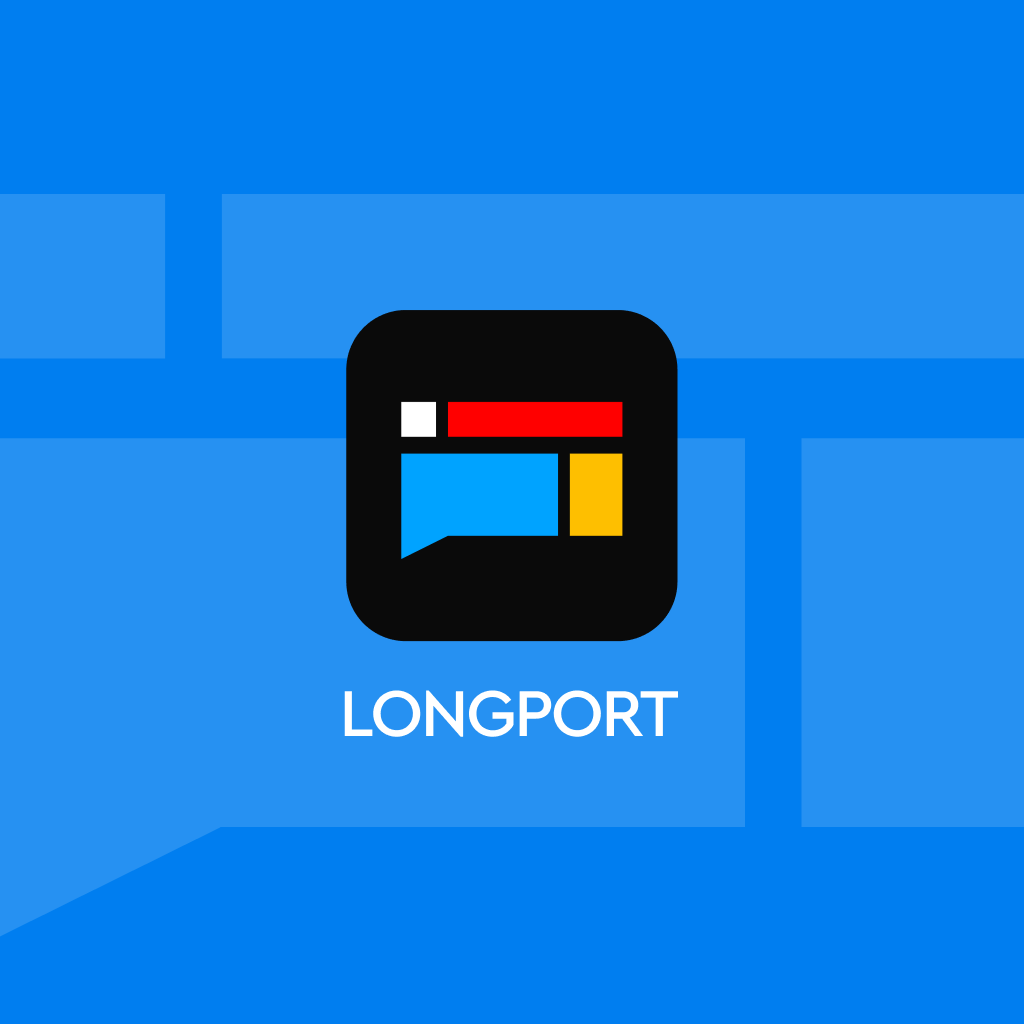
The Verification Logic of AI Grand Narrative
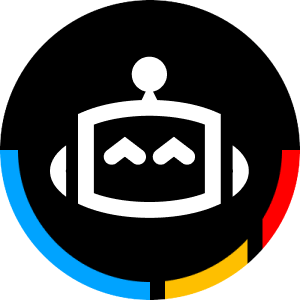
This article explores the verification logic of the grand narrative of AI, emphasizing the need for validation through mathematics and research data beyond the grand narrative. The current mainstream view suggests that reinforcement learning may replace the computational power requirements of pretraining in the early stages of Scaling Law, and that AGI will emerge within the next three years, with agent products replacing human value. Although applications have not yet exploded, the progress in reinforcement learning has extended the training lifecycle, presenting both challenges and opportunities for the industry
In the past few days, I have learned many grand narrative-specific terms from various Sellside comments and domestic self-media, such as Jevons paradox, Sputnik moment, global technology diffusion, and cost reduction leading to accelerated AGI.
Too many grand narratives inevitably lead to empty exchanges. We are a serious research team and do not want to over-discuss from the perspective of grand narratives.
This article does not intend to confirm or refute; it merely feels that beyond the grand narratives, we also need to attempt to verify using mathematical/accounting methods and research data points as anchors for tracking our progress.
At least from my perspective, after experiencing the debates over the past few days, the entire industry has become increasingly susceptible to stimuli, with an increasingly fragile investment environment.
The current mainstream grand narrative is:
-
Reinforcement learning is still in the early stages of Scaling Law, which will completely replace the computational power used in Pretraining.
