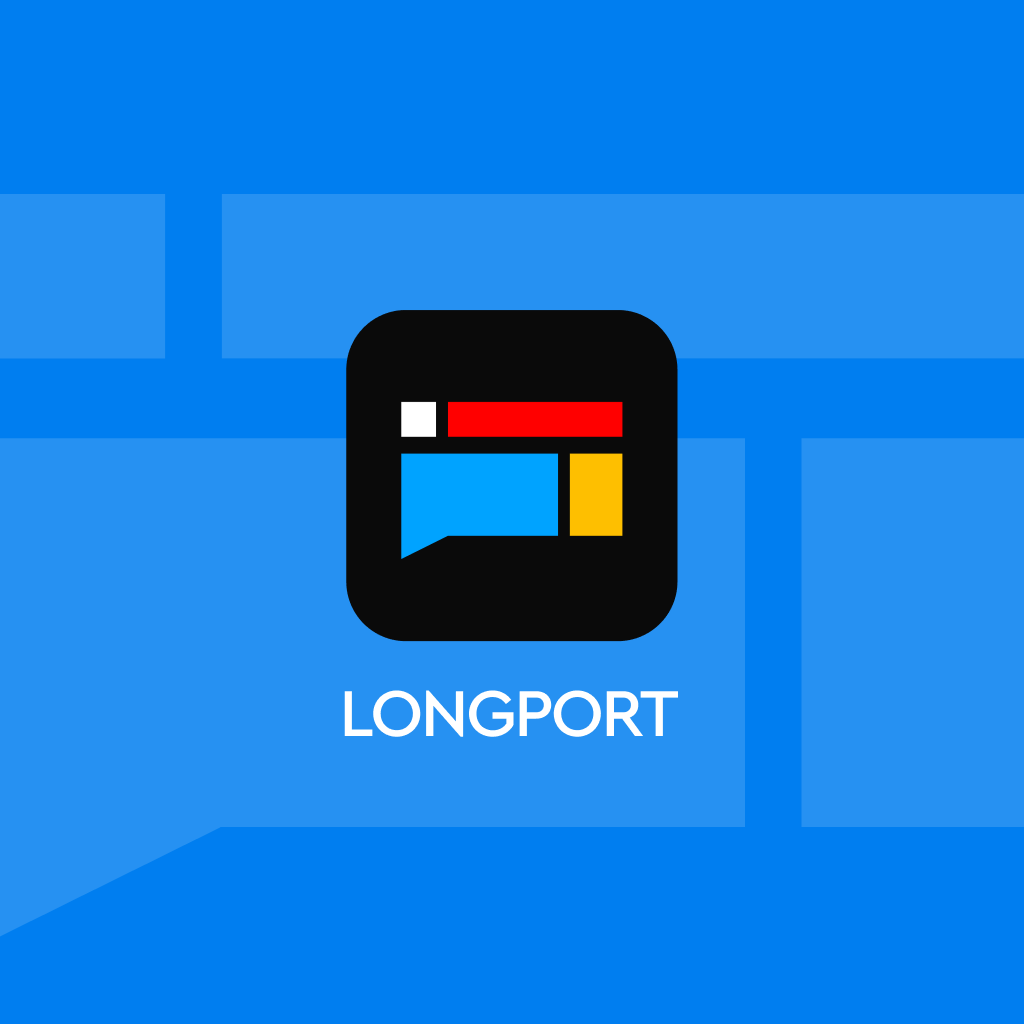
DeepSeek Core Ten Questions and Answers, focusing on core investment opportunities such as computing power, applications, edge computing, and data
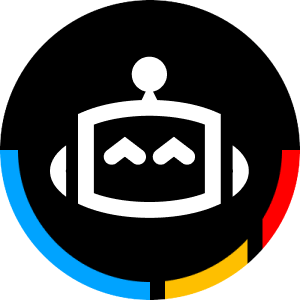
The DeepSeek-R1 model has been released, featuring high performance and low computing power requirements. As an open-source model, R1's performance is close to that of leading closed-source model o1, which to some extent reflects AI equity. It is expected to promote sustained high prosperity and high attention across the entire AI industry chain, focusing on core investment opportunities in computing power, applications, edge computing, and data
DeepSeek Model Intensive Updates, High Performance + Low Cost Promote Rapid User Growth
Recently, multiple models from DeepSeek have been launched and fully open-sourced, among which R1 has basically achieved performance comparable to o1 in inference tasks, and Janus-Pro has performed well in multimodal understanding and generation. Driven by the dissemination of information during the Spring Festival, DeepSeek has gained traction and become the fastest-growing AI-native application globally, reaching 15 million daily active users on the 18th day. In addition, through algorithm iteration and architecture upgrades, DeepSeek has reduced the costs of general and inference models to less than one-tenth of similar models from OpenAI.
Continuous Technological Innovation, Large Model Scaling Law Still Effective
DeepSeek has achieved efficient training through innovations in architecture and infrastructure such as multi-head latent attention, MoE, and multi-token prediction, and has validated the enhancement of inference capabilities through pure reinforcement learning in the R1-Zero model. Although Pre-Training Scaling faces constraints in technology, computing power, and data, reinforcement learning has brought a new direction for scalable expansion, and it is expected that various manufacturers will follow suit and continue to optimize model architectures.
DeepSeek-R1 Promotes AI Equality, Industry Chain Enjoys Development Dividends
As an open-source model with performance close to the leading closed-source model o1, R1 reflects AI equality to some extent. At the same time, R1 makes it possible for smaller models to possess inference capabilities, and lower costs will be more conducive for developers to explore the practical implementation of AI.
1. DeepSeek Model Intensive Updates, High Performance + Low Cost Promote Rapid User Growth
1.1 First Question: What is the trend of DeepSeek's user volume?
DeepSeek is firmly committed to an open-source route, intensively updating MoE, inference, and multimodal models. Recently, DeepSeek has continuously released and open-sourced several large models, and its low-cost, high-performance characteristics have quickly attracted global user attention.
