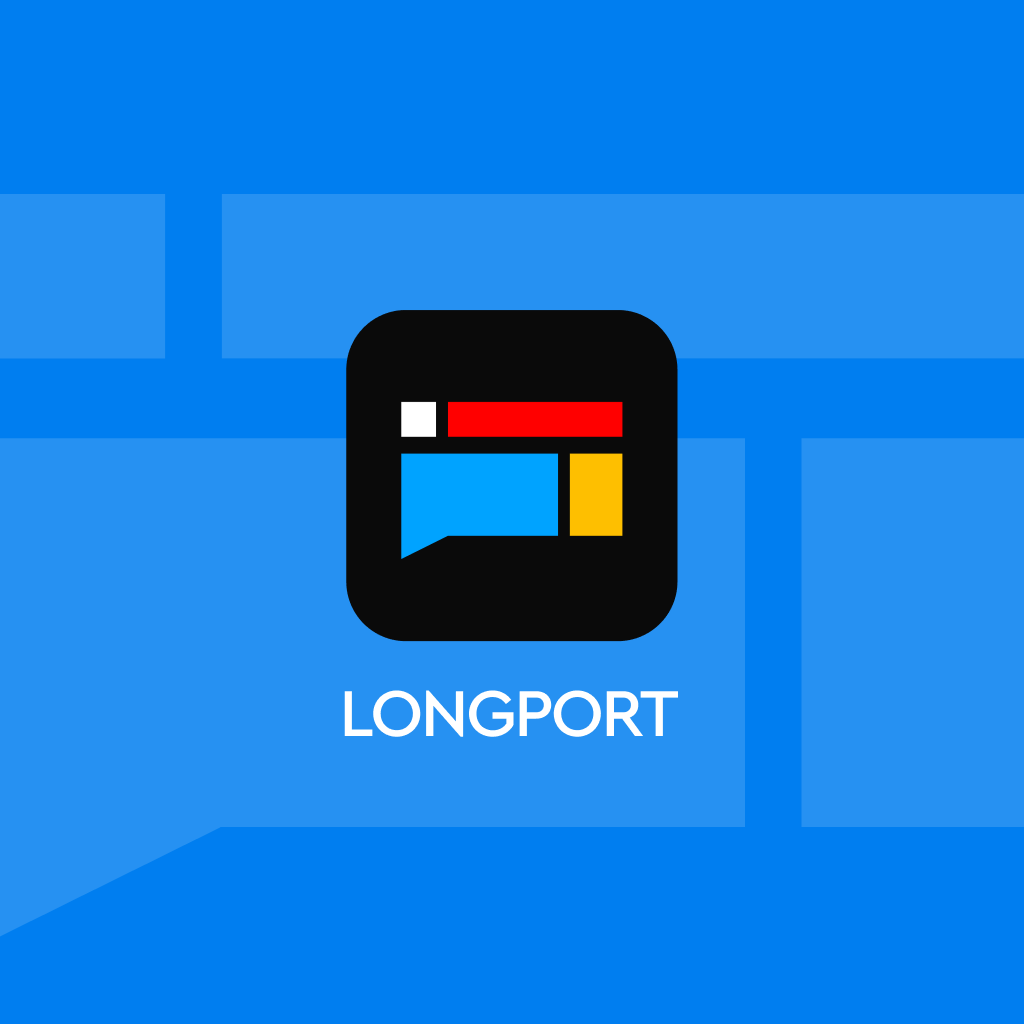
How American long-term investors of NVIDIA view the impact of DeepSeek
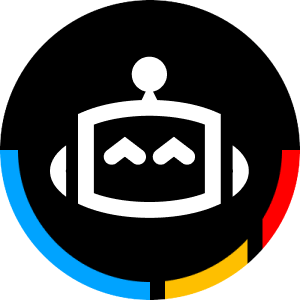
This article discusses DeepSeek AI's interpretation of Citrini Research's latest article, emphasizing China's innovation capabilities in the field of artificial intelligence. The open-source model launched by DeepSeek has performance close to the top levels of OpenAI and Anthropic, despite facing competition from NVIDIA and challenges from U.S. sanctions. The article points out that investors are concerned about the cost efficiency of these models, but the improvement in efficiency is a positive signal
Sansi Note: In this article, we haven't written a single word; the entire text is the interpretation of DeepSeek AI on the latest article from Citrini Research today. This American investment research institution is a long-term investor in NVIDIA and also joined the A/H semiconductor trading in June 2024.
