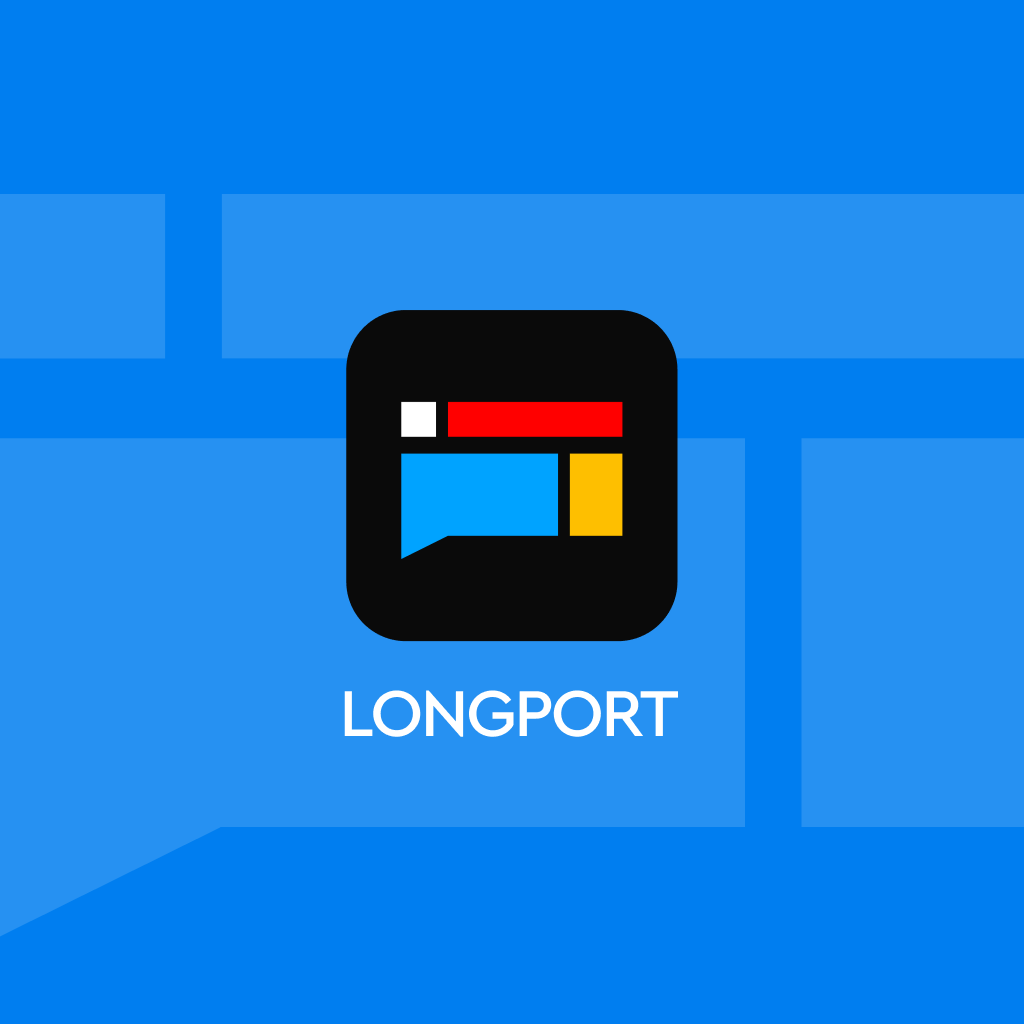
Wall Street Deep Research: Is DeepSeek the AI Apocalypse?
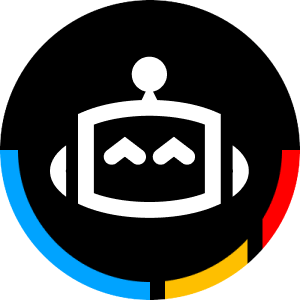
Against the backdrop of the "model scale law" continuously driving up costs, innovations such as MoE, model distillation, and mixed precision computing are crucial for the development of AI. Bernstein believes that the current demand for AI computing has not yet reached its ceiling, and the newly added computing power is likely to be absorbed by the ever-growing usage demand
During the Spring Festival, DeepSeek's next-generation open-source model sparked heated discussions with its astonishingly low cost and high performance, causing a seismic impact in the global investment community.
There are even claims in the market that DeepSeek "can replicate OpenAI for just $5 million," suggesting that this will bring about an "apocalypse" for the entire AI infrastructure industry.