Among them, the DeepSeek-V3 released on December 26, 2024, is a self-developed MoE model with 671 billion parameters, requiring only 37 billion to activate during operation, and has been pre-trained on 14.8 trillion tokens of data; the DeepSeek-R1 released on January 20, 2025, is a high-performance inference model with 660 billion parameters, allowing users to output thought chains and enabling users to train other models using R1 through distillation technology; on January 27, 2025, DeepSeek uploaded the visual model Janus-Pro and the multimodal understanding model JanusFlow -1.3B to the Hugging Face platform, further strengthening its efforts in the image field.
The access volume of DeepSeek's web and app platforms continues to grow, with the dissemination of information during the Spring Festival accelerating the product's attention explosion
On the web, from October 2024 to December 2024, DeepSeek's traffic reached 2.45 million / 4.22 million / 11.01 million, with November and December showing year-on-year growth of 72.24% / 160.90%, respectively. In December, traffic saw a significant increase due to the launch of the new open-source model V3;
On the app side, DeepSeek's official app launched on iOS/Android on January 10, 2025 (official public account published on January 15). Subsequently, benefiting from the high performance and low cost of the R1 model released on January 20, combined with the information dissemination during the Spring Festival, product attention experienced exponential growth. Specifically, the daily download volume of the DeepSeek app in the Chinese region for both Android/iOS saw a sharp increase around January 26, reaching daily downloads of 784.15 thousand / 29.92 thousand by January 29;
At the same time, DeepSeek ranked fourth in the Huawei App Store download rankings for the Android version, while the iOS version topped the charts in 160/162/171 out of 173 regions globally for overall (free) / applications (free) / efficiency (free) categories. Additionally, from the product launch date, the daily active users showed that DeepSeek surpassed ChatGPT on the fifth day, reaching 2.59 million daily active users on the fifteenth day, which is twice that of ChatGPT, making it the fastest-growing AI native application globally. On the eighteenth day, it reached 15 million daily active users, while ChatGPT only reached 15 million DAU on its 244th day after launch.
We believe that DeepSeek's user base will continue to grow rapidly. On one hand, as a steadfast practitioner of the open-source route, DeepSeek is expected to receive significant attention from global developers; on the other hand, benefiting from the information dissemination during the Spring Festival, DeepSeek's domestic penetration rate will continue to rise.
1.2 Second Question: How is the performance of the R1 and Janus-pro models?
DeepSeek-R1 has basically achieved performance comparable to OpenAI-o1 in inference tasks, although there is still a gap compared to the o3 model. During the testing process of the R1 model, DeepSeek selected benchmark tests in English, Chinese, mathematics, and code, comparing it with models such as Claude-3.5, GPT-4o, DeepSeek-V3, OpenAI o1, and OpenAI o1-mini:
Education-oriented knowledge tasks: In knowledge benchmarks represented by MMLU (R1 90.8 points; V3 88.5 points; o1 91.8 points) and GPQA Diamond (R1 71.5 points; V3 59.1 points; o1 75.7 points; o3 87.7 points), R1 demonstrated superior performance compared to V3, primarily due to significant improvements in accuracy on STEM-related questions facilitated by large-scale reinforcement learning (RL); in the FRAMES benchmark that relies on long context (R1 82.5 points; V3 73.7 points), R1 also showcased strong document analysis capabilities Chinese and English search and data analysis tasks: In the English factual benchmark SimpleQA (R1 30.1 points; V3 24.9 points; o1 47.0 points), R1 outperformed V3, demonstrating the model's fact-based query capability; however, in the Chinese factual benchmark C-SimpleQA (R1 63.7 points; V3 68.0 points), R1 performed worse than V3, mainly due to the model's tendency to refuse to answer certain queries after safety reinforcement learning.
Without safety RL, R1's accuracy could exceed 70%. Additionally, the R1 model also performed well in benchmarks such as IF-Eval (R1 83.3 points; V3 86.1 points), AlpacaEval2.0 (R1 87.6 points; V3 70.0 points), and ArenaHard (R1 92.3 points; V3 85.5 points), showcasing the model's ability in following format instructions, writing tasks, and open-domain question answering.
Mathematical tasks: In mathematical tasks, R1 demonstrated performance comparable to o1, outperforming other non-inference models, highlighting the dominance of inference models in mathematical testing. For example, in the AIME 2024 benchmark, R1/V3/o1/o3 scored 79.8/39.2/79.2/96.7 points respectively; in the Math-500 benchmark, R1/V3/o1 scored 97.3/90.2/96.4 points respectively.
Coding tasks: In coding tasks, inference models also performed better, for instance, in the Codeforces benchmark, R1/V3/o1/o3 scored 2029/1134/2061/2727 points respectively, surpassing 96.3%/58.7%/96.6%/99.9% of human participants; in the SWE-bench Verified benchmark, R1/V3/o1/o3 scored 49.2/42.0/48.9/71.7 points respectively.
Distillation technology can significantly enhance the reasoning ability of small models. By distilling the outputs of DeepSeek-R1 into a more efficient small model, the reasoning capability of the small model can be significantly improved.
For example, distilling the R1 model into Qwen2.5-Math-7B resulted in DeepSeek-R1-Distill-Qwen-7B (referred to as R1-7B hereafter), which comprehensively surpassed non-inference models like GPT-4o; distilling into Qwen2.5-14B resulted in R1-14B exceeding QwQ-32B-Preview on all evaluation metrics; while R1-32B and R1-70B distilled from Qwen2.5-32B and Llama-3.3-70B-Instruct significantly outperformed o1-mini in most benchmark tests.