I haven't changed a single word of the content output by DeepSeek; even the bold titles and formatting work were done by the AI. I have also included screenshots of DeepSeek's thought process for everyone to experience. The images may be a bit blurry, mainly due to WeChat compression. I have sent the original English text of the article along with this AI interpretation for comparison.
Since I also wanted to see more of DeepSeek's thought process, I asked a few more perspectives, which are: Author introduction -> Chinese title (I forgot to send the title when I uploaded the document, so it relied on its own understanding) -> Recommendation -> Full translation -> Content framework.
----
Chinese title (I forgot to send the title when I uploaded the document):
Recommendation:
Full Translation:
This week, two new open-source models from the Chinese artificial intelligence developer DeepSeek have attracted global attention, with performance comparable to the top benchmark levels of OpenAI and Anthropic.
We are not surprised by this. In fact, since last summer, we have anticipated that China would achieve breakthroughs through architectural innovation against the backdrop of hardware limitations:
"By focusing on research directions that rely less on NVIDIA (NVDA)-style hardware, they may find new ways to surpass the current paradigm.
However, it must be acknowledged that this will be a tough battle. NVIDIA's leading advantage is enormous, and U.S. sanctions are an insurmountable obstacle. However, from the journey of China rising as a technological superpower, we have learned the most important lesson: never underestimate them." The "Citrindex One-Year Report," published on CitriniResearch.com, June 2024
Ironically, the cost efficiency of these models has frightened investors and provided ammunition for those eager to declare the "end of the AI boom." However, both bears and bulls have missed the core of the issue.
Efficiency improvements are a good thing, and NVIDIA is not synonymous with AI. NVIDIA merely provides the critical hardware to meet the enormous computational demands of large language models (LLMs) and has become the core winner in the previous AI arms race.
Since we first covered the AI sector in May 2023, we have consistently focused on the investment strategy of "shovels and pickaxes" (i.e., "the first phase of the AI revolution").
While the commercialization path and product winners remain unclear, we choose to bet on known certainties—well-capitalized hyperscale cloud providers will invest billions of dollars in hardware and data center infrastructure. The latest capital expenditure guidance from Microsoft (MSFT) and Meta (META) indicates that this construction boom shows no signs of slowing down.
However, continuing to use the excuse of 'the future form of AI is unclear, so we default to investing in infrastructure' is becoming increasingly difficult. Although our focus has been on this for the past two years, our investment logic has never been 'data centers.' We cannot ignore technological evolution out of nostalgia for past successes. From the beginning, we have argued that once AI infrastructure is in place, large language models will move toward commercialization and democratization, thus opening the next phase of product development and real-world applications.
We also anticipate that the commercialization of large language models will become a key marker of the second wave of AI investment—shifting from infrastructure to application products. In this process, China will continue to play an important role by continuously improving LLM efficiency or challenging the CUDA ecosystem/training-specific chips (ASICs).
Whether directly or indirectly, breakthroughs from DeepSeek are significant progress in the evolution of AI, and investors should be wary of their potential impact.
Don't just take my word for it; here are the views of Microsoft CEO Satya Nadella:
Cheaper and higher-quality models may indeed impact companies that rely on capital expenditure to inflate valuations, but this is a significant boon for AI commercialization. In fact, high training costs (and to a lesser extent, inference costs) have always been the biggest obstacle to profitability in AI software. Perhaps through the democratization of top open-source models, demand will once again outstrip supply No matter the outcome of this debate (which we will explore in depth later), we are increasingly convinced that: The second phase of AI investment has begun.
But before diving into the discussion, let's first understand what DeepSeek is.
