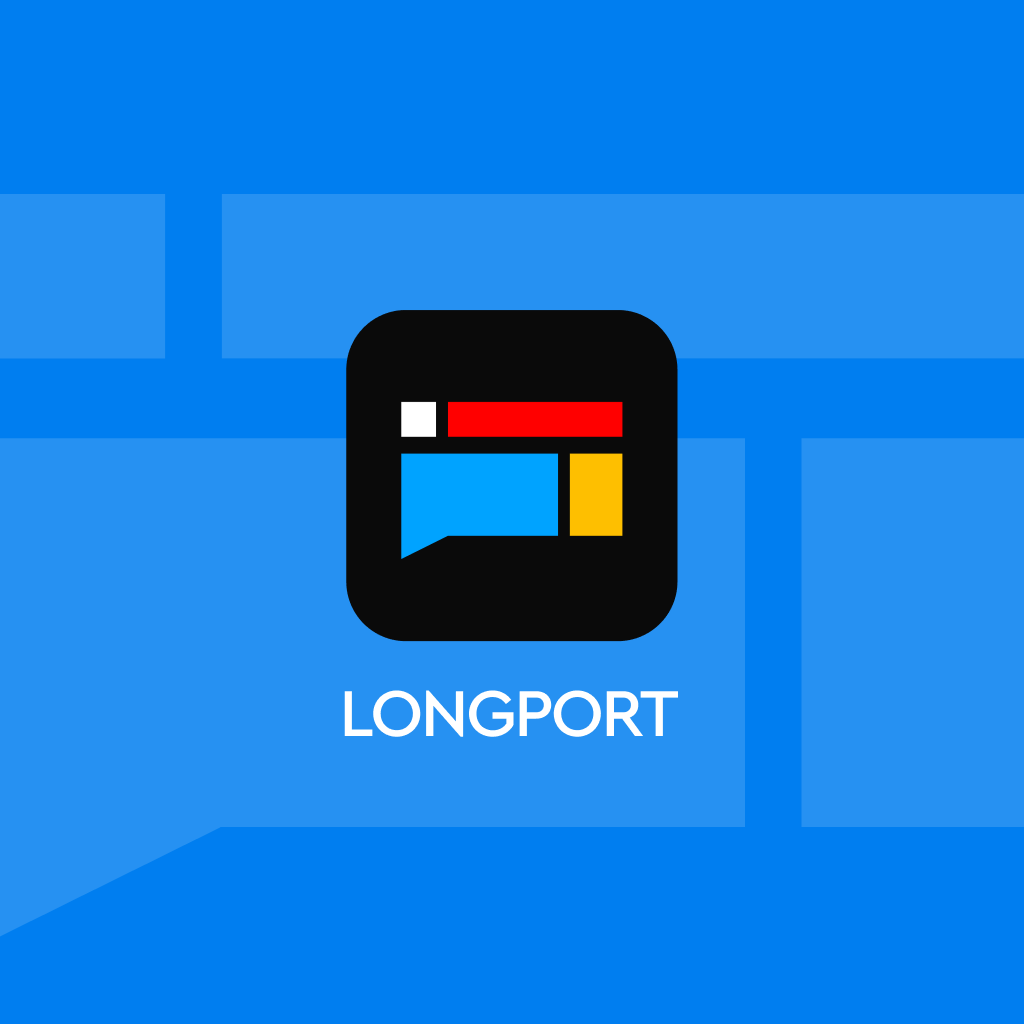
Scared Nvidia, burst the AI bubble, how does Wall Street view DeepSeek?
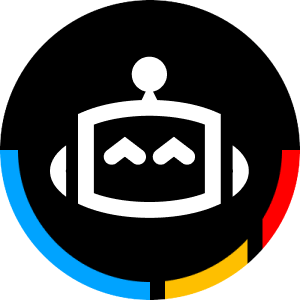
Wall Street believes that AI companies may face the risk of declining profits, and some compare this moment to the burst of the AI bubble, while others think that leading AI companies will not give up advanced GPUs. For the AI industry, artificial intelligence development has become easier, hardware requirements and costs will significantly decrease, competition is intensifying sharply, and the "moat" of tech giants is turning into a "small puddle."
DeepSeek exploded overnight, not only dominating the global internet but also causing multiple outages, even striking Wall Street, with analysts exclaiming that AI companies have reached their "Sputnik moment."
On Monday, U.S. stock futures plummeted before the market opened, with Nasdaq 100 futures down 5%, S&P 500 futures down nearly 3%, and Dow futures down over 1%.
Semiconductor stocks saw expanded losses before the market opened, with NVIDIA down over 13%, Broadcom down over 11%, ASML down nearly 11%, AMD down over 10%, and TSMC down nearly 10%. The entire European and American tech stock market could see a loss of $1.2 trillion in market value.
This collapse in U.S. stocks was triggered by DeepSeek, which just surpassed ChatGPT over the past weekend, outperforming industry-leading models in multiple mathematical and reasoning benchmark tests in terms of capability, cost, and openness, and quickly climbed to the top of the free app rankings in the Apple App Store in the U.S.