Janus-Pro outperforms unified models and single-function models in multimodal understanding and generation. Janus-Pro mainly continues Janus's research approach by decoupling multimodal understanding and generation, improving model performance through optimized training strategies, expanded training data, and model scale:
Multimodal Understanding: During the Janus testing process, widely recognized image-visual language benchmarks such as POPE, MME-P, MMB, SEED, MMMU, and MM-Vet were selected, along with a new dataset GQA for real-world visual reasoning and compositional question answering.
Compared to other cutting-edge unified models for image understanding and models used solely for understanding, Janus-Pro achieved the overall best results, such as Janus-Pro-7B scoring 79.2 on the multimodal understanding benchmark MMBench, surpassing Janus (69.4), TokenFlow (68.9), and MetaMorph (75.2). The main reason is that it decouples the visual encoding of multimodal understanding and generation, alleviating the conflict between these two tasks. Additionally, Janus-Pro remains competitive compared to larger models; for instance, Janus-Pro-7B outperformed TokenFlow-XL (13B) on other benchmark tests except for GQA.
Text-Image Generation: To evaluate Janus's visual generation capabilities, DeepSeek used two tools for testing: GenEval (text-to-image composition ability benchmark) and DPG-Bench (dense prompt image benchmark).
Janus-Pro-7B achieved an overall accuracy of 80% on GenEval, surpassing all other unified models or models used solely for generation, including Transfusion (63%), SD3-Medium (74%), and DALL-E 3 (67%), reflecting Janus-Pro's better instruction-following ability. Meanwhile, Janus-Pro scored 84.19 on DPG-Bench, exceeding all other methods, indicating that Janus-Pro excels in following dense instructions for text-to-image generation.
We believe that DeepSeek-R1's performance has basically reached the level of OpenAI-o1, with still a significant gap compared to the o3 model benchmark. As DeepSeek further iterates on technologies such as MoE architecture and reinforcement learning, the performance of the inference model is expected to continue to grow; Janus-Pro performs relatively well in multimodal understanding and generation, validating the feasibility of the decoupling approach for image understanding and generation to some extent.
1.3 Third Question: How to view the training cost of the DeepSeek-V3 model? The cost of DeepSeek's general and inference models has dropped to less than one-tenth compared to similar models from OpenAI:
In terms of general models, the DeepSeek-V3 update will go live on December 26, 2024, with the model API service pricing adjusted to 0.5 yuan per million input tokens (cache hit) / 2 yuan (cache miss), and 8 yuan per million output tokens.
Additionally, the V3 model offers a promotional price experience period lasting up to 45 days: until February 8, 2025, the API service price for V3 will remain at 0.1 yuan per million input tokens (cache hit) / 1 yuan (cache miss), and 2 yuan per million output tokens. Meanwhile, OpenAI's GPT-4o API service pricing is set at 1.25 USD per million input tokens (cache hit) / 2.5 USD (cache miss), and 10 USD per million output tokens.
For inference models, the DeepSeek-R1 API service pricing is 1 yuan per million input tokens (cache hit) / 4 yuan (cache miss), and 16 yuan per million output tokens. In contrast, OpenAI's o1 API service pricing is 7.5 USD per million input tokens (cache hit) / 15 USD (cache miss), and 60 USD per million output tokens.
It is important to note that different models may have different token segmentation methods; typically, 1 token corresponds to 1-2 Chinese characters, or 3-4 English characters, or 0.75 English words.
The total training cost of DeepSeek-V3 (the base model of R1) is only 5.576 million USD, excluding costs related to architecture, algorithms, etc.
Using H800 computing power, the pre-training phase of DeepSeek-V3 was completed in less than two months, consuming 2.664 million GPU hours, plus 119,000 GPU hours needed for context length expansion and 5,000 GPU hours for the post-training phase, resulting in a total of only 2.788 million GPU hours for complete training; assuming the rental price of H800 GPUs is 2 USD per GPU hour, our total training cost is only 5.576 million USD. It should be noted that the above cost only includes the formal training cost of DeepSeek-V3 and does not include costs related to preliminary research and ablation experiments concerning architecture, algorithms, or data.
According to our calculations, GPT-4 requires 25,000 A100 GPUs for 95 days (57 million A100 GPU hours), while OpenAI o1 needs 32,000 H100 GPUs for 90 days (69.12 million H100 SXM GPU hours):
GPT-4 consists of 16 MoE models with 111 billion parameters, of which two are used for forward propagation, and another 55 billion are shared for the attention mechanism, resulting in an activation parameter count of approximately 280 billion. We assume the activation parameter count of the o1 model is twice that of GPT-4, reaching 560 billion;
The token count of GPT-4's pre-training dataset is 13 billion, and we assume the o1 model is close to twice that, reaching 25 billion;
The training time for GPT-4 is approximately 90-100 days, we take the average of 95 days and assume the training period for o1 is 90 days;
The GPU utilization rate for GPT-4 is between 32% and 36%, we take the average of 34% and assume the GPU utilization rate for o1 is also 34%;
Based on the empirical formula provided by OpenAI in the Scaling Laws paper (C = rT ≈ 6PD, where P is the model parameter count, D is the training dataset token size, and r is the total throughput of the training cluster hardware FLOPS), OpenAI o1 pre-training requires 32,000 H100 GPUs.
Algorithm iteration and architectural upgrades have reduced the training costs of the DeepSeek-V3 model, aligning with industry trends. Compared to the GPT-4 and o1 models, the base model DeepSeek-R1 has significantly lower training costs. Combining the V3 technical report and the calculations above, we believe the cost optimization is mainly due to:
The V3 model uses the DeepSeekMoE architecture (to be further explained in section 3.1), employing more granular expert models while isolating some shared experts, improving computational resource utilization, with fewer activation parameters (only 37 billion) and lower computational consumption;
The V3 model adopts the MLA algorithm (to be further explained in section 3.1), which compresses attention key-value pairs through low-rank joint compression, reducing the key-value (KV) cache during inference and lowering the computational load;
The Dual Pipe framework achieves efficient pipeline parallelism, significantly improving GPU utilization;
DeepSeek has proposed a fine-grained mixed precision framework utilizing FP8 data format for training, optimizing training efficiency through low-precision training.
