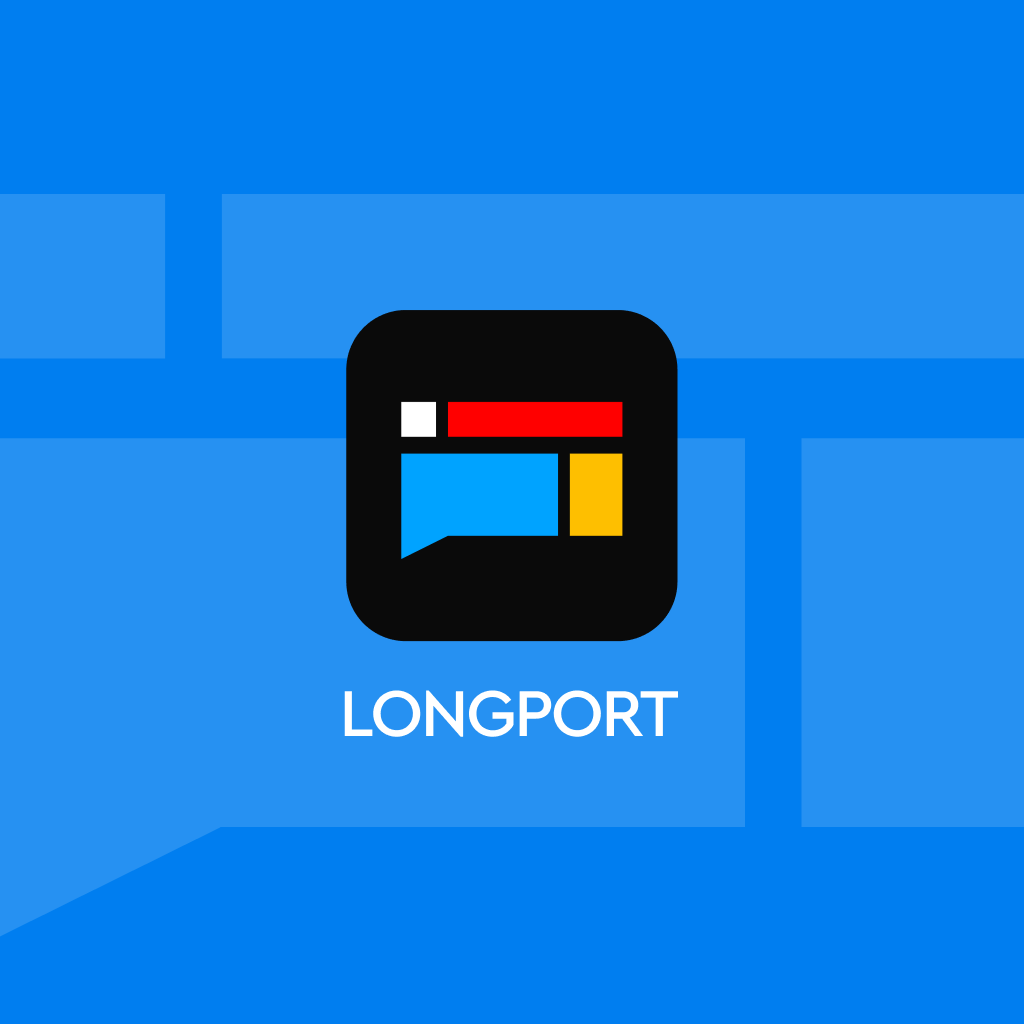
Make history! DeepSeek surpasses ChatGPT to top the China and US App Store
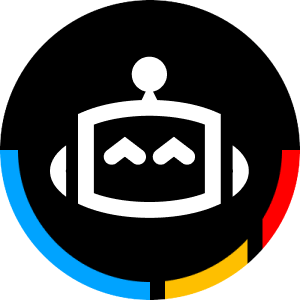
Since its release, DeepSeek has maintained its popularity, surpassing ChatGPT to top the App Store in both China and the United States. This application has become the preferred model for researchers at top universities in the U.S. and is even regarded as a black swan that OpenAI and NVIDIA did not foresee. A wave of interest in reproducing DeepSeek-R1 is emerging, and although its training data and scripts are not fully open-sourced, the technical report provides guidelines for reproduction. The AI community is actively discussing this, and interviews with the founder of DeepSeek have also attracted attention
Since its release on the 20th, DeepSeek has shown no signs of waning popularity. Upon waking up, the iOS app released by DeepSeek even surpassed the official ChatGPT app, directly topping the App Store.
