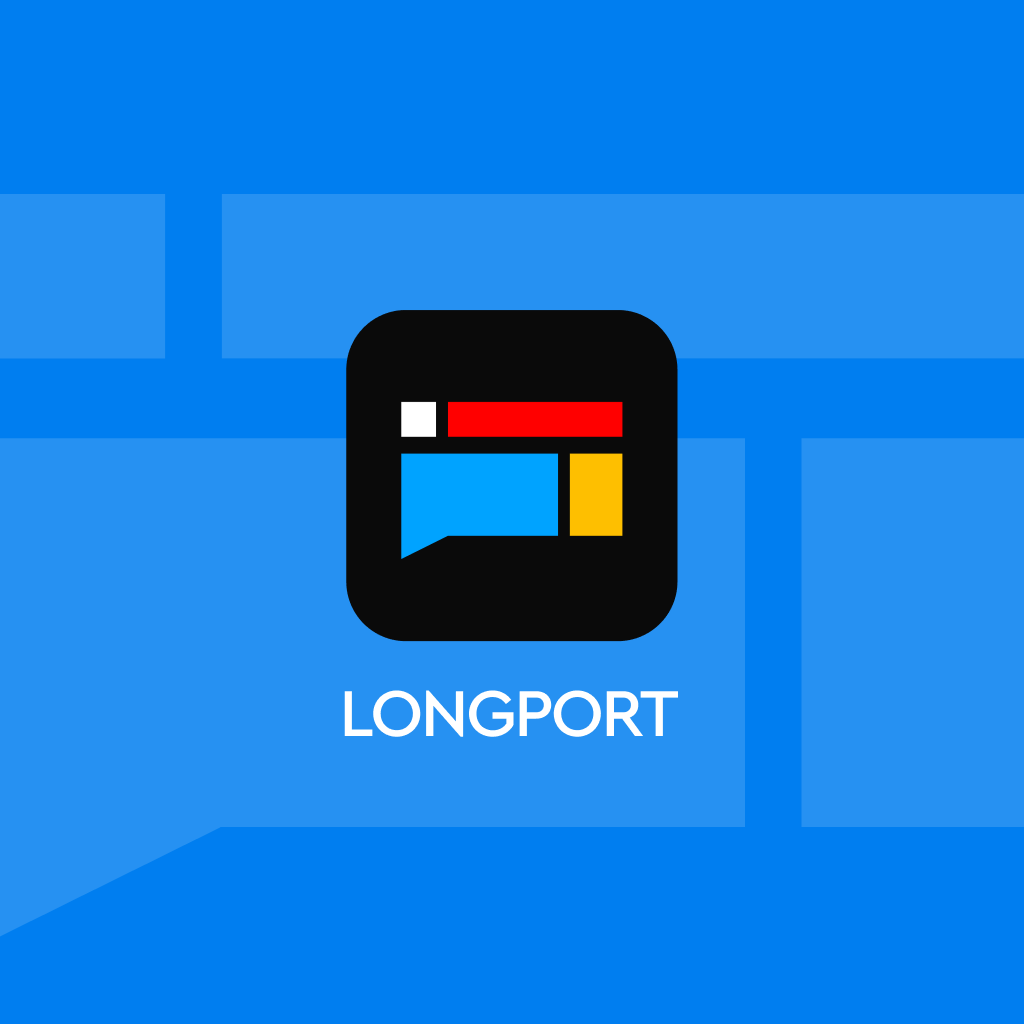
A comprehensive understanding: Which segments of the AI infrastructure industry chain are favorable for DeepSeek and which are unfavorable?
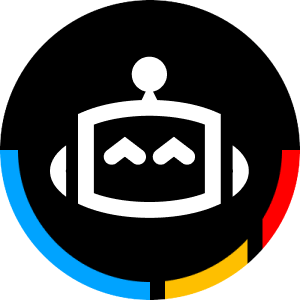
DeepSeek's cost-effective training technology has attracted market attention, with Citigroup analysts pointing out its impact on the AI infrastructure supply chain. DeepSeek's R1 model is expected to drive widespread adoption of AI models, reduce computing costs, and enhance return on investment. Despite challenges in certain areas, overall AI infrastructure construction will continue to grow robustly. The popularization of the inference stage brings new opportunities for GPUs, ASICs, etc., while reduced training demand may put pressure on Retimers and others. AI infrastructure will become a core driving force in the global technology industry
DeepSeek shakes Silicon Valley, with its cost-effective training technology attracting widespread market attention.
In the latest research report, Citigroup analysts Atif Malik, Asiya Merchant, and others detailed the potential impact of DeepSeek on various segments of the AI infrastructure supply chain, revealing which segments will benefit and which may face challenges.