DeepSeek (Dummies Version)
DeepSeek has released two new models—DeepSeek-V3 and DeepSeek-R1—along with an instruction set called "R1 Zero," whose performance is on par with the top products from OpenAI and Anthropic. These models have caused a sensation on social media, not only because their capabilities rival or even surpass well-known systems but also because their API call costs are just a fraction of their competitors.
Twelve months ago, or even six months ago, DeepSeek was still relatively unknown to many. But that does not mean they are "amateur players"—the company has hundreds of engineers and has invested significant amounts of money and time (with pre-training costs alone reaching $6 million). Their self-developed DualPipe GPU communication system and other custom optimization technologies have significantly improved hardware efficiency, thereby reducing training costs.
Some of the mystery stems from speculation: DeepSeek may have secretly hoarded a large number of banned GPUs (they indeed have many), or China is launching a cognitive war (PsyOp) and fabricating data.
Before analyzing the investment impact, this article will use a lot of terminology. Given that the readers are not AI researchers, we have invited an AI expert to help simplify the explanations. Here are the core points (so even a typical "dummy" can understand):
The researchers at DeepSeek want to verify: Can we significantly enhance the mathematical problem-solving capabilities of AI without relying on massive data or complex tools?
They started with a model that already had mathematical problem-solving capabilities (Qwen2.5-Math-7B), discarding traditional supervised learning with manually labeled data in favor of reinforcement learning. This technology is not new, but its previous results were far from impressive.
Imagine this: every time the AI answers a question correctly, it earns a "gold star"—this simple feedback mechanism guides the AI to continuously optimize its problem-solving strategies. Even more astonishing is that they completed training with just 8,000 math problems, while other teams typically require millions.
Moreover, they did not use complex supervisory tools or multi-layer feedback loops; the system logic is extremely simple: "Correct answer = reward." Although it seems rudimentary, this AI's performance surpasses models trained with massive data and complex processes.
DeepSeek has also incorporated several clever ideas—such as compressing memory usage and predicting multiple sets of tokens simultaneously—to achieve training with high efficiency. This means they can achieve top performance without stacking expensive GPUs Such breakthroughs lower the cost threshold and prove that small teams can still compete with industry giants if trained properly.
In summary, DeepSeek proves that it is possible to create AI capable of complex tasks without huge amounts of funding, hardware, or human intervention. The debate over their actual investment has become irrelevant—by focusing on reinforcement learning and efficiency optimization, they demonstrate that powerful models can be created with limited resources.
They have made all training steps and code publicly available for others to replicate, making the so-called "review concerns" seem absurd. The final result is a lightweight model that can run locally on two Mac Minis, yet performs comparably to Anthropic and OpenAI.
This process is called "model distillation," which involves training a small "student model" to mimic the step-by-step reasoning logic and outputs of a large "teacher model."
DeepSeek (Technical Beginner Version)
DeepSeek's innovations have significantly streamlined the training and inference processes. By default, it uses 8-bit floating-point precision (FP8), combined with chunking strategies, multi-label prediction, and key-value (KV) index compression techniques, its GPU requirements are only a fraction of those of industry giants.
Its mixture of experts (MOE) architecture allows for the integration of massive parameters within a single model, but only a portion of the parameters are activated during each inference. For example, the 671 billion parameter DeepSeek-V3 can run on consumer-grade GPUs because typical inference tasks only require about 37 billion parameters to be activated.
This idea is not original.
Google proposed a similar concept in a paper in early 2021 through the Switch Transformer. However, due to practical challenges such as training instability, inefficient multi-expert routing, and difficulty adapting to consumer-grade hardware, this solution has not been widely adopted.
However, the R1 model proves that this technology can effectively achieve automatic tuning of dedicated models. Large-scale reinforcement learning without supervised fine-tuning allows the model to evolve through trial and error. In the eyes of some, this reconstructs the way LLM development is thought about.
Fewer parameters, smarter training, better inference, and crucially, no human feedback is required at all.
