are challenges in implementing M365 technology in China: 1) computing resources; 2) Regulations: Data transparency and management of sensitive information.
We believe that in the United States, the application of AI technology has become very common in various industries, such as customer service consultation, artistic creation, meeting minutes, article writing, data analysis, etc. However, it should be noted that the current application of large models should be positioned as "co-pilots," requiring a certain degree of fault tolerance rather than deterministic decision-making. In addition, the application of overseas large models represented by Microsoft still faces significant difficulties in entering China. These difficulties are not only related to data security and compliance policy requirements but also face many challenges in the localized deployment of large models and computing resources.
Development Trends of Large Models and Vertical Models
First, it is highly likely that the large models of Google and Microsoft will be closed-source, while Meta may be the most important open-source "disruptor." Google has no way out as its search will be disrupted by large models; if it opens its large models, it will lose its advantages, and AI will become an important profit-making tool in the future, so it is highly likely to remain closed-source. Microsoft completely relies on OpenAI, hoping that GPT will empower efficiency tools like MS365 Copilot and the Bing search engine, and it is also unlikely that Microsoft will open-source its AI. Meta's most important business is social networking, and AI can serve as a chat assistant. Meta's approach is to create large models and then open-source them, becoming a "disruptor" within the large model space. Comparatively, Meta's large model has 175 billion parameters, while GPT-4 is estimated to have around 500 billion parameters. Meta has open-sourced a large model with over 65 billion parameters, which is estimated to be about 20% less accurate than ChatGPT. Many companies and learners use Meta's open-source model for fine-tuning, achieving results comparable to GPT with a much smaller model size. The significance of open-source lies in mobilizing millions of engineers worldwide to participate in fine-tuning.
Second, the trend is for large models to move to mobile devices. In the future open-source ecosystem of large models, large companies will create large models while small companies will focus on fine-tuning. Large models will also be simplified for various mobile terminals, such as changing from 32-bit floating-point operations to INT8, enhancing computational speed. Large language models will have a good ecosystem in open-source, similar to water and electricity, allowing the open-source ecosystem to thrive in certain niche areas. Some clever individuals in the open-source community can distill models to a very small size, such as changing from 36-bit floating-point operations to INT4, which can reduce the size by ten times, making it small enough to be installed on computers and mobile phones. In the future, many creative applications may be developed. iOS or Android may eventually embed large models, and all mobile applications may incur a fee to Apple for running once.
Third, the continued development of large models must consider the ROI of increasing parameter counts. From a research perspective, more parameters are certainly better, but from a commercial usage perspective, each additional parameter increases costs, including collection and training costs. ChatGPT 3.0 used 175 billion parameters, while a GitHub model that mimics GPT only used 70 billion parameters to achieve 90% of GPT's effectiveness From a business application perspective, it is necessary to find the parameters with the highest ROI.
Fourth, large models will ultimately dominate some vertical industries where data can be obtained via the internet, but may not cover certain vertical domain models where data cannot be obtained. Currently, Google is doing something to enable AI to learn internet content in real-time like humans, and in areas where data cannot be obtained offline, there may be forms of interaction between online large models and local models, but this involves a complex coupling issue.
We believe:
1. China may be the biggest beneficiary of open-source large models represented by Meta.
2. We should remain confident in the progress of domestic large models catching up to global leading levels. By pursuing based on the already defined technological direction and open-source large models, it actually saves the trial-and-error costs of starting from scratch. Especially for leading companies in vertical industries that do not have high requirements for the generality of large models, leveraging open-source large models can quickly build vertical large models and accelerate the application landing in vertical fields.
3. Deploying large models on the edge and mobile devices is an inevitable trend, especially after Google recently released large models for mobile and ChatGPT officially launched its app on Apple phones, this trend is gradually being recognized by the market. Large models are the long-awaited unified operating system for various non-standardized AIoT terminals.
Wall Street Journal invited Minsheng Securities Chief Computer Analyst Lv Wei to launch the “Finding the Next ‘NVIDIA’” master class, a course that clarifies investment opportunities across the entire AI industry chain. You are welcome to click here to join the learning.
— “Finding the Next ‘NVIDIA’” Course Schedule —
Risk Warning: The master class is a platform for selected third-party compliance professionals to teach investment research theory courses. The content taught does not constitute buy or sell recommendations for any specific products. The opinions expressed in the platform courses are for learning and reference only and do not represent the opinions or views of Wall Street Journal, nor do they address the specific investment goals, financial situation, or needs of users. The market is volatile and uncertain, and the platform is not responsible for any losses incurred by you relying on the opinions or information from the course. Investment carries risks; please make cautious decisions