2. Continuous technological innovation, the Scaling Law for large models remains effective
2.1 Fourth question: What are the technological innovations of DeepSeek-V3/R1? Through architecture and infrastructure innovation, DeepSeek-V3 has achieved efficient training, laying the foundation for R1 model optimization. In terms of architecture, DeepSeek-V3 continues the MLA and DeepSeek MoE architecture of the V2 model, while further pioneering a load balancing strategy without auxiliary loss and setting a multi-token prediction (MTP) training objective to enhance performance:
Multi-Head Latent Attention (MLA): The core mechanism of LLM is self-attention, which requires the model to consider the relationships of all previous words when generating each token, resulting in an overall complexity of O(n^3) when the text length is n; past research has proposed the KV Cache method, which uses key-value pairs (KV) to store computed attention information, reducing the overall complexity to O(n^2); MLA further reduces the cache requirements for keys and values by storing the dissimilar information of tokens through projection matrices, with almost no loss of information.
DeepSeek MoE: The Mixture of Experts (MoE) model is an alternative to feedforward neural networks (FNN) in current large model technology. Unlike FNN, which requires all weights to participate in computation, MoE uses a gating mechanism to determine which expert models should process the input data. Compared to mainstream MoE models, DeepSeek MoE employs finer-grained experts and isolates some models as shared experts, further optimizing activation parameters. Additionally, to address routing crashes and reduced computational efficiency caused by imbalanced expert loads, DeepSeek proposes a load balancing strategy without auxiliary loss, adding dynamically adjustable bias terms to each expert model to ensure balanced expert loads during training and improve model performance.
Multi-Token Prediction (MTP): Mainstream large models generate sequences token-by-token, and each token generation requires frequent interaction with memory, creating bottlenecks in training or inference due to memory efficiency. The MTP method primarily transforms single-token generation into multi-token generation, enhancing training and inference performance. DeepSeek has optimized past MTP algorithms to sequentially predict additional tokens while maintaining a complete causal chain at each prediction depth.
In addition to the architecture, DeepSeek has also made certain optimizations in infrastructure. For example, it has designed an innovative pipeline parallel algorithm, DualPipe, which overlaps computation and communication within each pair of forward and backward blocks, improving communication efficiency and accelerating model training; it has proposed a mixed precision framework for FP8 training, where most compute-intensive operations are performed at FP8 precision, while some key operations are strategically kept in their original data format to balance training efficiency and numerical stability During the training process, NVIDIA PTX (Parallel Thread Execution) assembly-level programming was used instead of the standard CUDA solution, achieving hardware-level deep optimization, reducing computational redundancy, and improving inference speed.
R1-Zero validates the enhancement of inference capabilities through pure reinforcement learning (RL), while R1 emphasizes the balance between cold start and multi-stage training. The uniqueness of R1-Zero lies in its ability to achieve strong inference capabilities without any supervised fine-tuning data, reflecting the model's ability to effectively learn and generalize solely through reinforcement learning.
Specifically, the R1-Zero model continues the relative policy optimization algorithm (GRPO) from the DeepSeek-V3 group during the RL process, optimizing policies through intra-group reward comparisons without the need for an additional discriminator, ultimately achieving a continuous increase in average response length on the training set, naturally learning to solve inference tasks by allowing more thinking time; additionally, the R1-Zero training process naturally gives rise to "thinking ability," where the model spontaneously learns to reassess its initial answers and allocate more thinking time to questions. This characteristic of "reflection" can somewhat address the hallucination problem of large models (where large models output token by token, lacking mechanisms to correct previously output errors, which instead continue to obscure earlier issues, leading to hallucination problems).
Despite the R1-Zero model demonstrating strong inference capabilities, it still faces challenges such as poor readability and language mixing, which the R1 model addresses through cold start and multi-stage training.
R1 also starts from the DeepSeek-V3-Base foundational model, undergoing fine-tuning (SFT) with thousands of high-quality long-chain thinking (CoT) data as a cold start, making the model's outputs more compliant and readable; subsequently, the fine-tuned model undergoes large-scale reinforcement learning similar to R1-Zero, introducing language consistency rewards until the model converges on inference tasks; after the reinforcement learning for inference converges, new SFT data is collected using the generated checkpoints, integrating data from other domains to enhance the model's capabilities in writing, role-playing, and other general tasks; finally, to further align the model with human preferences, a secondary RL phase is implemented, aimed at improving the model's usefulness and harmlessness, refining its inference capabilities. Through cold start and multi-stage training, the R1 model ultimately possesses strong inference performance while also performing well in readability.
The R1 series models provide a feasible direction for RL Scaling Law. In fact, when OpenAI launched the o1 model, it was discovered that inference performance steadily improved with training time and testing time calculations, known as the "RL Scaling Law." However, the industry has yet to achieve good results through methods such as Process Reward Models (PRM) and Monte Carlo Tree Search (MCTS), and the R1 technical report further mentions the challenges of scaling PRM and MCTS Issues such as reward deception.
The technical report of the R1 model provides a multi-stage training approach, where in the first stage of the RL process, researchers can enhance model performance by expanding the RL training set, or for a verifiable "RL Scaling law" direction; OpenAI's Chief Researcher Mark Chen also acknowledged that "DeepSeek has indeed independently discovered some core ideas of o1."