DeepSeek has sparked intense discussions across the internet, and what is truly noteworthy is its disruption of traditional AI capital expenditure scales.
The model was developed in just two months at a cost of less than $6 million. In contrast, the Meta team spends more than DeepSeek's entire training budget just on salaries, while OpenAI spends over $5 billion annually, and the "Interstellar Gate" project is generating an investment of $500 billion...
What does Wall Street think of DeepSeek? What does DeepSeek mean for the entire AI industry?
Wall Street: AI companies face declining profitability risks, and U.S. stocks are entering a moment of AI bubble burst
Jefferies analyst Graham Hunt's team pointed out that tech giants are facing questions about the rationality of capital expenditures, which may lead to a decline in data center revenue and profit growth trajectories:
Because DeepSeek can provide performance comparable to GPT-4o with only a small fraction of computing power, this may have a negative impact on builders, as AI participants face increasing pressure to justify the rationality of their capital expenditure plans, which could ultimately lead to a decline in data center revenue and profit growth trajectories.
We see at least two potential industry strategies: China is seeing more efficient training models that are being driven to innovate, further intensifying the competition for dominance in the AI field. For data center builders, the key question is whether they continue to adopt a “build at all costs” strategy to accelerate model improvements, or whether they will now shift focus to higher capital efficiency, putting pressure on the power demands and capital expenditure budgets of major AI participants. In the short term, the market will adopt the latter.
Short-term downgrade risk, with minimal impact on earnings. Although data center-related companies are susceptible to sentiment downgrades, their earnings will not be immediately affected. Any changes in capital expenditure plans have a lag effect, depending on the duration (>12 months) and the risk exposure in the order book. We believe the risk of changes or cancellations to existing orders is limited, and we expect expectations at this stage to shift towards higher investment returns driven by more efficient models. Overall, we remain optimistic about the industry, with scale leaders benefiting from an expanding moat and greater pricing power.
Mizuho mentioned that this occurs at a "painful moment":
We have just seen the Stargate announcement (expected to be around $500 billion by 2028) and Meta officially raising capital expenditures to $60-65 billion to expand Llama's scale, along with Microsoft's $80 billion announcement... The market now wants to know if this is the moment of the market's AI bubble burst (e.g., Cisco's internet bubble), with NVIDIA being the largest single company by weight in the S&P 500, accounting for 7%.
Citi analyst Atif Malik believes that AI companies will not abandon more advanced GPUs:
While DeepSeek's achievements may be groundbreaking, we question whether its accomplishments were achieved without fine-tuning using advanced GPUs and/or building the final model based on the underlying LLM through distillation techniques. Although the dominance of U.S. companies in state-of-the-art AI models may be challenged, we estimate that U.S. companies still hold an advantage in the inevitably stricter environment. Therefore, we expect leading AI companies will not abandon more advanced GPUs, and recent AI capital expenditure announcements indicate a demand for advanced chips.
The Bernstein report suggests that DeepSeek is actually discussing two series of models, but the talk of a price war is enough to exacerbate the already unclear investment returns for Mag7:
The first series is DeepSeek-V3, a mixed expert (MoE) large model that can deliver performance similar to or better than other large foundational models with significantly fewer computational resources through a series of optimizations and clever techniques. DeepSeek actually used a cluster of 2048 NVIDIA H800 GPUs for training for about 2 months. The often-cited figure of "$5 million" is calculated by assuming a rental price of $2/GPU hour for that infrastructure, excluding all other costs associated with prior research and experimentation related to architecture, algorithms, or data
The second model family is DeepSeek R1, which applies reinforcement learning (RL) and other innovative technologies to the V3 base model, significantly improving inference performance and competing fiercely with OpenAI's o1 inference model and other models. This model seems to have caused most of the anxiety. The DeepSeek R1 paper does not quantify the additional resources required to develop the R1 model.
Should V3's relative efficiency be surprising? As an MoE model, we don't think so... The significance of the Mixture of Experts (MoE) architecture lies in significantly reducing training and operational costs. Investigations comparing other MoE models indicate that V3's typical efficiency is about 3-7 times greater than that of similarly sized dense models with comparable performance; V3 appears to be even better than this (>10 times), possibly because the company has introduced some other innovations into the model. However, considering this as something completely revolutionary seems a bit exaggerated and not worth the uproar it has caused in the Twitterverse over the past few days.
But the talk of a price war is enough to exacerbate the already unclear return on investment for Mag7. DeepSeek's pricing is indeed far above its competitors, with the company's model priced 20 to 40 times cheaper than OpenAI's comparable models. Of course, we do not know the economic benefits of DeepSeek in this regard (and these models themselves are open, available for free to anyone who wants to use them), but the whole situation raises some very interesting questions about the role and feasibility of proprietary versus open-source efforts, which may warrant further research...