Many netizens believe this is well-deserved.
After all, as Anjney Midha, a partner at a16z and a board member of Mistral, said: from Stanford to MIT, DeepSeek-R1 has almost overnight become the model of choice for researchers at top universities in the United States.
Some netizens even believe that DeepSeek is a black swan that OpenAI and NVIDIA did not foresee.
Meanwhile, various news surrounding DeepSeek-R1 is emerging — organizations like Hugging Face are attempting to replicate R1, interviews previously conducted with DeepSeek have been translated into English and are sparking discussions in the AI community, and Meta, which developed the Llama series of models, seems to be in a state of anxiety... Let's briefly review some of the hot topics surrounding DeepSeek in the past two days.
The interview previously conducted with DeepSeek founder Liang Wenfeng has been translated into English and is sparking discussions in the AI community.
AI Community Kicks Off R1 Replication Craze
DeepSeek-R1 is open-source, but not completely open-source — the relevant training data, training scripts, etc., have not been disclosed. However, due to the technical report, there are guidelines for replicating R1, and recently, many people have emphasized the importance and feasibility of replicating R1.
X blogger @Charbax summarized the areas not covered in the DeepSeek documentation and some challenges in reproducing R1.
Details of the training process. Although the technical report discusses the reinforcement learning phase and distillation, it omits key implementation details, including hyperparameters (e.g., learning rate, batch size, reward scaling factor), the data pipeline used to generate synthetic training data (e.g., how to orchestrate 800K distilled samples), and the reward model architecture for tasks requiring human preference alignment (e.g., "language consistency reward" for multilingual output).
Cold start data generation. While the report mentions the process of creating "high-quality cold start data" (e.g., human standards, few-shot prompts), it lacks specific examples or datasets.
Hardware and infrastructure. There is no detailed information about computing resources (e.g., GPU clusters, training time) or software stack optimizations (e.g., DeepSeek-V3's AMD ROCM integration).
Reproduction challenges. There is a lack of components such as scripts for multi-stage reinforcement learning.
Of course, some teams have already started taking action.
Open R1: Reproducing a true open-source version of R1
Among the various projects aimed at reproducing R1, the most notable is Hugging Face's Open R1 project.
Open R1 claims to be a "fully open reproduction" of DeepSeek-R1, which can fill in the technical details that DeepSeek has not made public. The project is currently ongoing, and completed parts include:
GRPO implementation
Training and evaluation code
Generator for synthetic data
Tweet from Hugging Face CEO Clem Delangue
According to its project introduction, the Open R1 project plans to be implemented in three steps:
Step 1: Reproduce the R1-Distill model, specifically by distilling a high-quality corpus from DeepSeek-R1.
Step 2: Reproduce the pure reinforcement learning pipeline used by DeepSeek to create R1-Zero. This step involves orchestrating a new large-scale dataset that includes mathematics, reasoning, and code dataStep 3: Obtain a reinforcement learning fine-tuned model from the base model through multi-stage training.
7B Model 8K Sample Reproducing R1-Zero and R1
Another team reproducing R1 is led by Junxian He from the Hong Kong University of Science and Technology, and they used a very small base model and sample size: based on the 7B model, only using 8K sample examples, but the results obtained were "surprisingly strong".
It is important to note that most of the experiments conducted by this team were completed before the release of R1. They found that the 7B model could exhibit long chains of thought (CoT) and self-reflection abilities using only 8K MATH examples, and its performance in complex mathematical reasoning was also quite good.
Specifically, they started with the base model Qwen2.5-Math-7B and directly performed reinforcement learning using only 8K samples from the MATH dataset. Ultimately, they obtained Qwen2.5-SimpleRL-Zero and Qwen2.5-SimpleRL.