The idea that distillation enables small models to possess strong logical reasoning abilities may differ from OpenAI's o1-mini. According to Zhang Junlin's analysis, the o1 series models are more likely to be retrained (OpenAI has repeatedly emphasized that o1-mini has strong logical reasoning abilities but is weak in world knowledge; if it is based on the GPT series models, its world knowledge should not be weaker than GPT 4o-mini), while DeepSeek-R1 is obtained through reinforcement learning training based on V3.
Therefore, DeepSeek significantly enhances the reasoning ability of small models by distilling the outputs of DeepSeek-R1 into more efficient small models, likely taking a different path from OpenAI's o1-mini, thus effectively breaking the previous research conclusion that "the logical reasoning ability of small models is difficult to improve through distillation."
At this point, small models are expected to decouple language, world knowledge, and logical reasoning abilities through a "capability divide and conquer" (DCA) model, where language ability relies on the small model itself, logical reasoning relies on RL + distillation, and world knowledge relies on external RAG, thereby possessing the capabilities of the currently most powerful models, making deployment more user-friendly for small and medium-sized developers.
We believe that the core breakthroughs of the DeepSeek-V3/R1 series models are:
Technological and architectural upgrades significantly optimize model training costs, with engineering optimizing the MoE model architecture; it is expected that future manufacturers will continue to optimize attention head architectures around the MoE model;
The Group Relative Policy Optimization algorithm (GRPO) essentially relies only on the model's recent iterations, achieving "reflective capability";
It provides a concrete and feasible direction for "RL Scaling law," which manufacturers may follow up on and continue to explore other directions;
Distillation enables small models to possess strong logical reasoning abilities, which is expected to promote small and medium-sized developers to launch related applications.
2.2 Question 5: What are the technological innovations of the Janus series models?
The Janus series models alleviate the conflict between multimodal understanding and generation, enhancing model performance.
There is an inherent conflict in multimodal understanding and generation tasks regarding the need for visual encoders. In understanding tasks, the purpose of the visual encoder is to extract and represent high-level semantic information; whereas generation tasks primarily focus on generating local details while maintaining global consistency in the image, thus requiring a low-dimensional encoding representation of spatial structure and texture details. The core technology of the Janus series models lies in decoupling multimodal understanding and generation, alleviating the conflict through two independent visual encoding paths, thereby improving the model's performance and scalability.
There is no consensus on the architecture of multimodal generation models, with autoregressive and diffusion models continuing to evolve.
Currently, image generation models mainly include three types of architectures: autoregressive generation represented by Transformers, diffusion models represented by DDPM, LDM, DiT, and masked autoregressive image generation represented by MaskGIT, MAR. Autoregressive architectures generate pixels one by one through algorithms, with DeepSeek's Janus series model being a representative; masked autoregressive optimizes the number and order of pixels generated at once, improving the speed and performance of autoregressive models; diffusion models, represented by Sora, represent image generation as a process of transforming a noise image into a target image, with input and output being complete images throughout. Currently, both autoregressive and diffusion models are experiencing continuous breakthroughs in cutting-edge technologies, leading to sustained improvements in model capabilities.
We believe that multimodal models are still in the process of technological exploration. The core of the Janus series lies in providing a decoupled architecture for understanding and generation, which has improved model performance to some extent. Future developments in autoregressive and DiT technologies will further enhance the performance of multimodal models.
2.3 Question 6: What are the characteristics of the DeepSeek dataset?
Synthetic (generated) data plays an important role in the training of large models. In the context of high-quality training data being exhausted and the internet being flooded with a large amount of noisy data, synthetic data has become an important source of datasets in the training process of large models. As of September 2024, there are over 1,000 datasets labeled as "synthetic" on the Hugging Face platform. Specifically, synthetic data is mainly generated by algorithms and models, providing richer and more targeted information for training large models, helping to expand model performance:
General large models: In the training of general large models, synthetic data is mainly used to enrich the dataset and enhance model performance. Taking the training of DeepSeek-V3 as an example, during the supervised fine-tuning phase, sample data is generated using the DeepSeek-R1 model, and after RL training, high-quality data is selected for final model training through rejection sampling, effectively improving the model's inference capabilities Inference Model: In the training of inference models, synthetic data is mainly used to optimize the training process. For example, DeepSeek-R1 utilizes R1-Zero generated + manually labeled data for fine-tuning during the cold start phase, and in the supervised fine-tuning phase, it collected about 600,000 inference-related training samples and about 200,000 inference-unrelated training samples through the V3 model. Additionally, the process of distilling R1 to smaller models is actually achieved through R1 generated data for supervised fine-tuning of the smaller models.
Multimodal Model: In the training of multimodal models, synthetic data can improve data quality and significantly enhance visual generation capabilities. Janus-Pro introduced approximately 72 million synthetic aesthetic data samples during the pre-training phase compared to Janus, achieving a 1:1 ratio of real to synthetic data, thereby accelerating model convergence speed and improving image generation quality. Kimi-1.5, as a multimodal large model trained through reinforcement learning, enhanced its reasoning and knowledge-based task answering capabilities with synthetic data during the pre-training phase and synthesized image-text interleaved data during the multimodal training phase.
The GRPO algorithm to some extent frees the model from the constraints of human experience.
As described in 2.1, the R1-Zero model continues the relative policy optimization algorithm (GRPO) of the DeepSeek-V3 group during the RL process. This algorithm optimizes policies through intra-group reward comparisons without the need for an additional discriminator, ultimately achieving a continuous improvement in average response length on the training set, allowing the model to naturally learn to solve reasoning tasks with more thinking time.
In fact, GRPO also has significant implications for the handling of RL datasets. Specifically, the PPO algorithm relies on a value model to estimate state values to help calculate advantage functions; whereas the GRPO algorithm only performs relative advantage calculations on the output language content, without the need to design a value model. The establishment of a value model itself contains human preferences, which limit the value of the dataset through human experience. The GRPO algorithm can essentially be seen as a self-play of the model's generated content, allowing the model to break free from the constraints of human experience, continuously expanding its performance by enhancing thinking depth, and ultimately possibly surpassing human levels.