Moreover, this may open a crack to break NVIDIA's monopoly. DeepSeek's PCIe architecture indicates that they do not rely on NVLink or eight network cards per node.
What has not received enough attention is DeepSeek's R1 Zero instruction set (in homage to AlphaZero). They have demonstrated that reasoning can be computationally scaled using reinforcement learning alone (i.e., "thinking/reflection"), completely without the need for human-supervised fine-tuning.
This is already compelling in itself and also corroborates OpenAI's earlier claim that "this is just a language model" (which no one believed) — R1 Zero does not use complex mechanisms like Monte Carlo Tree Search (MCTS).
The "thinking process" of R1 Zero often appears illogical: it not only switches languages randomly but even generates text that is meaningless to humans. However, this has its rationale: the model merely converges to the global loss function's minimum, and the outputs are essentially the final representation serving humans.
In short, R1 Zero has found a better way of thinking by "cracking" the loss function.
Bold Speculation
Perhaps human readability is not the optimal solution for LLM reasoning and may even pose obstacles in certain scenarios. This adds a new dimension to the discussion of AI agents: is there a need to build a new application layer specifically designed for AI interaction on top of existing tools?
The R1 model also introduces another dimension: robust reasoning capabilities that rival top OpenAI models. Unlike the closed approaches of Anthropic or OpenAI, DeepSeek not only thoroughly discloses technical details but also adopts a permissive license for anyone to reproduce.
Its V3 model has made breakthroughs in mixed-precision training and MOE loss peak suppression, significantly reducing training costs. This further confirms that OpenAI's "just a language model" statement is not a smokescreen.
Transparency reveals how they guide the model to generate stepwise logic through pure reinforcement learning and carefully designed reward functions (rather than massive labeled data), supporting self-correction along the way.
They have also added language consistency checks to ensure that even if the model breaks complex tasks into multiple steps, the final output remains clear and coherent.
We have always patiently awaited the starting gun for the commercialization of LLMs, even asserting that this is a necessary prerequisite for the popularization of AI applications and the upgrade of agentic AI. Surprisingly, this turning point has come from China — previously, the AI field was thought to be dominated by a few Western giants, but now Chinese laboratories have entered the top ranks!
Such progress (which the West may follow) may signal a future where there are no discrete "new models," but rather large-scale mutual training, continuous optimization, and recursive self-improvement among systems. This process will accelerate to a point beyond human control, and ultimately we may not fully understand its optimization objectives.
The Qwen 1.5b r1 distilled version surpasses GPT-4o and Claude in certain reasoning tasks, which should not be overlooked. The democratization potential of AI reasoning driven by open source is also worthy of attention
Impact
So, is this a cognitive war in China?
We believe the publicly available data from its last training is credible, as it can be reproduced and verified. However, the total resource investment may be misleading, and the paper has nearly hinted at this.
But the leap in efficiency is undeniable.
The widely circulated view on social media over the weekend that "DeepSeek has ended the demand for NVIDIA chips" is incorrect. However, locally run distilled models will become more prevalent, which poses a downside for NVIDIA (part of the reason we have fully exited), but at the same time, it is a huge benefit for the overall development of AI and the expansion of use cases. Open source is an active choice of DeepSeek.
All of this makes us increasingly confident: China's breakthroughs in ASIC (Application-Specific Integrated Circuit) will reshape the hardware landscape (and answer our previous question about whether "China will flood the market with ASICs" — the answer is yes).
We remain optimistic about the Chinese semiconductor industry (although our optimism towards the U.S. has slightly decreased).
Our Outlook on the Future
At the very least, the market's pessimism towards Chinese AI may come to an end. This is favorable for us — we have positioned ourselves for China's positive developments and reinforced the view that "Chinese domestic semiconductors will break through in ASICs."
We initially included Chinese AI companies in our portfolio in May 2024 and doubled down on this in our October report titled "Another Brick in the Wall of Worries."