Or as stated in their blog: "No reward model, no SFT, only 8K Math samples for validation, the resulting model achieved a pass@1 accuracy of 33.3% on AIME, 62.5% on AMC, and 77.2% on MATH, outperforming Qwen2.5-math-7B-instruct, comparable to PRIME and rStar-MATH which used 50 times more data and more complex components."
Training dynamics of Qwen2.5-SimpleRL-Zero
The pass@1 accuracy of the resulting model compared to the baseline model
Reproducing R1 with a 3B model for $30
TinyZero is an attempt to reproduce DeepSeek-R1-Zero, and according to its author, Jiayi Pan, a PhD student at Berkeley AI Research, the project is based on the CountDown game, and the complete recipe can be summarized in one sentence: "Follow the algorithm of DeepSeek R1-Zero — a base language model, prompts, and ground-truth rewards, then run reinforcement learning."
During the experiment, the model's initial output was quite clumsy, but it gradually developed strategies such as modification and search. Below is an example showing how the model proposed solutions, self-validated, and repeatedly modified until successful.
In the experiment, the team also made some interesting discoveries:
The quality of the base model is crucial. The 0.5B small model stops after guessing an answer, while starting from the 1.5B scale, the model begins to learn to search, self-validate, and correct answers, achieving significantly higher scores.
Both the base model and the instruction model are viable. The experiment found that the instruction model learns faster, but its performance converges to a level comparable to that of the base model; at the same time, the outputs of the instruction model are more structured and readable.
The specific reinforcement learning algorithm used is not important. The team tried PPO, GRPO, and PRIME, but their differences were minimal.
The model's reasoning behavior heavily depends on the specific task. For the CountDown game, the model learns to perform searches and self-validation; for numerical multiplication, the model learns to use the distributive property to break down the problem and solve it step by step.
The model learns the distributive property of multiplication
What is most astonishing is that the entire project's computational cost was less than $30.
Meta's Anxiety: The Next Generation Llama May Not Keep Up with R1
A few days ago, an article by Machine Heart titled "Is Meta in Panic? Internal Leak: Crazy Analysis of Copying DeepSeek, High Budget Difficult to Explain" attracted widespread attention and discussion.
In the article, a Meta employee posted anonymously on the American workplace community teamblind, mentioning that the recent series of actions by the domestic AI startup DeepSeek has put Meta's generative AI team in a state of panic.
Today, The Information's latest article revealed more details.
In the article, The Information disclosed that leaders, including Mathew Oldham, Director of AI Infrastructure at Meta, expressed concerns that the next version of Meta Llama may not perform as well as DeepSeek'sMeta also hinted that the next version of Llama will be released this quarter.
In addition, the article revealed that Meta's generative AI team and infrastructure team organized four war rooms to study how DeepSeek works.
Two of the war rooms are trying to understand how the magic square reduces the cost of training and running DeepSeek models. One employee stated that Meta hopes to apply these technologies to Llama.
Some developers revealed that although Meta's models are free, their operating costs are generally higher than those of OpenAI's models, partly because OpenAI can lower prices by processing millions of queries from its model clients in bulk. However, small developers using Llama do not have enough queries to reduce costs.
According to an employee with direct knowledge of the situation, the third war room is trying to figure out what data the magic square might use to train its models.
The fourth war room is considering new technologies based on the DeepSeek model to restructure Meta's models. Meta is considering launching a Llama version similar to DeepSeek, which will include multiple AI models, each handling different tasks. This way, when a customer requests Llama to perform a task, only certain parts of the model need to be processed. This approach can make the entire model run faster and operate with less computing power.
It remains to be seen what kind of open-source model Meta will produce under such pressure in 2025. Perhaps Meta will also join the wave of reproducing R1.
However, it can be anticipated that with the stir caused by DeepSeek, the landscape of large models is undergoing a transformation in the new year.
What are your expectations for the development and application of AI technology in the new year? Feel free to leave a comment for discussion.
Source: Machine Heart, original title: "Creating History! DeepSeek Surpasses ChatGPT to Top the China and U.S. App Store"
Risk Warning and Disclaimer
The market has risks, and investment requires caution. This article does not constitute personal investment advice and does not take into account the specific investment goals, financial situation, or needs of individual users. Users should consider whether any opinions, views, or conclusions in this article align with their specific circumstances. Investment based on this is at your own risk