-
Cost reductions brought by models like Deepseek will ultimately stimulate Token usage significantly, leading to accelerated catalysis of the application ecosystem, and the total amount will be larger than training.
-
We will see AGI within the next three years, with Agent products replacing human value and contributing significantly to consumption. The question of AGI is no longer whether it will happen, but when it will happen.
This has changed the perception of the grand narrative we heard a year ago, which was:
-
The progress of Agents and applications is more gradual, and there may be an Air Pocket between large-scale reasoning and the slowing growth of training budgets.
-
The timing of the Air Pocket may coincide with the discovery that AI can handle relatively simple scenarios such as Coding, Math, and customer service, but extending to more complex scenarios will take longer.
-
The future is very bright, but the process may still follow the Gartner curve, going through a phase of overheating → cooling → maturity.
The shift in grand narratives is due to:
-
The main supply chain is performing well, showing no signs of an Air Pocket.
-
Although we have not yet seen an explosion in applications, the logic of reinforcement learning based on marginal data improvements makes it easier to tackle vertical scenarios.
-
The lifecycle of training has also been further extended due to reinforcement learning.
1 Progress and Ceiling of Reinforcement Learning
In a previous article, we detailed the key elements of reinforcement learning: the quantity and quality of synthetic data.
During our tracking of Scaling Law, there have been several noticeable shifts in mindset.
In the first half of 2024 and prior, there was no dispute over Scaling Law; the logic of Pretraining is clearer and simpler than Posttraining. Each generation of models may require 2-3 years, but a 10x increase in parameter volume brings corresponding improvements By mid-2024, we began to observe in individual cases that the usage of reinforcement learning had already surpassed Pretrain, and this was a very good path for generating synthetic data, which would ultimately feed back into Pretrain. Therefore, at that time, we were very optimistic, as the Scaling Law had two driving curves.
In the fourth quarter of 2024, we noticed some changes:
-
The path of reinforcement learning feeding back into Pretrain seems less clear than before and is difficult to generalize.
-
Despite countless attempts, the returns from investing additional computational power into Pretrain have significantly decreased. This is mainly due to the depletion of high-quality raw datasets, while synthetic data (a potential solution) has yet to deliver satisfactory results.
-
However, although Pretrain has hit a wall, the Scaling Law still has an early curve for reinforcement learning, and we mentioned that reinforcement learning has not even reached the stage of GPT-3.
-
At this stage, our thoughts are very consistent with the current mainstream narrative logic.
In the past month, we have seen some more changes:
-
Reinforcement learning also faces data constraints, the current data generation methods still lean towards manual processes, relying on human annotations. Meanwhile, the methods for problem-solving are primarily applicable to coding and math, making it difficult to generalize further.
-
If we continue to use the current synthetic data production scheme, the marginal costs will increasingly rise, leading to issues with both Data Efficiency and Data Quality.
-
However, we are still uncertain whether the exponential increase in training power brought by GB can solve the Data Efficiency problem, while also addressing the Data Quality issue through extensive experimentation, ultimately leading to generalizable Self-play, breaking through the data bottleneck, and surpassing human intelligence.
-
Additionally, it should be emphasized that the recently discussed Deepseek R1-zero differs from what we refer to as self-play, as it still heavily relies on data generated by humans, essentially aligning with human efforts, and we need to face the development of technology under a grand narrative.
Thus, the most critical validation point here is whether, after the volume of GB, extensive experimentation can truly lead to genuine Self-play. This time point is very close, likely in Q2-Q3, when a clear conclusion may emerge (unless the large cluster of GB going fully online further delays this).
Before this validation point, we believe that all large model companies need to make substantial computational reserves to validate this logic, in other words, this period is relatively safe for CAPEX.
However, as we approach the validation point, the risks of uncertainty also increase
2 Cost Reduction Leading to Usage Stimulus - Jevons Paradox
Fuel, coal, and electricity are typical Jevons paradox commodities, and their continuous price reductions have stimulated greater demand.
IaaS products are similar; leading CSPs reduce prices by 5-8% annually, and through performance improvements relative to OnPrem, they ultimately achieve stable growth.
Large model APIs are still very close to the PaaS products of the past software industry. The logic of cost reduction stimulating usage accompanies every stage of PaaS products.
We have heard similar stories in various PaaS products with different barriers such as CDN, SMS, RTC, and databases: "Price reductions will lead to greater usage, resulting in accelerated revenue growth."
A recent story across all Consumption SaaS is that since 2022, all customers have begun to feel that the pricing of Consumption SaaS is too expensive. Consumption SaaS has also started to respond to customer demands and has begun to sketch a new big picture for all our investors, "Price reductions stimulate more usage, benefiting all without harm; we will soon accelerate growth again."
This acceleration in growth took a year at the shortest and three years at the longest.
As for LLM APIs, I also believe they will ultimately conform to the endgame of Jevons paradox, but the path in between may still be quite tortuous.