We believe that the application of synthetic data in models such as DeepSeek-V3/R1/Janus aligns with the research trend of large models, and the GRPO algorithm further enables the model to break free from the limitations of human experience during the RL process, thereby maximizing the value of the dataset and advancing towards the path of AGI that surpasses human capabilities 2.3 Question Seven: Is the Scaling Law Effective?
The training-side Scaling Law drives continuous improvement in model capabilities, but still faces constraints from technology, computing power, and data. As early as 2020, OpenAI proposed the "Scaling Law" in a paper, which suggests that the ultimate performance of large models is primarily related to the amount of computation, the number of model parameters, and the amount of training data, and is largely independent of the specific structure of the model (number of layers/depth/width).
Under the concept of the "Scaling Law," the industry pursues the use of more high-quality data on the training side to train models with larger parameter scales. Especially with the support of parallel computing in the MoE architecture, the parameters of large models can even exceed trillions, greatly enhancing model performance.
However, constrained by technology, computing power, and data, the training-side "Scaling Law" is facing bottlenecks:
Training models with higher parameter scales is relatively complex: When the parameter scale reaches trillions, the technical methods for further adjusting the model still need breakthroughs;
The scale of computing power somewhat restricts model development: NVIDIA's H100 can currently achieve full interconnection with 32,000 cards in a single cluster, but errors occur once every two hours (Founder Park interview with Shixiang Technology CEO Li Guangmi). Once the computing power cluster increases to 100,000 cards, errors may occur every 20-30 minutes, requiring high operational capabilities for the data center; otherwise, the utilization rate of computing power will significantly decline. At this point, more powerful computing cards are needed.
Lack of high-quality data: There have been reports that large model training has exhausted high-quality data. Therefore, simply increasing the training set size often results in a significant portion of repeated data, limiting the improvement of model capabilities. Moreover, data synthesis technology has not yet made breakthroughs, which also somewhat restricts model development.
Thinking chain and other methods open up space for improving the reasoning capabilities of large models.
As the progress of the training-side "Scaling Law" slows down, OpenAI released a series of new models, o1, in September 2024, which utilize reinforcement learning technology to significantly optimize model performance by increasing the reasoning time. It can also generate high-quality data during the training process, addressing the issue of natural data scarcity. For example, the thinking chain technology parallels the human thought process, allowing large models to break down complex problems into several simple steps during reasoning, gradually generating correct answers based on the user's questions.
The performance of OpenAI's o1 model steadily improves with training time and testing time calculations, and the depth of thought (time) in the post-training and reasoning phases may become the new "Scaling Law." Compared to OpenAI's non-open-source reasoning algorithms, the DeepSeek-R1 series models provide a feasible direction for RL Scaling Law, which is expected to encourage various vendors to follow suit and continue exploring other expansion directions on the reasoning side
The Scaling Law progresses along three paths simultaneously, aiding in the continuous improvement of model performance. As Jensen Huang, CEO of NVIDIA, mentioned in his keynote speech at CES 2025, after the launch of the o1 model, the Scaling Law for large models has effectively divided into three paths:
Pre-Training Scaling: Corresponding to the conclusion proposed by OpenAI in 2020, the larger the training data scale, model scale, and computational resources invested, the better the performance of the AI model will be. Although Pre-Training Scaling is currently facing bottlenecks due to technology, computing power, and data limitations, more powerful foundational models remain the main direction pursued by various manufacturers. The technical report of DeepSeek-R1 also states, "The reasoning patterns discovered by larger foundational models are crucial for enhancing reasoning capabilities." In the future, with optimizations in areas such as MoE architecture and model infrastructure, Pre-Training Scaling is expected to continue to develop.
Post-Training Scaling: This includes techniques such as reinforcement learning and human feedback, optimizing model performance by inputting a large number of high-quality prompts. In practice, limited by human work efficiency, the original human feedback reinforcement learning (RLHF) faces challenges in scaling (for example, the low efficiency of manually labeled data and inconsistent standards among different labelers), while DeepSeek-R1's pure RL technical solution effectively breaks this limitation, providing a feasible solution for Post-Training Scaling for various manufacturers.
Test-Time Scaling: This emphasizes reallocating resources, considering how much computing power to invest during the inference phase, and using a chain of thought to break down problems into smaller steps to solve them one by one. By thinking more deeply during the model inference phase, the model will possess stronger performance.
We believe that the Scaling Law remains effective, and the continuous iteration of RL technology brings new directions for the scaling expansion of model capabilities. In particular, DeepSeek has proposed pure RL and phased model training methods through architectural and technological innovations, achieving good performance. It is expected that various manufacturers will successively follow DeepSeek's algorithm direction and continuously adjust their architectures to explore more ideal model optimization methods.
3. DeepSeek-R1 promotes AI equity, and the industry chain enjoys development dividends
3.1 Question Eight: Does R1 mean that AI equity has been achieved? The open-source DeepSeek-R1 has sparked a global replication craze, with small models + RL achieving "reflection" emergence. Against the backdrop of the U.S. implementing AI chip blockades against China, DeepSeek has successfully trained the R1 inference model, which ranks among the top tier globally, at an extremely low cost. At the same time, DeepSeek has fully open-sourced the model weights, adhering to the very permissive MIT License open-source agreement, allowing other developers to use the model for commercial purposes and perform model distillation, which has been praised by Facebook's Chief AI Scientist, Yang Likun, as "the victory of open-source models over closed-source models."
Since the release of R1, leading teams worldwide have actively replicated it, achieving good results.