Here is their performance so far:
Secondly, if tool costs decrease, the era of AI agents will accelerate. Building a new layer specifically designed for AI interaction (meaningless to humans) on top of the existing application layer may be the optimal path. It is wise to position ourselves in companies that are likely to build or integrate massive agents. We believe that continuing with the "first phase" infrastructure strategy (first casting a wide net, then narrowing the scope as trends become clearer) will work again.
But we have talked enough about AI itself. I know you are all asking, "What does this mean for NVIDIA?"
The bearish logic is obvious: efficiency improvements will reduce the demand for GPUs — the capital expenditure frenzy of global cloud vendors over the past two years is based on this. This risk has been fully priced in.
Less discussed is another scenario — DeepSeek may actually become a positive for NVIDIA NVIDIA's Bullish Logic: Jevons Paradox
"Now we can have more AI."
——Dylan Patel, SemiAnalysis
Perhaps open-source and distilled models will spawn more AI startups, while the improvement in computing efficiency will ultimately be consumed by the surging demand.
Inference costs have shown a deep deflationary trend, but the market has not panicked as a result.
The bullish logic is: capital expenditure remains unchanged, but model performance far exceeds expectations. Why?
Because we are not optimizing on demand—expanding computing power will only stimulate demand to grow in sync. Larger clusters will still be built, but the result may be an accelerated entry into the "Matrix Era." Additionally, the threat of competition from China may further stimulate U.S. giants to increase their investments.
Our Position
We are not very enthusiastic about this—just look at the market's reaction to the announcement of "half a trillion dollars in space investment": the next phase of the arms race may take a different form, and the unpriced incremental capital expenditure space is already very small.
This is why we are liquidating our NVIDIA holdings—the risk-reward ratio is no longer attractive.
As we wrote in "The 25 Biggest Deals of 2025":
"We believe that NVIDIA's dominance will face substantial threats by 2025. These threats may not yet be commercialized, but will become part of the narrative, forcing valuation models to reflect risks. Although it still has significant competitive advantages (which may continue), we believe NVIDIA's risk-reward is no longer enticing.
The commoditization of AI training and inference workloads may become a reality next year, driving demand for high-cost-performance solutions, especially after the market passes the current explosive growth phase."
This does not even consider the market psychology during the hype cycle—we are undoubtedly in this cycle.
At the peak of the hype, fluctuations can be triggered without major changes, just by worries—worries about overbuilding, loss of pricing power, declining margins, or competition. Most of us are not experts when investing in AI, and success does not mean the market truly understands the technology.
Panic could erupt at any moment and lead to a crash.
But one thing must be clear:
Open-source, efficient, and commoditized LLMs are extremely beneficial for AI development, accelerating AI applications and innovations in real scenarios.
The cornerstone of the internet is the geek in the garage; more open-source means more geeks, which in turn spawns more AI products and use cases. Here is our current layout.
Investment Strategy Adjustments
What changes have been made to our AI investment portfolio?
You can check the adjustment details through the "Recent Changes" tab in the Citrindex table Core adjustment direction: Just as we cast a wide net in the first phase (buying 120 potential data center beneficiaries and eliminating 60-70% as trends became clearer), we believe it is now time to shift from the first phase to the second phase.
We are shorting SMH (semiconductor ETF) to increase holdings in targets that may benefit from the explosion of open source and AI agents, while also focusing on companies that may benefit from the "second phase requiring the construction of new layers specifically designed for AI agent interactions."
Ultimately, the first phase may continue, but the asymmetric return potential in the second and third phases is greater. The main risk is that these companies may fall behind or be replaced, but we remain confident in the Chinese software sector—intelligent agents are just the icing on the cake.
Here are the newly added potential beneficiaries for the second phase and the reasons:
We recommend revisiting our initial "AI Beneficiary Framework" and "AI Winners/Losers" report. Although some viewpoints may be outdated, reviewing the framework remains valuable when updating our understanding.
Full text framework:
Risk warning and disclaimer
The market has risks, and investment should be cautious. This article does not constitute personal investment advice and does not take into account the specific investment goals, financial situation, or needs of individual users. Users should consider whether any opinions, views, or conclusions in this article are suitable for their specific circumstances. Investing based on this is at your own risk