In response, the well-known Wall Street investment bank Bernstein released a report after thoroughly studying DeepSeek's technical documentation, stating that this market panic is clearly excessive, and the claim that DeepSeek can "replicate OpenAI for $5 million" is a market misinterpretation.
Additionally, the bank believes that while DeepSeek's efficiency improvements are significant, they are not miraculous from a technical perspective. Moreover, even if DeepSeek indeed achieves a tenfold increase in efficiency, it would only correspond to the current annual cost growth rate of AI models.
The bank also stated that the current demand for AI computing has not yet reached its ceiling, and the new computing power is likely to be absorbed by the continuously growing usage demand, thus maintaining an optimistic outlook on the AI sector.
"Replicating OpenAI for $5 million" is a Misinterpretation
Regarding the claim of "replicating OpenAI for $5 million," Bernstein believes that it is actually a one-sided interpretation of the training costs of the DeepSeek V3 model, simply equating GPU rental costs with total investment:
This $5 million is merely an estimate of the V3 model's training costs based on a rental price of $2 per GPU hour and does not include initial R&D investment, data costs, and other related expenses.
Technological Innovation: Significant Efficiency Improvement but Not a Disruptive Breakthrough
Subsequently, Bernstein detailed the technical characteristics of the two major models released by DeepSeek, V3 and R1, in the report.
(1) Efficiency Revolution of the V3 Model
The bank stated that the V3 model adopts an expert mixture architecture, achieving performance comparable to mainstream large models with 2048 NVIDIA H800 GPUs and approximately 2.7 million GPU hours.
Specifically, the V3 model employs a Mixture of Experts (MoE) architecture, which is designed to reduce training and operational costs. On this basis, V3 also integrates Multi-Head Latent Attention (MHLA) technology, significantly reducing cache size and memory usage.
At the same time, the use of FP8 mixed precision training further optimizes performance. The combined application of these technologies allows the V3 model to achieve performance that meets or exceeds that of similarly sized open-source models while requiring only about 9% of the computing power during training.
For example, V3 pre-training requires only about 2.7 million GPU hours, while the same scale open-source LLaMA model requires about 30 million GPU hours.
- MoE Architecture: Activates only a portion of parameters at a time, reducing computational load.
- MHLA Technology: Reduces memory usage and enhances efficiency.
- FP8 Mixed Precision Training: Further improves computational efficiency while ensuring performance
Regarding the efficiency improvements brought by the V3 model, Bernstein believes that it is not a disruptive breakthrough compared to the common efficiency improvements of 3-7 times in the industry:
The focus of the MoE architecture is to significantly reduce the costs of training and operation, as only a portion of the parameter set is active at any one time (for example, when training V3, only 37B out of 671B parameters are updated for any given token, while all parameters in a dense model are updated).
Surveys comparing other MoE models indicate that typical efficiency is 3-7 times, while similarly sized dense models have comparable performance;
V3 appears to be even better than this (over 10 times), possibly considering some other innovations the company has brought to the model, but to regard this as a completely revolutionary idea seems a bit exaggerated and not worth the hysteria that has swept Twitter in recent days.
(2) The reasoning ability of the R1 model and the "distillation" strategy
DeepSeek's R1 model significantly enhances reasoning ability based on V3 through innovative technologies such as reinforcement learning (RL), making it comparable to OpenAI's o1 model.
It is worth mentioning that DeepSeek also employs the "model distillation" strategy, using the R1 model as a "teacher" to generate data for fine-tuning smaller models, which can compete in performance with models like OpenAI's o1-mini. This strategy not only reduces costs but also provides new ideas for the popularization of AI technology.
- Reinforcement Learning (RL): Enhances model reasoning ability.
- Model Distillation: Uses large models to train smaller models, reducing costs.
Maintaining Optimism for the AI Sector
Bernstein believes that even if DeepSeek indeed achieves a 10-fold efficiency improvement, it only corresponds to the current annual cost growth of AI models.
In fact, against the backdrop of the "law of model scale" continuously driving up costs, innovations such as MoE, model distillation, and mixed precision computing are crucial for the development of AI.
According to Jevons' Paradox, efficiency improvements often lead to greater demand rather than cost-cutting. The firm believes that current AI computing demand is far from reaching its ceiling, and the new computing power is likely to be absorbed by the continuously growing usage demand.
Based on the above analysis, Bernstein remains optimistic about the AI sector