Among them, the team from UC Berkeley replicated DeepSeek R1-Zero in the CountDown game, achieving self-validation and search with a 3B base language model through reinforcement learning at a cost of less than $30; the team from Hong Kong University of Science and Technology replicated DeepSeek-R1-Zero and DeepSeek-R1 training on a 7B model using only 8K samples, achieving strong results in complex mathematical reasoning; even the Hugging Face team, the largest open-source platform globally, announced on January 26 that they have begun replicating all pipelines of DeepSeek-R1 and will open-source all training data and scripts upon completion of the replication.
Global tech giants are connecting to R1, and under the impact of DeepSeek, OpenAI's strategic direction may shift.
Despite U.S. concerns about DeepSeek's security and privacy issues, overseas giants such as NVIDIA, Intel, Microsoft, and AMD have integrated DeepSeek into their products; domestic companies like Silicon-based Flow and Huawei Cloud have also jointly launched the DeepSeek R1/V3 inference service based on Huawei Cloud's Ascend cloud services. In response to the global enthusiasm for DeepSeek, Sam Altman admitted that OpenAI's open-source strategy "stands on the wrong side of history" and stated that discussions are underway to open-source some models.
Additionally, on February 1, OpenAI urgently updated the o3-mini series, allowing even free users to experience the search function of o3-mini by selecting "Search+Reason." However, the current pricing for the o3-mini model is $0.55 per million input tokens (cache hit) / $1.10 per million input tokens (cache miss), and $4.40 per million output tokens, which is significantly higher than the R1 model.
Referring to the changes in Android and iOS market shares, the open-source ecosystem is expected to inject vitality into the AI industry. In the field of smartphone operating systems, the open-source nature of Android and the closed nature of iOS have led to distinctly different ecological models:
Android: The Android company was established in 2003, acquired by Google in 2005, and officially launched the Android operating system in 2007. Ecologically, the Android system is open-source, allowing numerous phone manufacturers to customize development based on its underlying architecture, increasing its market share from 2.8% in 2008 to 48% in 2011. However, this also brought a series of issues such as patent lawsuits, software piracy, and system security. In 2011, Google launched Android 4, and since then, Android devices have gradually become standardized, reaching a market share of 73.49% by December 2024.
iOS: Similarly, in the year Android was officially released in 2007, Apple launched the first generation iPhone equipped with the iOS system, marking the beginning of a new era for smartphones. Compared to the openness of Android, Apple's iOS system adopts a closed ecosystem, strictly controlling the software review process, which somewhat limits the system's flexibility but provides users with a consistent and high-quality experience. In terms of market share, iOS's market share has remained relatively stable in recent years, with a market share of 26.04% in December 2024, down from 35.56% in January 2009.
AI Industry: Drawing a parallel to the smartphone operating system field, the current AI industry is also facing a struggle between open-source and closed-source models. Referring to the development history of the Android system, the open-source model can attract developers worldwide to participate in AI technology innovation, allowing newcomers to quickly develop applications and iterate products based on existing achievements, thereby promoting the rapid implementation of AI applications and accelerating the development of the AI industry.
We believe that DeepSeek-R1, as an open-source model with performance close to the leading closed-source model o1, has already reflected a degree of AI equity. In fact, OpenAI's previous lead was largely based on first-mover advantage, and as the performance of open-source models catches up with closed-source models, the research and development capabilities of global teams can keep the performance of open-source models at the forefront. The recent active reproduction of the R1 model by various research teams further validates the advantages of the open-source model. Moreover, DeepSeek-R1 enables small models to possess reasoning capabilities, and lower costs will be more conducive for developers to explore the practical implementation of AI, leading to more valuable products.
3.2 Question Nine: What is the geometric impact of DeepSeek's emergence on the industry?
DeepSeek comprehensively influences the AI industry chain with its low cost and high performance. The AI industry chain can be roughly divided into three layers: the foundational layer (computing power, data, technology, etc.), the model layer (general/industry large models, development platforms), and the application layer (general/vertical applications, Agents, etc.). Although founder Liang Wenfeng stated that DeepSeek's technological breakthrough is just "one of the many innovations happening in the U.S. every day," its low cost, high performance, and the distillation method that brings powerful reasoning capabilities to small models, Still has an impact on the AI industry chain:
Computing Power: The explosive popularity of DeepSeek has brought attention to the economic term "Jevons Paradox," which refers to the idea that "improvements in fuel efficiency often lead to an increase in fuel consumption."
If this theory is extended to the field of computing power, the improvement in the efficiency of computing power applications by models may actually lead to an increase in the demand for computing power. In fact, the "Jevons Paradox" reflects a simple economic principle—when the price elasticity of demand is greater than 1, a decrease in price will lead to an increase in sales revenue. Therefore, the key to whether the demand for computing power increases under the influence of DeepSeek lies in the price elasticity of computing power, which is affected by the uses of computing power (generally speaking, the more uses a product has, the greater the demand elasticity).
As the underlying foundation of a new round of technological revolution, computing power will be applied across various industries. DeepSeek-R1 enables small models to possess strong logical reasoning capabilities through distillation, further accelerating the emergence of downstream applications. Thus, the price elasticity of computing power is more likely to be greater than 1, in line with the "Jevons Paradox," thereby maintaining robust demand. Additionally, Liang Wenfeng mentioned in an interview that the embargo on high-end chips may become a bottleneck, which also reflects the importance of self-controllable computing power chips.
Models: The breakthrough of the DeepSeek-R1 model actually reflects the narrowing gap between China and the U.S. in cutting-edge large models.
Taking GPT-4, released in March 2024, as an example, the Zhizhu GLM-4, released in January 2024, only reached 90%-100% of its performance on some benchmarks, with a model gap of over 10 months; whereas the R1, released in January 2025, is already close to OpenAI's o1 model released in September 2024, shortening the model gap to about 4 months.