This requires very good rhythm control. Currently, the average cost reduction of APIs each quarter is about 20-30%. This means that API prices will decrease by 70% each year; in other words, a doubling of token growth is needed to maintain stable API revenue. A sixfold increase in tokens is required to double API revenue.
The models that have caused cost reductions in each quarter in the past include GPT4 Turbo, GPT4o, and GPT4o mini.
A too-rapid price adjustment may also lead to a 1-2 month dip (that's right, the AGI era is already much friendlier than the previous PaaS era, after all, it's a great era), and then it will take more time to recover until acceleration occurs.
So in this round, we may need to consider, if cost reduction does not necessarily lead to an immediate acceleration in the total reasoning computing capacity (it may still be gradual growth), which products' shares will increase when the narrative changes?
At the same time, it is necessary to distinguish whether it is better models or cheaper models driving usage in the current scenario.
In most 2C scenarios, a lower price means a lower trial-and-error cost, allowing for coverage of more customers, and there is no issue with this.
However, in 2B scenarios, customers already have stronger payment capabilities, and only better models will lead to more usage; the elasticity brought by price may be limited. For example, the Salesforce Agentforce product currently has a common customer discount of 20-30%, and the cost reduction of the model is unlikely to drive customers to pay 10% to stimulate more volume However, the improvement in the model's capabilities can not only lead to an increase in usage but also to a higher ASP.
Therefore, stronger O4, O5 models, or the Orion model may provide greater assistance for usage.
Returning to our observation time point, observing API growth is more direct than observing the progress of Agent companies.
This time point may be in February to March. The official version of O1 has reduced costs by 60% compared to O1 Preview, and after the emergence of O3, whether it can lead to an increase in usage is significant for inference elasticity.
Currently, after observing the usage of O1 Preview for 2 months, there has not been a surge in usage.
3 IT Spending and CAPEX Mathematical Logic
Mainstream narratives easily equate the two, but there is a significant difference in mathematical and accounting logic.
We have previously estimated OpenAI's training costs, which include depreciation costs for training in 2024-2026 of 3.6B, 8.6B, and 15B respectively. The depreciation cost for 2025 may be lower than OpenAI's original plan, considering that if the Stargate project can be successfully financed, the depreciation costs for 2025-2026 will continue to rise.
In the above assumptions, although training expenses still have a 70% increase in 2026, there is no longer growth at the CAPEX level. Training expenses align more with revenue growth trends; we assume OpenAI will still have doubled revenue growth in 2026. However, there is no doubt that in this arithmetic, 2025 is a super year for CAPEX, but it also leaves more questions for 2026.
We attempt to incorporate the impact of Stargate. It is still unclear how much overlap there is between Stargate and OpenAI's original CAPEX.
In the first batch of $100 billion Stargate TCO, 15% is for funding and operational costs. After deducting this, the CAPEX investments for 2025-2027 (including the above apple2apple, such as venues) are $10 billion, $25 billion, and $50 billion respectively. If half of the CAPEX in 2025-2026 comes from OpenAI's past CAPEX plans, that means the deduplicated CAPEX for 2025-2026 is $30 billion and $37.5 billion, with 2027 growing even faster.
In this arithmetic scenario, Stargate is very important; whether it can be successfully financed and launched determines the CAPEX narrative for 2026.
The same mathematical calculations can also be seen in the recent descriptions by the CEO of Anthropic.
Therefore, the biggest verification point here is the financing progress of Stargate and the reasonableness of its ROI. According to the current ROI estimate, the IRR of the largest computing power supplier, Oracle, is only 5-8%.
4 Sputnik moment
This topic is very debatable, and I don't want to elaborate too much.
But it seems more like the Sputnik moment of closed-source AI, rather than the Sputnik moment of the American AI industry.
Comparing the efficiency of Deepseek with North American large models, although it is impossible to provide an Apple-to-Apple answer, observing the practices of North American companies has indeed changed long-term thinking.
Regarding data optimization, OpenAI does almost no disclosure, and Deepseek has also written very little, with both sides shrouded in a fog of war, relying on guesses for comparison.
But even if OpenAI's efficiency is higher than Deepseek's, there is still a significant amount of computing power utilization space that OpenAI can explore.
OpenAI has the most efficient networking, NV babysitter-level support, the best cards, and the best configurations. It is possible that, during periods of minimal optimization, its efficiency is higher than Deepseek's, but Deepseek's engineering practices have still provided many optimization insights for North American large model companies.
Not to mention that companies like META, which were previously very rough in computing power applications, are also large model companies.
5 The most important
What is the most important verification point in the entire story?
It should be whether large-scale, generalizable self-play can be successfully implemented.
Author of this article: Bo Taijin, source: Consensus Crusher, original title: "The Verification Logic of AI Grand Narrative"
Risk Warning and Disclaimer
The market has risks, and investment should be cautious. This article does not constitute personal investment advice and does not take into account the specific investment goals, financial conditions, or needs of individual users. Users should consider whether any opinions, views, or conclusions in this article align with their specific circumstances Invest based on this information at your own risk