Citigroup stated that DeepSeek's R1 model is expected to drive the widespread adoption of AI models, especially in consumer and enterprise markets, as computing costs decrease, the potential return on investment (ROI) for AI will significantly increase.
At the beginning of the report, Citigroup first defined scaling laws and pointed out that the latest view holds that scaling laws have three stages: pre-training, post-training, and test-time scaling. Among them, DeepSeek is an excellent example of "test-time scaling."
Pre-Training: The process of training machine learning models on large datasets to generate general features.
Post-Training: A series of techniques to optimize the model through reinforcement learning, human feedback, and other methods after pre-training.
Test-Time Scaling: Extending the model's thinking process during the inference phase through multi-step reasoning.
For the AI infrastructure supply chain, the impact of DeepSeek's innovative technology is multifaceted. Citigroup believes that while certain segments may face short-term challenges, overall, the construction of AI infrastructure will continue to maintain strong growth. The popularization of the inference phase will bring new opportunities to segments such as GPU, ASIC, and DCI, while the reduction in training demand may exert some pressure on segments like Retimers and optical modules.
As AI technology continues to advance, the construction of AI infrastructure will become one of the core driving forces in the global technology industry. DeepSeek's innovation not only promotes the popularization of AI models but also brings new opportunities and challenges to various segments of the AI infrastructure supply chain.
Impact on Various Subfields of AI Infrastructure
GPU: Neutral.
As the core hardware for AI training and inference, the market demand for GPUs has remained strong. Citigroup believes that although DeepSeek's technology may reduce the demand for large-scale training, the computational demand during the inference phase will increase, thus keeping the overall GPU market neutral.
ASIC (Application-Specific Integrated Circuit): Neutral to Positive.
ASICs perform particularly well in the AI inference phase. Citigroup expects that as inference demand increases, the market share of ASICs will gradually expand. Although the reduction in training demand may have some impact on the ASIC market, in the long run, the growth in inference demand will offset this negative impact. ASICs may ultimately be more related to inference, so the potential reduction in the training phase may be offset by future growth in inference Retimers: Neutral to Negative.
Retimers are primarily used for high-speed data transmission, especially during the AI training phase. Citigroup pointed out that as AI computing shifts from training to inference, the demand for Retimers may decline, as the computational intensity during the inference phase is lower and the need for high-speed data transmission is relatively reduced.
Optical Modules (Intra Server/DC): Neutral to Negative.
Similar to Retimers, the demand for optical modules is high during the AI training phase. Citigroup believes that as the inference phase becomes more prevalent, the market demand for optical modules may be affected, particularly in terms of connection needs within data centers.
DCI (Data Center Interconnect): Positive.
DCI is relatively unaffected by training and inference, as it is not closely related to the specific details of AI models and workloads. Citigroup believes that the demand for DCI is relatively unaffected by changes in the ratio of training to inference, and the growth of the inference phase will bring new opportunities for DCI.
Switches: Neutral to Positive.
As a core component of data center networks, the market demand for switches is closely related to the proliferation of AI computing. Citigroup pointed out that although the reduction in training demand may have a short-term impact on the switch market, the growth of the inference phase will drive higher network bandwidth demand, and in the long run, the switch market will remain neutral to positive.
Connectors: Neutral to Positive.
Similar to switches, connectors are considered unrelated to training and inference, with their market demand closely tied to the overall growth of AI infrastructure. Citigroup believes that as AI computing becomes more widespread, the demand for connectors will remain stable, especially with the push for distributed computing, the connector market is expected to see new growth points.
Storage: Neutral to Positive.
Storage OEMs have not yet been affected by AI spending, and discussions about AI's impact are still premature. Citigroup pointed out that although the current impact of AI on storage devices has not fully manifested, the acceleration of the inference phase will drive demand in areas such as data management, data movement, and data security, thereby boosting the storage market.
Server OEMs: Neutral.
Citigroup believes that although the demand for AI training servers may decrease, the demand for inference servers will increase, and overall, the server OEM market will remain neutral.
PC/Smartphones: Neutral to Positive.
With the optimization of AI models, the AI computing capabilities on local devices will be enhanced. Citigroup pointed out that this will drive the upgrade of PCs and smartphones, especially for devices with higher computing capabilities, where market demand will remain neutral to positive.
Risk Warning and Disclaimer
The market carries risks, and investments should be made cautiously. This article does not constitute personal investment advice and does not take into account the specific investment goals, financial situation, or needs of individual users. Users should consider whether any opinions, views, or conclusions in this article align with their specific circumstances. Investment based on this is at one's own risk