The large models themselves and their corresponding Chat bot products have low user switching costs, leading to a "winner-takes-all" phenomenon. For instance, kimi achieved a context-lossless input length increase to 2 million words in March 2024, resulting in a significant surge in traffic; in December 2024, the popularity of Byte's Volcano Engine rose, and the release of DeepSeek-V3 also brought rapid traffic growth. Against this backdrop, it is expected that major companies will follow up on the research and development of DeepSeek model layers, and technology open-sourcing will also promote continuous investment from major companies, forming a positive feedback loop. Furthermore, DeepSeek has improved model performance through pure RL algorithms, architectural optimizations, and other methods, which may encourage more exploration by various manufacturers in related fields.
Application: DeepSeek-V3/R1 serves as a general-purpose/inference foundational model, with performance upgrades and improvements in various benchmark scores, which itself brings greater possibilities for application implementation.
However, for developers, a more critical point is whether the model can be adapted and tuned for applications, providing stable API services and more cost-effective token costs. Referring to the price war brought about by the release of DeepSeek-V2 in May 2024, even if the model costs are higher, major companies like ByteDance and Alibaba have significantly reduced prices according to the logic of burning money for subsidies, essentially because developers are price-sensitive, and large companies are willing to lose money to seize market share and cultivate developers' usage habits.
Considering that the development and invocation costs of DeepSeek-R1 are relatively low, and it has improved the inference capability of small models through distillation, application developers can deploy models or invoke APIs at a lower cost while maintaining relatively excellent performance. As the barriers to application development are lowered, it is expected that more product exploration directions will emerge, until a breakthrough "killer" application appears.
At the same time, the low price of DeepSeek-R1 is also expected to bring about a new round of price wars for inference models (the price of o3-mini has already validated this viewpoint), providing developers with more cost-effective choices. Finally, when the capabilities of the DeepSeek model reach the top tier globally, it can provide more stable services for domestic application developers (invoking GPT API may be subject to various restrictions), which will also promote the emergence of various applications.
Data: The training process of the DeepSeek series models still highlights the importance of high-quality data. For example, the V3 model was trained using 14.8 trillion tokens covering various fields and languages; R1 improved model performance and readability through carefully selected and processed cold start data; Janus-Pro also increased the number of samples for multimodal understanding by about 90 million and about 72 million for synthetic aesthetic data in visual generation compared to previous models. Considering the possibility of the RL paradigm, it is expected that high-quality data will still play an important role in model training.
4. Investment Recommendations
4.1 Question Ten: What investment opportunities will DeepSeek bring?
Computing Power: As the underlying foundation of a new round of technological revolution, computing power will continue to benefit from application demands across various industries.
With the addition of DeepSeek-R1 bringing generalization possibilities to the inference paradigm, it is expected that the computing power industry chain will continue to thrive under the technological explorations of various manufacturers. In addition, with the intensifying AI competition between China and the U.S., the importance of self-controllable high-end computing chips has further highlighted the need for domestic computing power. It is recommended to focus on the computing power segment centered on domestic computing and AI inference demand, especially in IDC, servers, and domestic chip-related industries Application: DeepSeek-R1 is expected to trigger a new round of price reductions for large model APIs, and small models equipped with strong reasoning capabilities through distillation will also encourage developers to explore more possibilities for application implementation.
AI applications, as a new generation of productivity tools, are optimistic about the continuous development of C-end software, while B-end application software is commercializing at a faster pace. It is recommended to focus on B-end Agents, where OA + ERP serves as the core entry point, making it easier to combine with AI, which is expected to commercialize first. Additionally, pay attention to software companies with a large user base, good ecosystems, and cloud capabilities.
Edge Side: The enhancement of small model capabilities has also promoted the deployment of edge models, and we are optimistic about the potential explosion of AI terminals as a new generation of computing platforms.
Firstly, we believe that AI + education, as a high-frequency application scenario, is likely to land first, especially with the Ministry of Education's actions to empower education with AI being progressively advanced, which is expected to drive demand for AI learning machines, AI education large screens, etc. We recommend companies like Shiyuan Co., Ltd. and iFLYTEK;
Secondly, we believe that the shipment of new terminals such as AI glasses, AIPC, and robots is expected to increase as the scope of use expands with model upgrades. Therefore, it is advisable to pay attention to terminal suppliers or internal core software suppliers represented by AI glasses, PCs, and robots.
Data: High-quality data remains an indispensable part of large model training, and the landing of B-end Agents also requires industry know-how for fine-tuning. It is recommended to focus on companies related to vector databases, data processing enterprises, and vendors with industry-specific professional data.
Risk Warning
(1) The commercialization of the AI industry may not meet expectations: Currently, the commercialization models of AI products at various stages are still in the exploratory phase. If the advancement pace of products at various stages does not meet expectations, it may adversely affect the performance of related enterprises;
(2) Market competition risk: Overseas AI manufacturers, leveraging their first-mover advantage and strong technological accumulation, are in a favorable position in competition. If domestic AI manufacturers' technology iterations do not meet expectations, their operating conditions may be affected. Additionally, many domestic companies are currently investing in AI product research and development, which may lead to risks of homogenized competition in the future, thereby impacting the revenues of related enterprises;
(3) Policy risk: The development of AI technology is directly influenced by the policies and regulations of various countries. As AI penetrates various fields, governments may further introduce corresponding regulatory policies to standardize its development. If companies fail to adapt to and comply with relevant policies in a timely manner, they may face corresponding penalties or even be forced to adjust their business strategies. Furthermore, policy uncertainties may lead to errors in corporate strategic planning and investment decisions, increasing operational uncertainties;
(4) Geopolitical risk: In the context of fluctuations in the global geopolitical environment, especially the United States' export restrictions on China, domestic companies' access to computing power chips may be directly affected, thereby impacting their product research and development and market competitiveness. At the same time, geopolitical risks may also create obstacles for AI products in expanding overseas markets, affecting the revenue situation of related enterprises