Significance for the AI Industry: Competition Intensifies Sharply, Tech Giants' "Moats" Become "Puddles," Disruption Accelerates
In terms of significance for the AI industry, Dropbox AI Vice President Morgan Brown made a series of summaries:
First, some background: Currently, the cost of training top AI models is extremely high. OpenAI, Anthropic, and others have spent over $100 million just on computing. They need large data centers with thousands of $40,000 GPUs, akin to needing an entire power plant to run a factory.
DeepSeek suddenly appeared and asked, "What if we do this for $5 million?" They are not just talking — they actually did it. Their models rival or even surpass GPT-4 and Claude on many tasks. The AI world is shaken.
How did they do it? They rethought everything from scratch. Traditional AI is like writing every number as a 32-bit decimal; DeepSeek asks, what if we only use 8-bit decimals? It's still accurate enough! Boom — memory requirements reduced by 75%.
Then there's their multi-label prediction; ordinary AI reads like a first grader: "This... cat... sits..." DeepSeek reads the entire phrase at once, twice as fast, with 90% accuracyWhen you are dealing with billions of words, this is important.
But what is truly smart is: they built an "expert system." They did not use a large AI trying to understand everything (like making a person simultaneously a doctor, lawyer, and engineer), but rather have specialized experts that only wake up when needed.
Traditional model: all 1.8 trillion parameters are always active, DeepSeek has a total of 671B, but can only activate 37B at a time. It's like having a large team but only calling on the experts truly needed for each task.
7. The results are shocking: training cost: $100 million → $5 million; required GPUs: 100,000 → 2,000; API cost: 95% cheaper; can run on gaming GPUs instead of data center hardware.
The craziest part is all open source, anyone can check their work, the code is public, and the technical papers explain everything.
Importantly, it breaks the model of "only large tech companies can get into AI," you no longer need a billion-dollar data center, a few good GPUs can do it.
This is terrifying for NVIDIA. Their entire business model is built on selling super expensive GPUs with profit margins up to 90%, and if everyone can suddenly do AI with regular gaming GPUs... well, you know where the problem lies.
The key is: DeepSeek's team is less than 200 people, but Meta's team has a salary that exceeds DeepSeek's entire training budget... and their model isn't that good.
This is a classic disruption story: incumbents optimize existing processes, while disruptors rethink fundamental approaches.
13. The impact is huge: AI development becomes easier; competition intensifies sharply; the "moat" of large tech companies looks more like a puddle; hardware requirements (and costs) drop significantly.
Giants like OpenAI and Anthropic will not stand still. They may already be implementing these innovations, but the efficiency genie is out of the bottle—there's no going back to the "just throw more GPUs at it" approach.
Final thought: this feels like a turning point we will look back on, just like personal computers made mainframes less important, or cloud computing changed everything. AI is about to become more widespread and cheaper. The question is not whether it will disrupt current players, but how fast the disruption will happen.
NVIDIA is facing unprecedented threats
Additionally, analysis indicates that NVIDIA is actually struggling to meet the high expectations of the market. Analysts state that NVIDIA faces unprecedented competitive threats, and the premium valuation of 20 times expected sales and 75% gross margin is becoming increasingly difficult to justify. The company's so-called "moat" in hardware, software, and efficiency is showing worrying cracks, as thousands of the smartest people on the planet, backed by billions of dollars in capital resources, are trying to attack them from all angles
In terms of hardware, the innovative architectures of Cerebras and Groq indicate that NVIDIA's interconnect advantage (the cornerstone of its data center dominance) can be circumvented through a complete redesign. More traditionally, every major NVIDIA customer (Google, Amazon, Microsoft, Meta, Apple) is developing custom chips, which could erode revenue from high-margin data centers.
The software moat also appears equally fragile, with new high-level frameworks like MLX, Triton, and JAX abstracting the importance of CUDA, while efforts to improve AMD drivers may unlock cheaper hardware alternatives. Most importantly, we are witnessing the emergence of LLM-driven code translation, which can automatically port CUDA code to run on any hardware target, potentially eliminating one of NVIDIA's most powerful lock-in effects.
Most shockingly, DeepSeek's recent efficiency breakthrough has achieved comparable model performance at about 1/45th the computational cost. This suggests that the entire industry has been massively over-provisioning computing resources. Coupled with the emergence of more efficient inference architectures through chain-of-thought models, the overall demand for computation may be much lower than current forecasts. The economic implications here are compelling: when DeepSeek can achieve GPT-4 level performance while reducing API call costs by 95%, it indicates that either customers are wasting money or margins must decline significantly.
The fact that TSMC will produce competitive chips for any well-funded customer naturally limits NVIDIA's architectural advantage. But fundamentally, history shows that the market will ultimately find ways to bypass artificial bottlenecks, leading to excess profits.
These combined threats suggest that NVIDIA faces far greater difficulties in maintaining its current growth trajectory and profit margins than its valuation implies. There are five different avenues of attack—architectural innovation, customer vertical integration, software abstraction, efficiency breakthroughs, and manufacturing democratization—at least one of which seems likely to significantly impact NVIDIA's margins or growth rates. At current valuations, the market has yet to price in any of these risks.